Transfer learning on structural brain age models to decode cognition in MS: a federated learning approach
MULTIPLE SCLEROSIS JOURNAL(2023)
摘要
Introduction: Classical deep learning research requires lots of centralised data. However, data sets are often stored at different clinical centers, and sharing sensitive patient data such as brain images is difficult. In this manuscript, we investigated the feasibility of federated learning, sending models to the data instead of the other way round, for research on brain magnetic resonant images of people with multiple sclerosis (MS). Methods: Using transfer learning on a previously published brain age model, we trained a model to decode performance on the symbol digit modalities test (SDMT) of patients with multiple sclerosis from structural T1 weighted MRI. Three international centers in Brussels, Greifswald and Prague participated in the project. In Brussels, one computer served as the server coordinating the FL project, while the other served as client for model training on local data (n=97). The other two clients were Greifswald (n=104) and Prague (n=100). Each FL round, the server sent a global model to the clients, where its fully connected layer was updated on the local data. After collecting the local models, the server applied a weighted average of two randomly picked clients, yielding a new global model. Results: After 22 federated learning rounds, the average validation loss across clients reached a minimum. The model appeared to have learned to assign SDMT values close to the mean with a mean absolute error of 9.04, 10.59 and 10.71 points between true and predicted SDMT on the test data sets of Brussels, Greifswald and Prague respectively. The overall test MAE across all clients was 10.13 points. Conclusion: Federated learning is feasible for machine learning research on brain MRI of persons with MS, setting the stage for larger transfer learning studies to investigate the utility of brain age latent representations in cogni- tive decoding tasks.
### Competing Interest Statement
Stijn Denissen prepares a PhD with icometrix as industrial partner. Diana Sima is an employee (senior researcher) of icometrix. Guy Nagels is minority shareholder of icometrix and on a 10% secondment from the UZ Brussel to icometrix as medical director.
### Funding Statement
This study was funded by a personal industrial PhD grant (Baekeland, HBC.2019.2579) appointed by Flanders Innovation and Entrepreneurship to Stijn Denissen and a personal travel grant (V412023N) appointed by the "Fonds Wetenschappelijk Onderzoek" (FWO) to Stijn Denissen for his stay in Prague in the context of this study. This project was furthermore funded by an IOF-POC grant from the Vrije Universiteit Brussel (VUB), by an ITEA grant (20030 HeKDisco, HBC.2021.0500) from Flanders Innovation and Entrepreneurship, by an institutional support from the Czech Ministry of Health (RVO-VFN 64165) and by the National Institute for Neurological Research, Czech Republic, Programme EXCELES, ID Project No. LX22NPO5107, funded by the European Union - Next Generation EU. Guy Nagels is a senior clinical research fellow of the FWO Flanders (1805620N).
### Author Declarations
I confirm all relevant ethical guidelines have been followed, and any necessary IRB and/or ethics committee approvals have been obtained.
Yes
The details of the IRB/oversight body that provided approval or exemption for the research described are given below:
The "Commissie Medische Ethiek" (CME) of the UZ Brussel judged this retrospective study to be exempt from ethical approval (B.U.N. 1432022000303). For data at each center in this study, ethical approval was obtained prior to data acquisition (Brussels: B.U.N. 143201423263, Greifswald: BB159/18, Prague: 113/22 S-IV and 28/17), and written informed consent was acquired from all subjects prior to inclusion.
I confirm that all necessary patient/participant consent has been obtained and the appropriate institutional forms have been archived, and that any patient/participant/sample identifiers included were not known to anyone (e.g., hospital staff, patients or participants themselves) outside the research group so cannot be used to identify individuals.
Yes
I understand that all clinical trials and any other prospective interventional studies must be registered with an ICMJE-approved registry, such as ClinicalTrials.gov. I confirm that any such study reported in the manuscript has been registered and the trial registration ID is provided (note: if posting a prospective study registered retrospectively, please provide a statement in the trial ID field explaining why the study was not registered in advance).
Yes
I have followed all appropriate research reporting guidelines, such as any relevant EQUATOR Network research reporting checklist(s) and other pertinent material, if applicable.
