High-Order Correlation-Guided Slide-Level Histology Retrieval With Self-Supervised Hashing.
IEEE transactions on pattern analysis and machine intelligence(2023)
摘要
Histopathological Whole Slide Images (WSIs) play a crucial role in cancer diagnosis. It is of significant importance for pathologists to search for images sharing similar content with the query WSI, especially in the case-based diagnosis. While slide-level retrieval could be more intuitive and practical in clinical applications, most methods are designed for patch-level retrieval. A few recently unsupervised slide-level methods only focus on integrating patch features directly, without perceiving slide-level information, and thus severely limits the performance of WSI retrieval. To tackle the issue, we propose a High-Order Correlation-Guided Self-Supervised Hashing-Encoding Retrieval (HSHR) method. Specifically, we train an attention-based hash encoder with slide-level representation in a self-supervised manner, enabling it to generate more representative slide-level hash codes of cluster centers and assign weights for each. These optimized and weighted codes are leveraged to establish a similarity-based hypergraph, in which a hypergraph-guided retrieval module is adopted to explore high-order correlations in the multi-pairwise manifold to conduct WSI retrieval. Extensive experiments on multiple TCGA datasets with over 24,000 WSIs spanning 30 cancer subtypes demonstrate that HSHR achieves state-of-the-art performance compared with other unsupervised histology WSI retrieval methods.
更多查看译文
关键词
retrieval,hashing,high-order,correlation-guided,slide-level,self-supervised
AI 理解论文
溯源树
样例
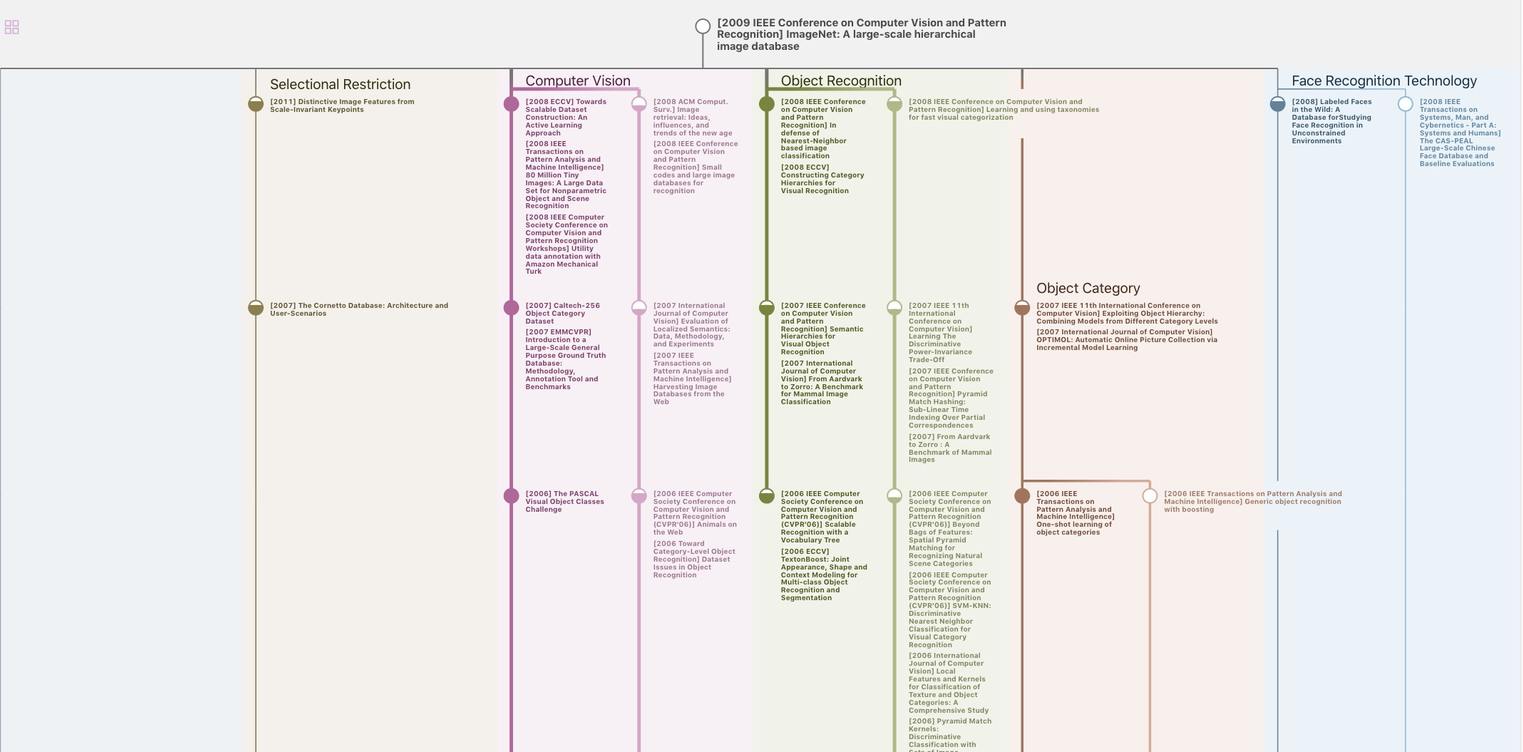
生成溯源树,研究论文发展脉络
Chat Paper
正在生成论文摘要