Contrastive Energy Prediction for Exact Energy-Guided Diffusion Sampling in Offline Reinforcement Learning
ICML 2023(2023)
摘要
Guided sampling is a vital approach for applying diffusion models in real-world tasks that embeds human-defined guidance during the sampling procedure. This paper considers a general setting where the guidance is defined by an (unnormalized) energy function. The main challenge for this setting is that the intermediate guidance during the diffusion sampling procedure, which is jointly defined by the sampling distribution and the energy function, is unknown and is hard to estimate. To address this challenge, we propose an exact formulation of the intermediate guidance as well as a novel training objective named contrastive energy prediction (CEP) to learn the exact guidance. Our method is guaranteed to converge to the exact guidance under unlimited model capacity and data samples, while previous methods can not. We demonstrate the effectiveness of our method by applying it to offline reinforcement learning (RL). Extensive experiments on D4RL benchmarks demonstrate that our method outperforms existing state-of-the-art algorithms. We also provide some examples of applying CEP for image synthesis to demonstrate the scalability of CEP on high-dimensional data.
更多查看译文
关键词
offline reinforcement learning,contrastive energy prediction,diffusion sampling,energy-guided
AI 理解论文
溯源树
样例
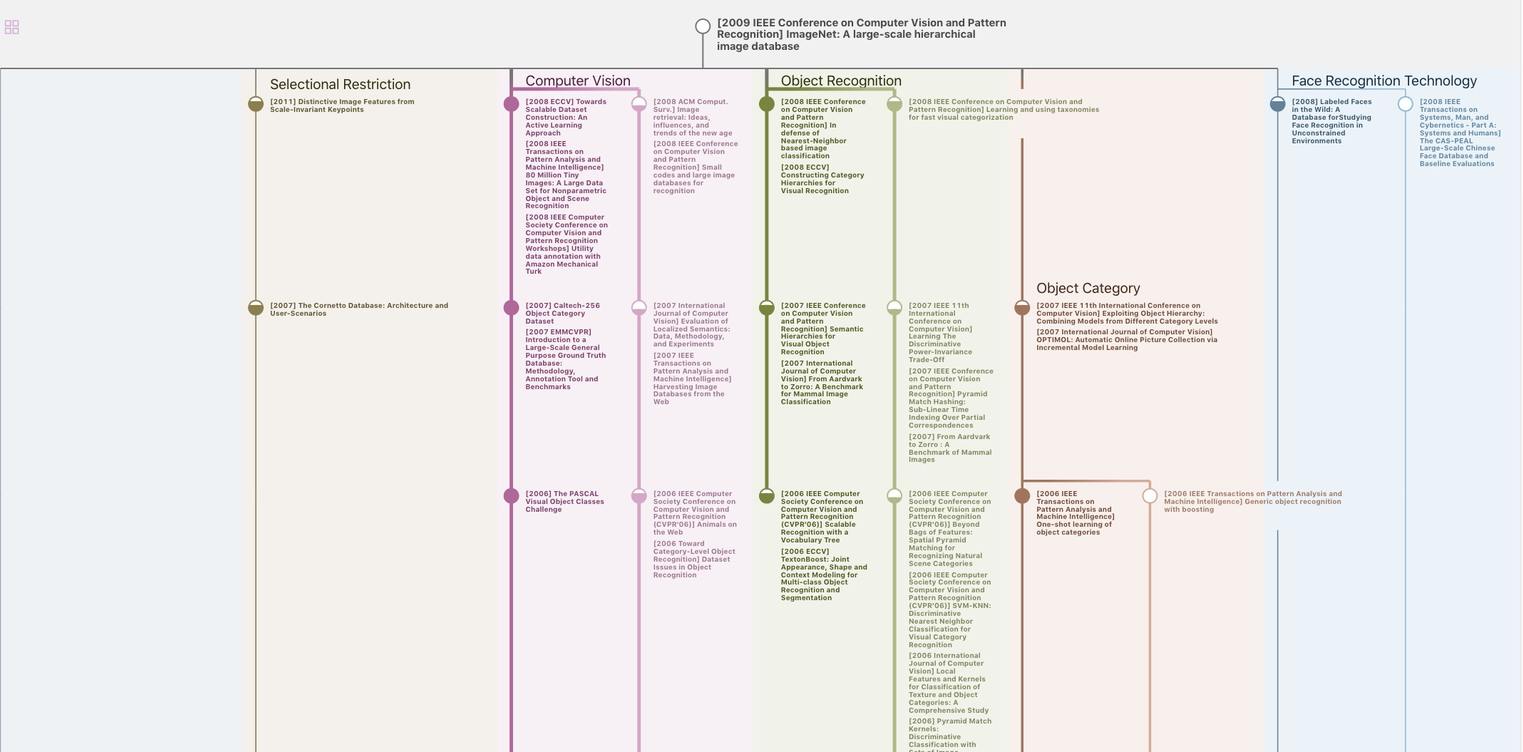
生成溯源树,研究论文发展脉络
Chat Paper
正在生成论文摘要