Anomaly Detection for Sensor Signals Utilizing Deep Learning Autoencoder-Based Neural Networks
Bioengineering(2023)
摘要
Anomaly detection is a significant task in sensors’ signal processing since interpreting an abnormal signal can lead to making a high-risk decision in terms of sensors’ applications. Deep learning algorithms are effective tools for anomaly detection due to their capability to address imbalanced datasets. In this study, we took a semi-supervised learning approach, utilizing normal data for training the deep learning neural networks, in order to address the diverse and unknown features of anomalies. We developed autoencoder-based prediction models to automatically detect anomalous data recorded by three electrochemical aptasensors, with variations in the signals’ lengths for particular concentrations, analytes, and bioreceptors. Prediction models employed autoencoder networks and the kernel density estimation (KDE) method for finding the threshold to detect anomalies. Moreover, the autoencoder networks were vanilla, unidirectional long short-term memory (ULSTM), and bidirectional LSTM (BLSTM) autoencoders for the training stage of the prediction models. However, the decision-making was based on the result of these three networks and the integration of vanilla and LSTM networks’ results. The accuracy as a performance metric of anomaly prediction models showed that the performance of vanilla and integrated models were comparable, while the LSTM-based autoencoder models showed the least accuracy. Considering the integrated model of ULSTM and vanilla autoencoder, the accuracy for the dataset with the lengthier signals was approximately 80%, while it was 65% and 40% for the other datasets. The lowest accuracy belonged to the dataset with the least normal data in its dataset. These results demonstrate that the proposed vanilla and integrated models can automatically detect abnormal data when there is sufficient normal data for training the models.
更多查看译文
关键词
time series anomaly detection,outlier detection,semi-supervised learning,signal processing,autoencoder (AE),vanilla autoencoder,long short-term memory (LSTM),LSTM-based autoencoder,kernel density estimation (KDE)
AI 理解论文
溯源树
样例
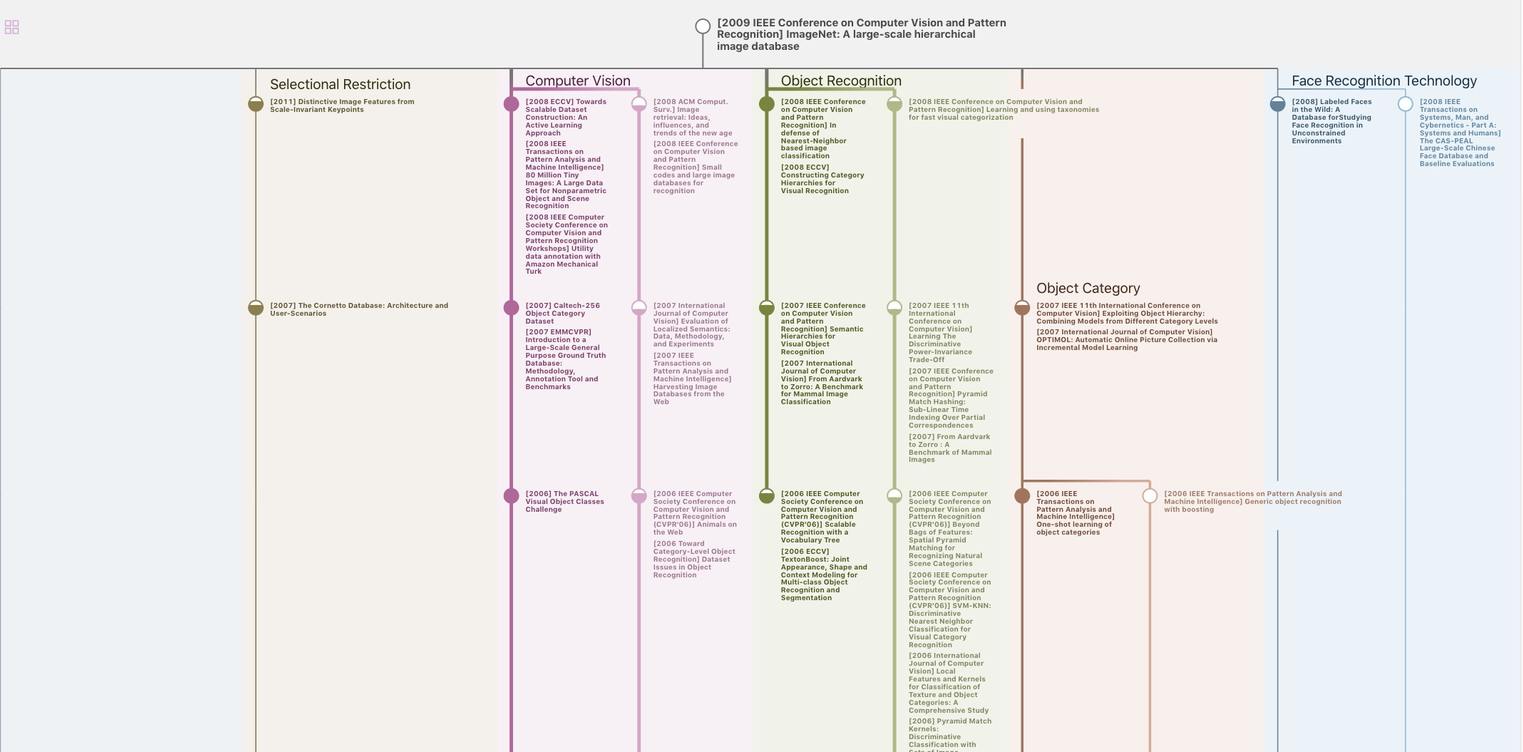
生成溯源树,研究论文发展脉络
Chat Paper
正在生成论文摘要