Estimation of body condition score change in dairy cows in a seasonal calving pasture-based system using routinely available milk mid-infrared spectra and machine learning techniques
Journal of dairy science(2023)
摘要
Body condition score (BCS) is a subjective estimate of body reserves in cows. Body condition score and its change in early lactation have been associated with cow fertility and health. The aim of the present study was to estimate change in BCS (ABCS) using mid-infrared spectra of the milk, with a particular focus on estimating ABCS in cows losing BCS at the fastest rate (i.e., the cows most of interest to the producer). A total of 73,193 BCS records (scale 1 to 5) from 6,572 cows were recorded. Daily BCS was interpolated from cubic splines fitted through the BCS records, and subsequently used to calculate daily ABCS. Body condition score change records were merged with milk mid-infrared spectra recorded on the same week. Both morning (a.m.) and evening (p.m.) spectra were avail-able. Two different statistical methods were used to estimate ABCS: partial least squares regression and a neural network (NN). Several combinations of variables were included as model features, such as days in milk (DIM) only, a.m. spectra only and DIM, p.m. spectra only and DIM, and a.m. and p.m. spectra as well as DIM. The data used to estimate ABCS were either based on the first 120 DIM or all 305 DIM. Daily ABCS had a standard deviation of 1.65 x 10-3 BCS units in the 305 DIM data set and of 1.98 x 10-3 BCS units in the 120 DIM data set. Each data set was divided into 4 sub-data sets, 3 of which were used for training the prediction model and the fourth to test it. This process was repeated until all the sub-data sets were considered as the test data set once. Using all 305 DIM, the lowest root mean square error of validation (RMSEV; 0.96 x 10-3 BCS units) and the strongest correlation between actual and estimated ABCS (0.82) was achieved with NN using a.m. and p.m. spectra and DIM. Using the 120 DIM data, the lowest RMSEV (0.98 x 10-3 BCS units) and the strongest correlation between actual and estimated ABCS (0.87) was achieved with NN using DIM and either a.m. spectra only or a.m. and p.m. spectra together. The RMSEV for records in the lowest 2.5% ABCS percentile per DIM in early lactation was reduced up to a maximum of 13% when spectra and DIM were both considered in the model compared with a model that considered just DIM. The performance of the NN using DIM and a.m. spectra only with the 120 DIM data was robust across different strata of farm, parity, year of sampling, and breed. Results from the present study demonstrate the ability of mid-infrared spectra of milk coupled with machine learning tech-niques to estimate ABCS; specifically, the inclusion of spectral data reduced the RMSEV over and above using DIM alone, particularly for cows losing BCS at the fastest rate. This approach can be used to routinely generate estimates of ABCS that can subsequently be used for farm decisions.
更多查看译文
关键词
machine learning,days in milk,energy status,mid-infrared spectroscopy
AI 理解论文
溯源树
样例
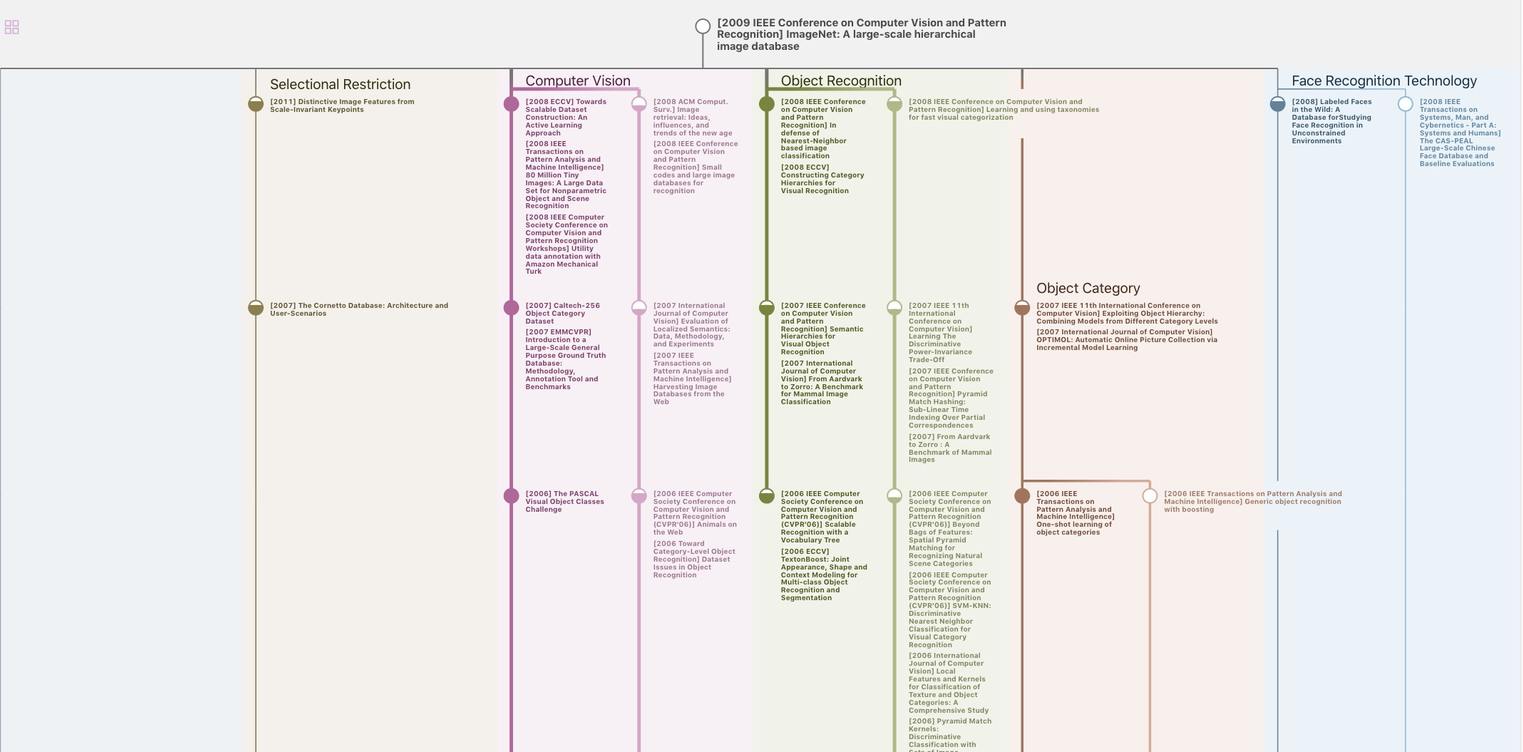
生成溯源树,研究论文发展脉络
Chat Paper
正在生成论文摘要