Optimal Covariance Cleaning for Heavy-Tailed Distributions: Insights from Information Theory
Physical review E(2023)
摘要
In optimal covariance cleaning theory, minimizing the Frobenius norm between the true population covariance matrix and a rotational invariant estimator is a key step. This estimator can be obtained asymptotically for large covariance matrices, without knowledge of the true covariance matrix. In this study, we demonstrate that this minimization problem is equivalent to minimizing the loss of information between the true population covariance and the rotational invariant estimator for normal multivariate variables. However, for Student's t distributions, the minimal Frobenius norm does not necessarily minimize the information loss in finite-sized matrices. Nevertheless, such deviations vanish in the asymptotic regime of large matrices, which might extend the applicability of random matrix theory results to Student's t distributions. These distributions are characterized by heavy tails and are frequently encountered in real-world applications such as finance, turbulence, or nuclear physics. Therefore, our work establishes a connection between statistical random matrix theory and estimation theory in physics, which is predominantly based on information theory.
更多查看译文
关键词
Covariance Matrices
AI 理解论文
溯源树
样例
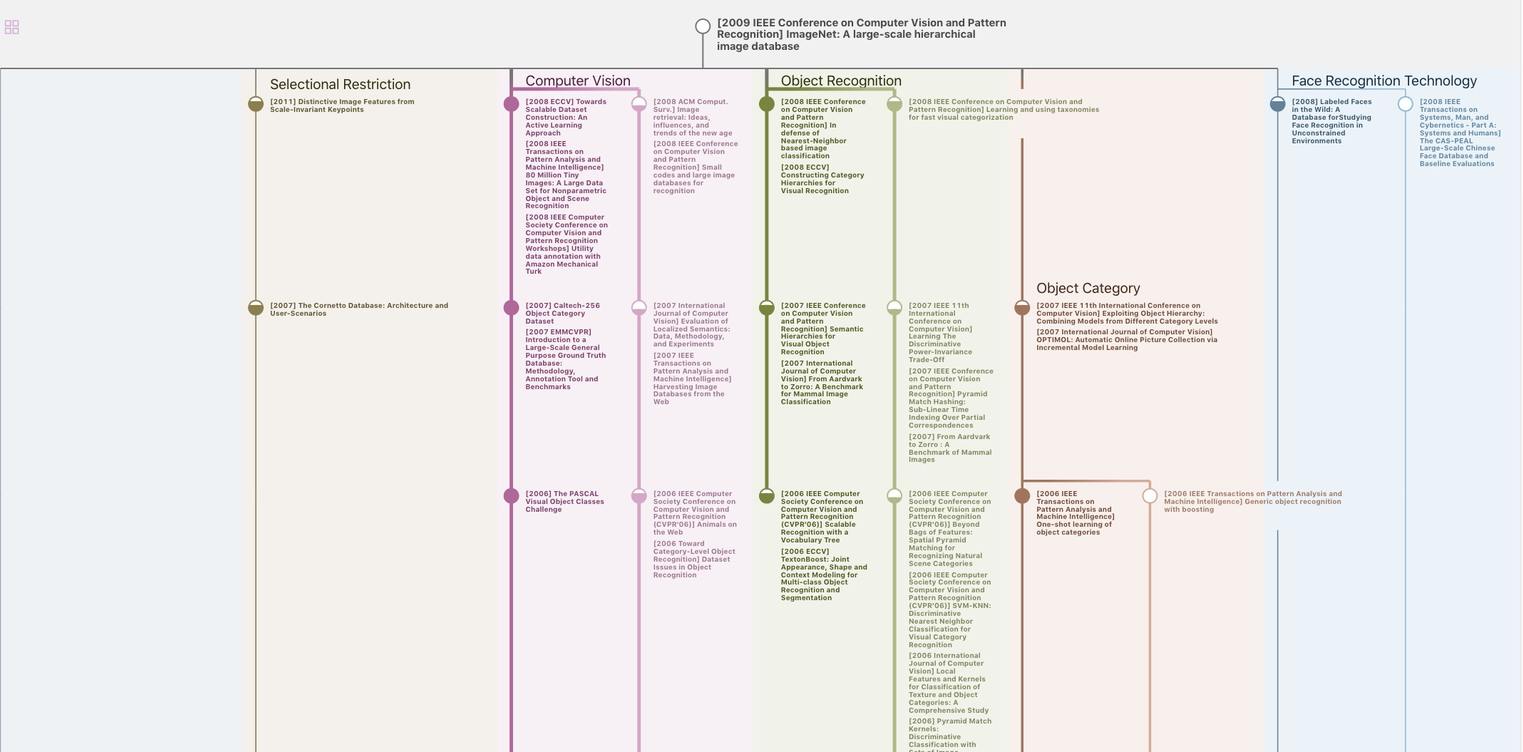
生成溯源树,研究论文发展脉络
Chat Paper
正在生成论文摘要