Automated calibration of consensus weighted distance-based clustering approaches using sharp
BIOINFORMATICS(2023)
摘要
Motivation In consensus clustering, a clustering algorithm is used in combination with a subsampling procedure to detect stable clusters. Previous studies on both simulated and real data suggest that consensus clustering outperforms native algorithms.Results We extend here consensus clustering to allow for attribute weighting in the calculation of pairwise distances using existing regularized approaches. We propose a procedure for the calibration of the number of clusters (and regularization parameter) by maximizing the sharp score, a novel stability score calculated directly from consensus clustering outputs, making it extremely computationally competitive. Our simulation study shows better clustering performances of (i) approaches calibrated by maximizing the sharp score compared to existing calibration scores and (ii) weighted compared to unweighted approaches in the presence of features that do not contribute to cluster definition. Application on real gene expression data measured in lung tissue reveals clear clusters corresponding to different lung cancer subtypes.Availability and implementation The R package sharp (version >= 1.4.3) is available on CRAN at https://CRAN.R-project.org/package=sharp. Graphical Abstract In consensus weighted clustering, the COSA algorithm is applied on multiple subsamples of the data with different regularisation parameters. A co-membership matrix can be obtained by applying a distance-based clustering algorithm (e.g. hierarchical clustering) with a given number of clusters on each of these weighted distance matrices. The consensus matrix is then calculated by aggregating all co-membership matrices over the subsamples for a given regularisation parameter and number of clusters. We propose to calibrate jointly these two hyper-parameters by maximising the sharp score.
更多查看译文
AI 理解论文
溯源树
样例
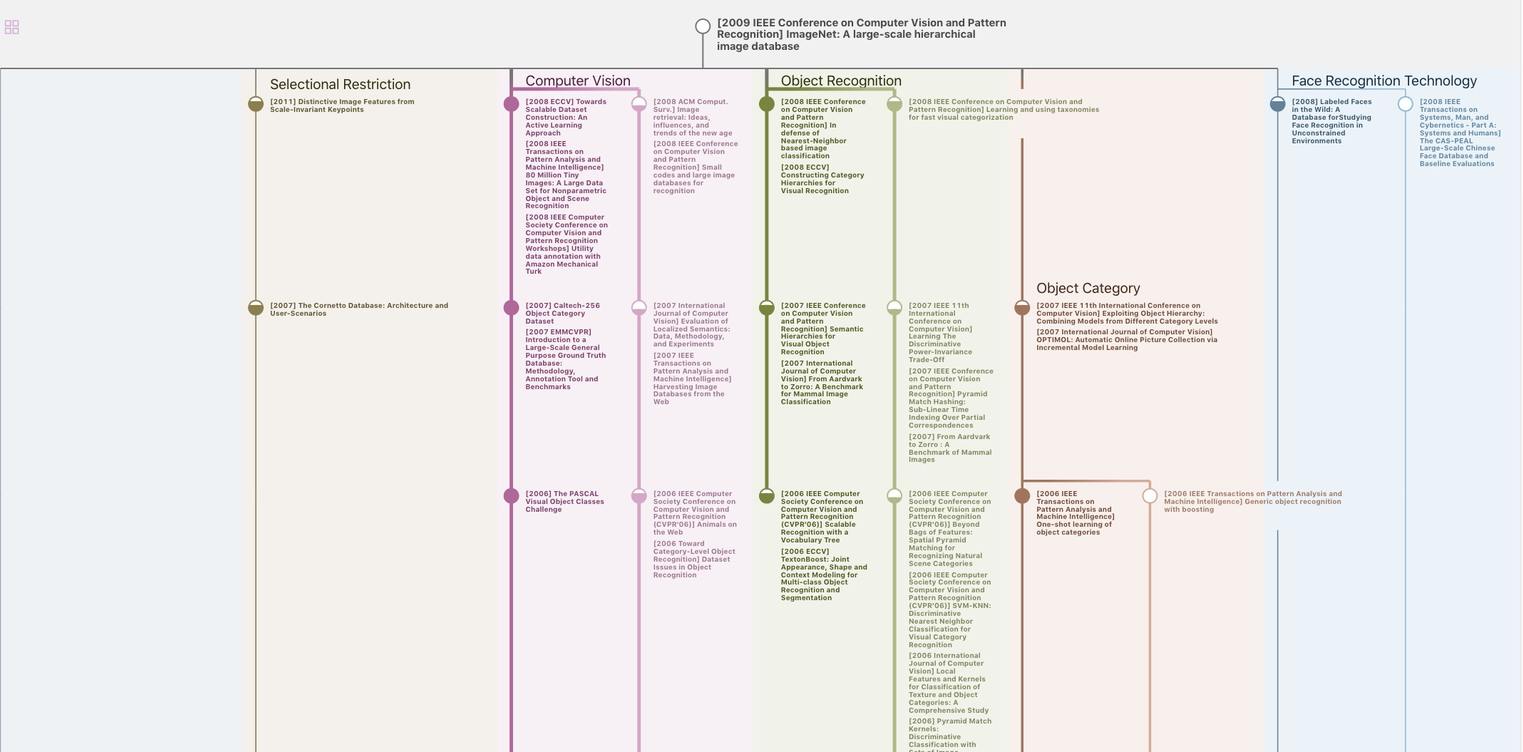
生成溯源树,研究论文发展脉络
Chat Paper
正在生成论文摘要