Matrix-free GPU-accelerated saddle-point solvers for high-order problems in $H(\mathrm{div})$
arXiv (Cornell University)(2023)
摘要
This work describes the development of matrix-free GPU-accelerated solvers for high-order finite element problems in $H(\mathrm{div})$. The solvers are applicable to grad-div and Darcy problems in saddle-point formulation, and have applications in radiation diffusion and porous media flow problems, among others. Using the interpolation-histopolation basis (cf. SIAM J. Sci. Comput., 45 (2023), A675-A702, arXiv:2203.02465), efficient matrix-free preconditioners can be constructed for the $(1,1)$-block and Schur complement of the block system. With these approximations, block-preconditioned MINRES converges in a number of iterations that is independent of the mesh size and polynomial degree. The approximate Schur complement takes the form of an M-matrix graph Laplacian, and therefore can be well-preconditioned by highly scalable algebraic multigrid methods. High-performance GPU-accelerated algorithms for all components of the solution algorithm are developed, discussed, and benchmarked. Numerical results are presented on a number of challenging test cases, including the "crooked pipe" grad-div problem, the SPE10 reservoir modeling benchmark problem, and a nonlinear radiation diffusion test case.
更多查看译文
关键词
matrix-free,gpu-accelerated,saddle-point,high-order
AI 理解论文
溯源树
样例
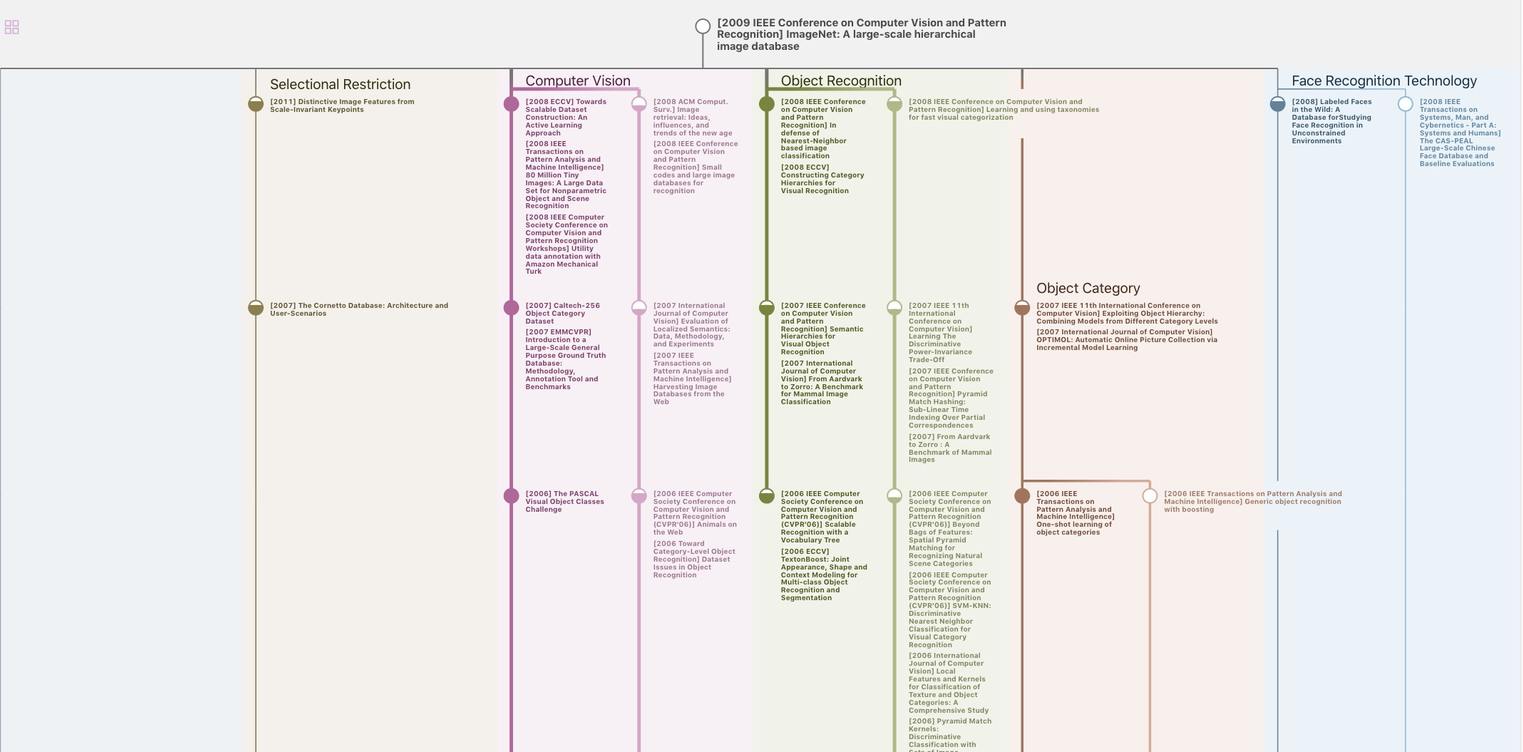
生成溯源树,研究论文发展脉络
Chat Paper
正在生成论文摘要