Hourly forecasting of traffic flow rates using spatial temporal graph neural networks.
Procedia computer science(2023)
摘要
Traffic congestion forms a large problem in many major metropolitan regions around the world, leading to delays and societal costs. As people resume travel upon relaxation of COVID-19 restrictions and personal mobility returns to levels prior to the pandemic, policy makers need tools to understand new patterns in the daily transportation system. In this paper we use a Spatial Temporal Graph Neural Network (STGNN) to train data collected by 34 traffic sensors around Amsterdam, in order to forecast traffic flow rates on an hourly aggregation level for a quarter. Our results show that STGNN did not outperform a baseline seasonal naive model overall, however for sensors that are located closer to each other in the road network, the STGNN model did indeed perform better.
更多查看译文
关键词
Highway Traffic,Metropolitan Regional Congestion,Seasonal Naive,Spatial Temporal Graph Neural Network,Traffic Flow Forecasting
AI 理解论文
溯源树
样例
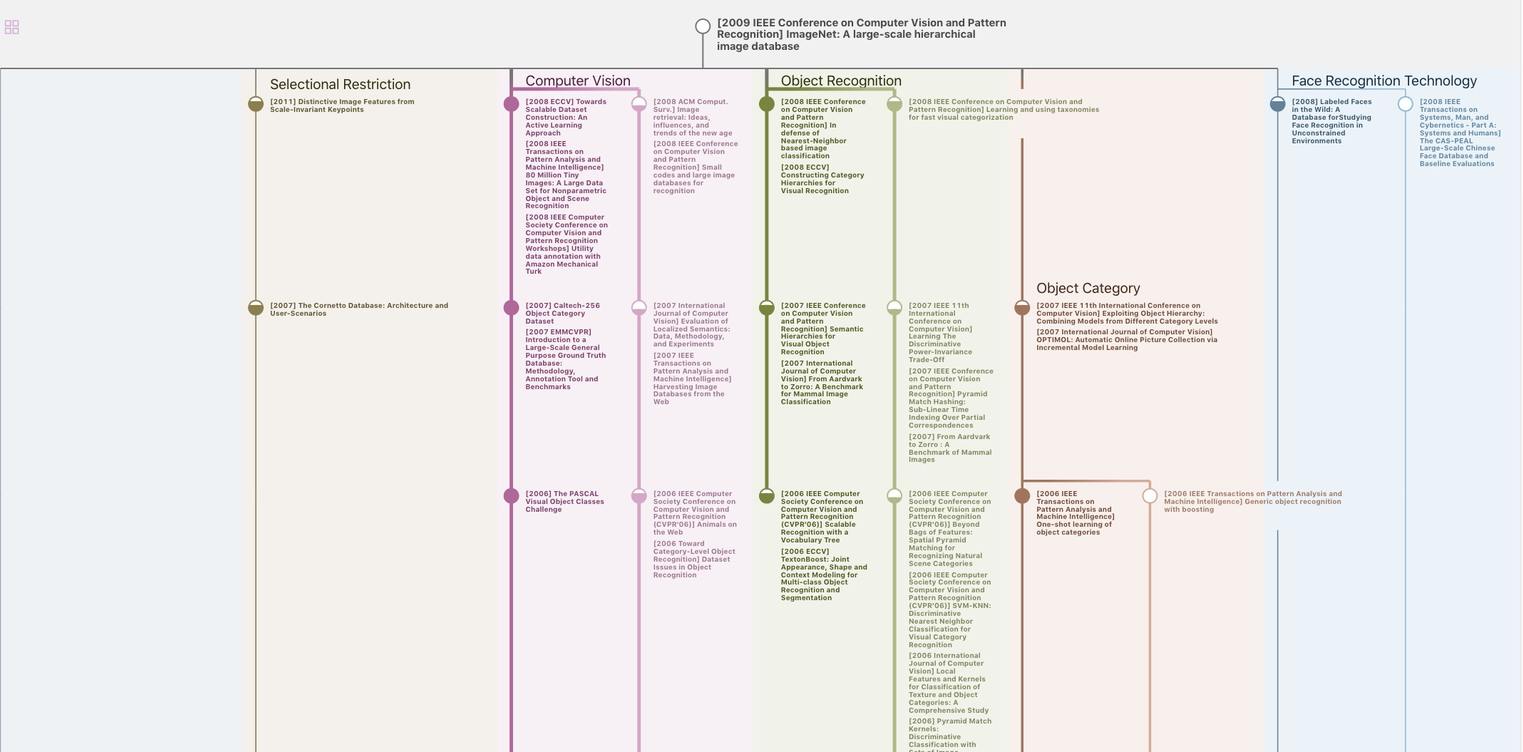
生成溯源树,研究论文发展脉络
Chat Paper
正在生成论文摘要