De-Identification of Chinese-English Code-Mixed Clinical Text Using Pre-Trained Language Models and In Context-Learning of Large Language Models (Preprint)
crossref(2023)
摘要
BACKGROUND The widespread use of electronic health records in clinical and biomedical fields makes the removal of protected health information (PHI) essential to maintain privacy. However, a significant portion of information is recorded in unstructured textual form posing a challenge to de-identify. In countries like Taiwan, medical records could be written in a mixture of more than one language, referred to as code-mixing (CM). Most current clinical natural language processing techniques are designed for monolingual texts, and there is a need to address the de-identification of CM texts. OBJECTIVE The aim of this study was to investigate the effectiveness and underlying mechanism of fine-tuned PLMs in identifying PHIs in CM context. Additionally, we also aimed to evaluate the potential of prompting LLMs in recognizing PHIs in a zero-shot manner. METHODS We compiled the first clinical CM deidentification dataset consisting of texts written in Chinese and English. We explored the effectiveness of fine-tuning pre-trained language models (PLMs) in recognizing PHIs in CM content, focusing on whether PLMs exploit naming regularity and mention coverage to achieve superior performance by probing the developed models’ outputs to examine their decision-making process. Furthermore, we investigated the potential of prompt-based in-context learning of large language models (LLMs) in recognizing PHIs in CM text. RESULTS The developed methods were evaluated on a CM de-identification corpus of 1,700 discharge summaries. We observed that different PHI types had their preference in their occurrence within the different types of language-mixed sentences, and PLMs could effectively recognize PHIs by exploiting the learned name regularity. However, the models may exhibit suboptimal results when regularity was weak or mentions contain unknown words that the representations cannot generate well. We also found that the availability of CM training instances is essential for the model’s performance. Furthermore, LLM-based de-identification method is a feasible and appealing approach that can be controlled and enhanced through natural language prompts. CONCLUSIONS The study contributes to understanding the underlying mechanism of PLMs in addressing the de-identification process in CM context and highlights the significance of incorporating CM training instances into the model training phase. The LLM-based de-identification method is a feasible approach, but carefully crafted prompts are essential to avoid unwanted output. However, the use of such methods in the hospital setting requires careful consideration of data security and privacy concerns. Further research could explore the augmentation of PLMs and LLMs with external knowledge to improve their strength in recognizing rare PHIs.
更多查看译文
AI 理解论文
溯源树
样例
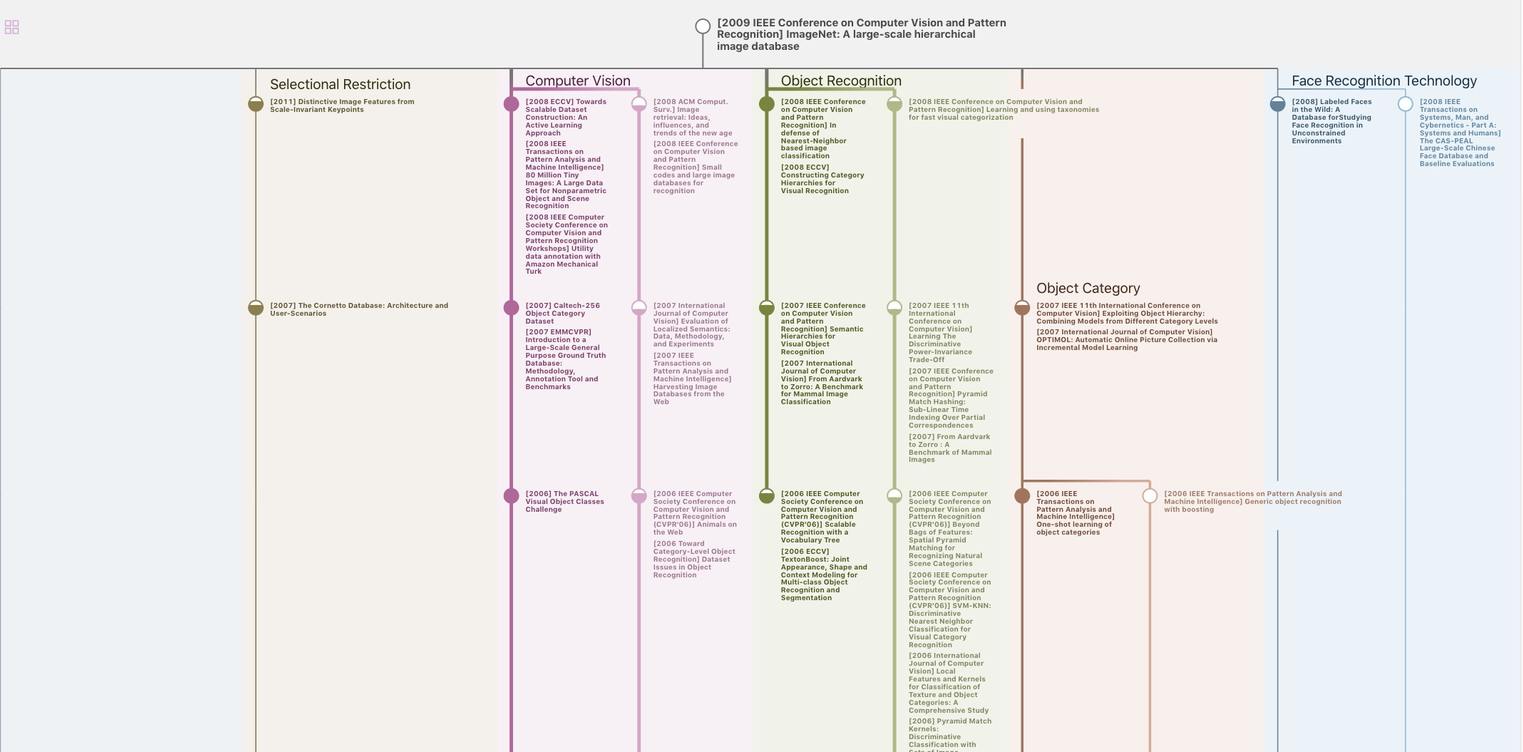
生成溯源树,研究论文发展脉络
Chat Paper
正在生成论文摘要