MTPL-G2T: Graph-to-Text Generation Task Based on Mixed Template Prompt Learning
2022 IEEE/WIC/ACM International Joint Conference on Web Intelligence and Intelligent Agent Technology (WI-IAT)(2022)
摘要
The Graph-to-Text(G2T) generation tasks are mainly done by pre-training and fine-tuning currently, but the drawback of fine-tuning is that it changes all parameters of the pre-trained model. In this paper, we aim to accomplish the text generation task through prompt learning so that no or a small number of model parameters can be changed. Also, we analyze the impact of three different prompt templates on the generation results. The results show that when the pre-trained language model is large (e.g., T5), prompt learning is competitive with finetuning, but the number of parameters that need to be modified for prompt learning is much smaller than for fine-tuning; meanwhile, compared with text templates and soft templates, using mixed prompt templates can make the model converge faster.
更多查看译文
关键词
Prompt learning,Graph-to-Text,T5
AI 理解论文
溯源树
样例
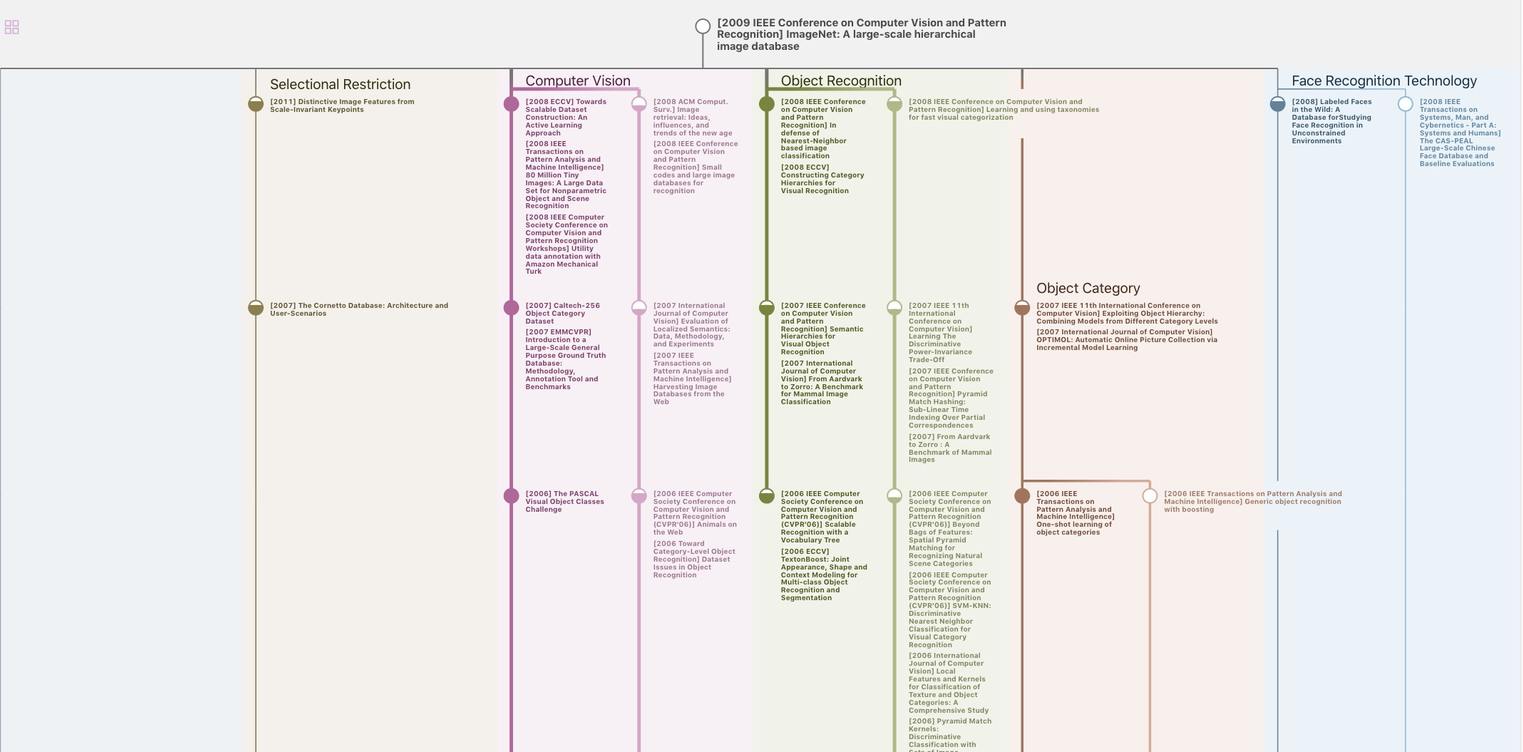
生成溯源树,研究论文发展脉络
Chat Paper
正在生成论文摘要