Biological Signals for Diagnosing Sleep Stages Using Machine Learning Models
2023 28th International Computer Conference, Computer Society of Iran (CSICC)(2023)
摘要
Sleep quality is important for health and can prevent diseases. Polysomnography is a standard scientific and clinical tool to evaluate sleep. Sleep staging is one of the main tasks in the sleep study that characterizes sleep cycles. In recent years, many studies are conducted using machine learning approaches to classify sleep stages. These studies can improve the accuracy and speed of the classification task, but most of them have not considered the performance of minority sleep classes. Since the number of samples in the sleep classes varies greatly, it can be considered as imbalanced data. In this study, we propose an ensemble method to handle class imbalance problem in the sleep staging task. For this purpose, we select nine biomedical signals including two EEG channels, two EOG channels, EMG, ECG, Abdominal, Thorax, and Airflow from the Sleep Heart Health Study (SHHS1) dataset. Then we use an ensemble of data-level resampling methods to rebalance the data space of sleep classes. Finally, we employ different machine learning algorithms to classify sleep stages. To evaluate the performance of the proposed method, in addition to general metrics, we use various measures such as Geometric mean (G-mean) and Matthew's Correlation Coefficient (MCC), which are proper metrics for the imbalanced data classification. The results of the developed method show that it achieves high accuracies of 0.9727, 0.9410, 0.8816, and 0.8725 for two, three, five, and six sleep classes, respectively.
更多查看译文
关键词
Machine Learning,Sleep Staging,Imbalanced Data,Biological Signals,Polysomnography,Sleep Heart Health Study
AI 理解论文
溯源树
样例
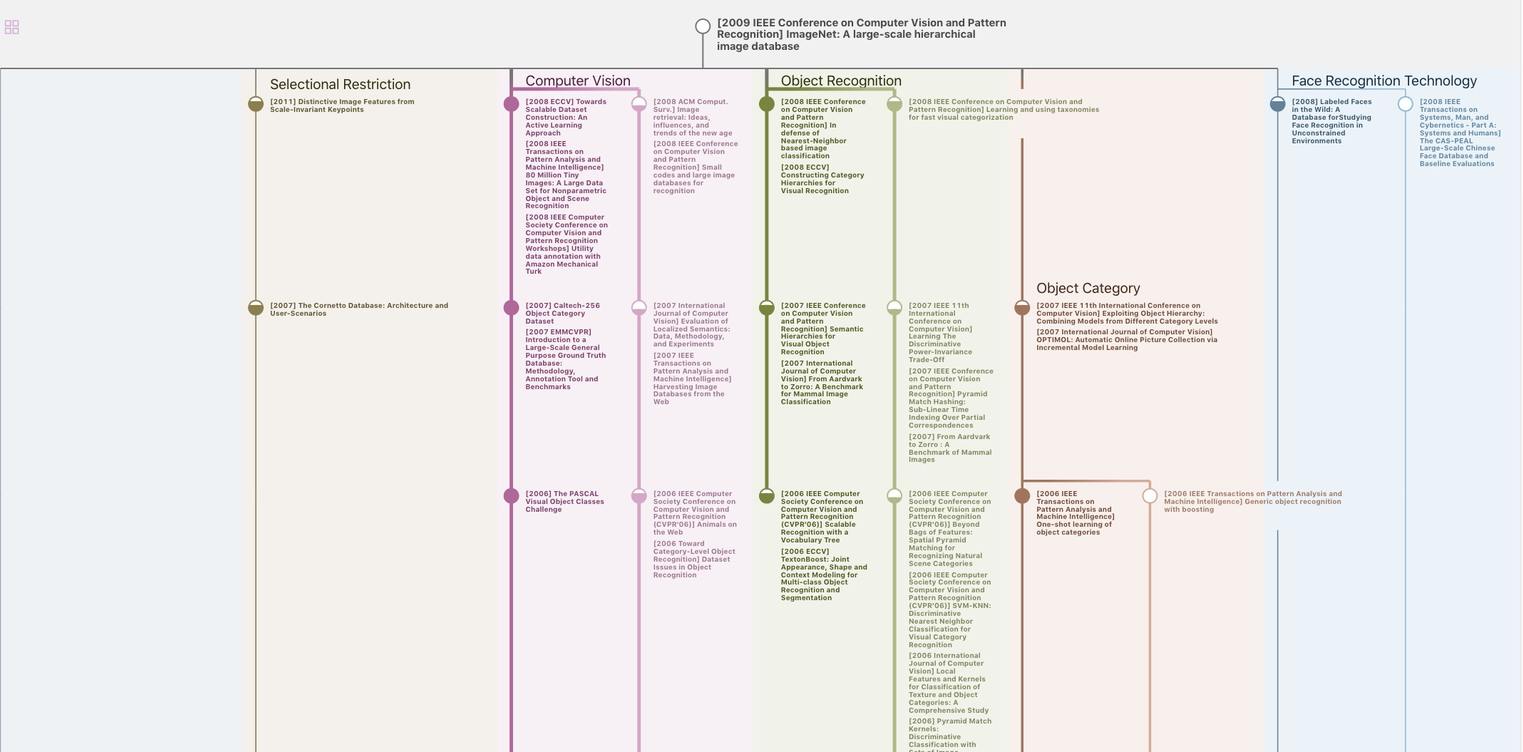
生成溯源树,研究论文发展脉络
Chat Paper
正在生成论文摘要