Comparing Zealous and Restrained AI Recommendations in a Real-World Human-AI Collaboration Task
CHI 2023(2023)
摘要
When designing an AI-assisted decision-making system, there is often a tradeoff between precision and recall in the AI's recommendations. We argue that careful exploitation of this tradeoff can harness the complementary strengths in the human-AI collaboration to significantly improve team performance. We investigate a real-world video anonymization task for which recall is paramount and more costly to improve. We analyze the performance of 78 professional annotators working with a) no AI assistance, b) a high-precision "restrained" AI, and c) a high-recall "zealous" AI in over 3,466 person-hours of annotation work. In comparison, the zealous AI helps human teammates achieve significantly shorter task completion time and higher recall. In a follow-up study, we remove AI assistance for everyone and find negative training effects on annotators trained with the restrained AI. These findings and our analysis point to important implications for the design of AI assistance in recall-demanding scenarios.
更多查看译文
关键词
human-AI team, AI-assisted decision making, precision and recall, real-world application, empirical study, computer vision, face detection, video annotation
AI 理解论文
溯源树
样例
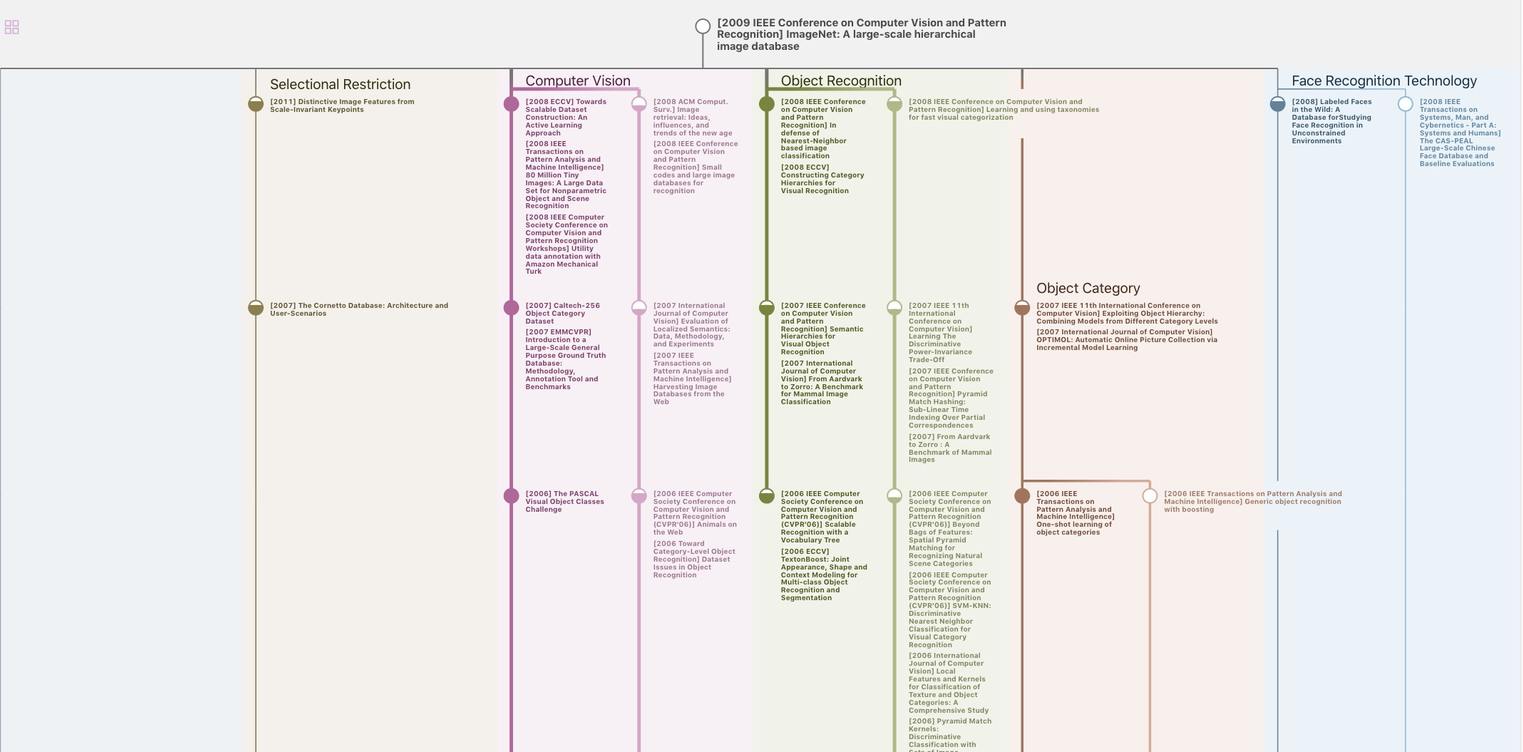
生成溯源树,研究论文发展脉络
Chat Paper
正在生成论文摘要