Yes
Retrospective data used in the present study are confidential clinical data. Interested researchers can contact Guy Nagels for the Brussels data, Matthias Grothe for the Greifswald data and Manuela Vaneckova for the Prague data.
更多查看译文
关键词
structural brain age models,learning,cognition,ms
AI 理解论文
溯源树
样例
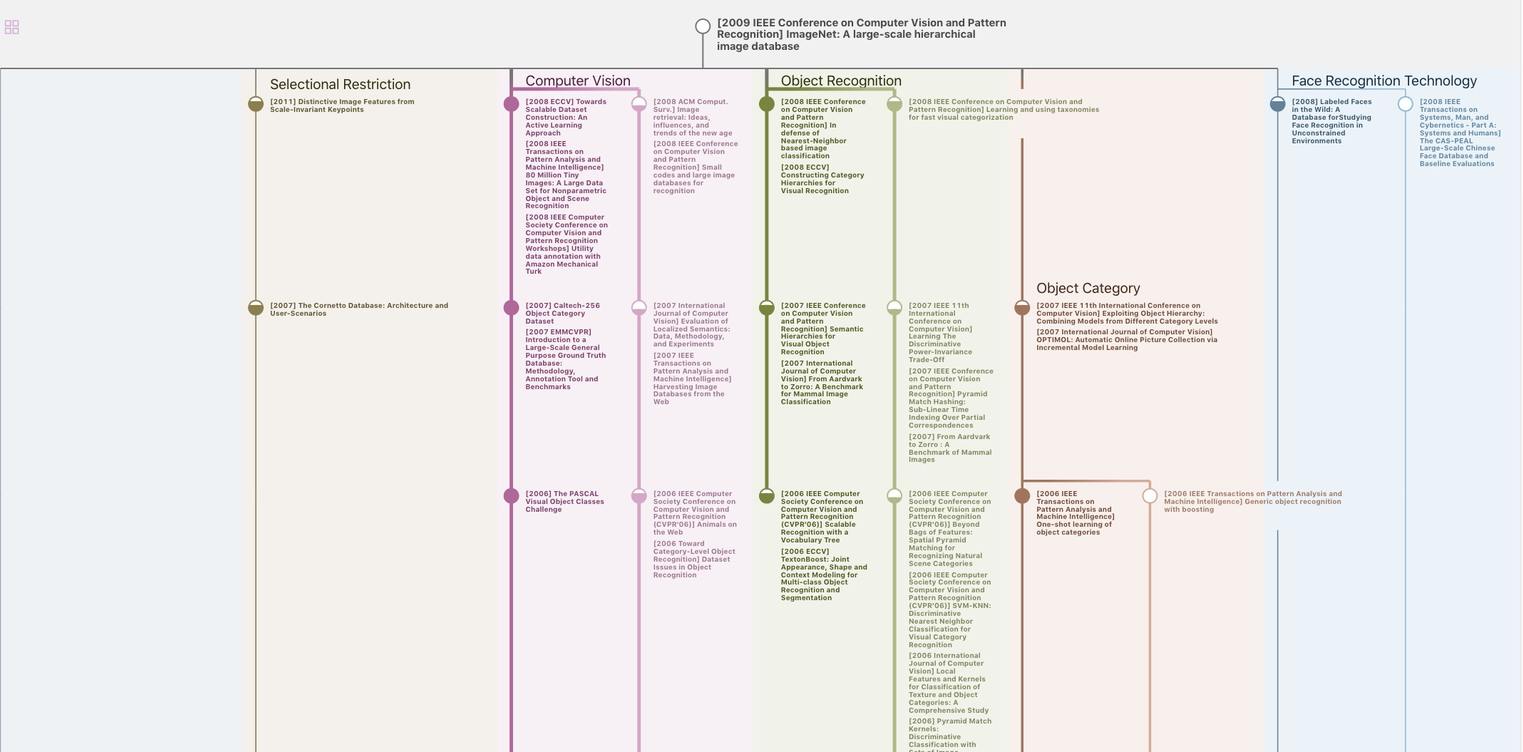
生成溯源树,研究论文发展脉络
Chat Paper
正在生成论文摘要