Priors, population sizes, and power in genome-wide hypothesis tests
BMC bioinformatics(2023)
摘要
Background Genome-wide tests, including genome-wide association studies (GWAS) of germ-line genetic variants, driver tests of cancer somatic mutations, and transcriptome-wide association tests of RNAseq data, carry a high multiple testing burden. This burden can be overcome by enrolling larger cohorts or alleviated by using prior biological knowledge to favor some hypotheses over others. Here we compare these two methods in terms of their abilities to boost the power of hypothesis testing. Results We provide a quantitative estimate for progress in cohort sizes and present a theoretical analysis of the power of oracular hard priors: priors that select a subset of hypotheses for testing, with an oracular guarantee that all true positives are within the tested subset. This theory demonstrates that for GWAS, strong priors that limit testing to 100–1000 genes provide less power than typical annual 20–40% increases in cohort sizes. Furthermore, non-oracular priors that exclude even a small fraction of true positives from the tested set can perform worse than not using a prior at all. Conclusion Our results provide a theoretical explanation for the continued dominance of simple, unbiased univariate hypothesis tests for GWAS: if a statistical question can be answered by larger cohort sizes, it should be answered by larger cohort sizes rather than by more complicated biased methods involving priors. We suggest that priors are better suited for non-statistical aspects of biology, such as pathway structure and causality, that are not yet easily captured by standard hypothesis tests.
更多查看译文
关键词
Genome-wide association studies (GWAS),Genomics,Multiple hypothesis testing,Population genetics,Statistical genetics
AI 理解论文
溯源树
样例
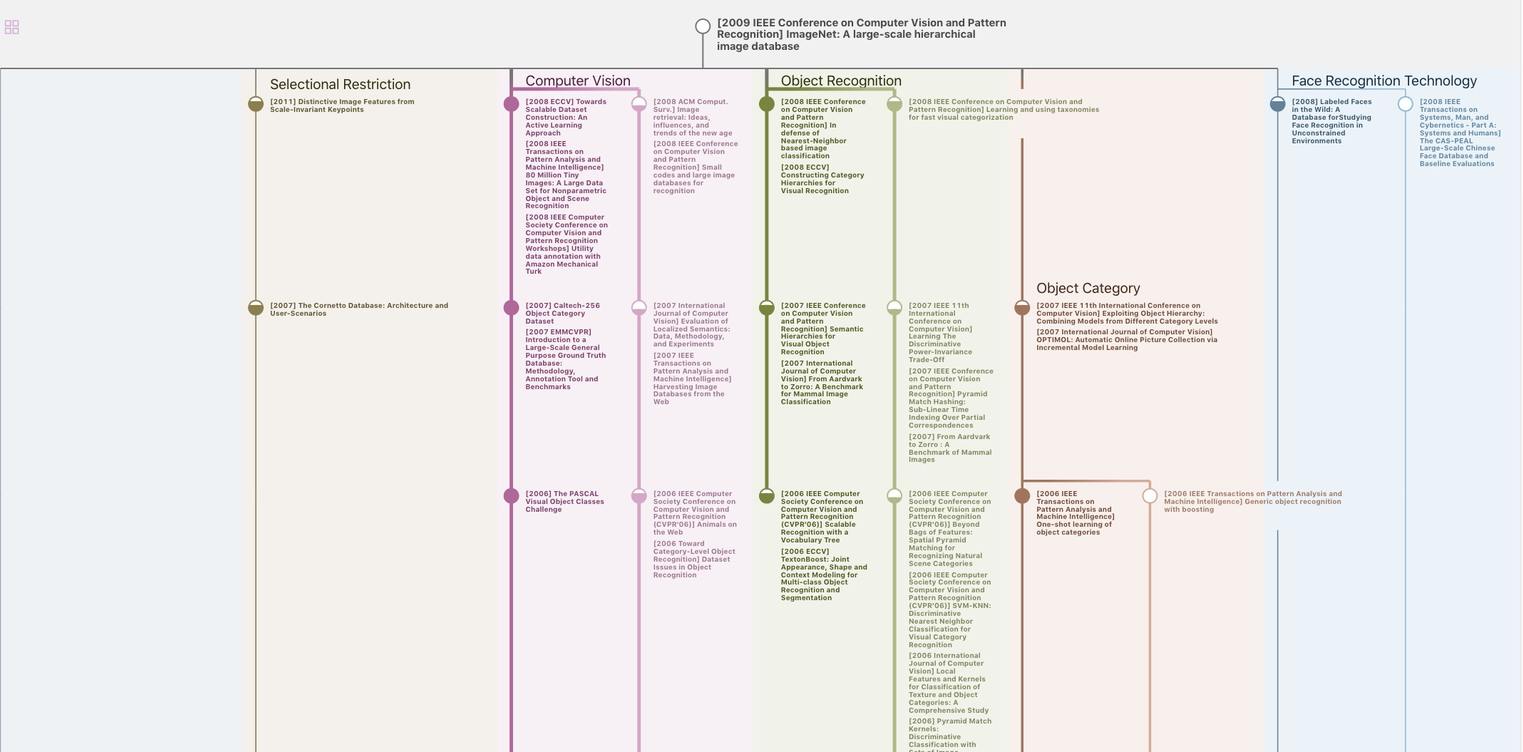
生成溯源树,研究论文发展脉络
Chat Paper
正在生成论文摘要