Using Machine Learning for the Risk Factors Classification of Glycemic Control in Type 2 Diabetes Mellitus.
Healthcare (Basel, Switzerland)(2023)
摘要
Several risk factors are related to glycemic control in patients with type 2 diabetes mellitus (T2DM), including demographics, medical conditions, negative emotions, lipid profiles, and heart rate variability (HRV; to present cardiac autonomic activity). The interactions between these risk factors remain unclear. This study aimed to use machine learning methods of artificial intelligence to explore the relationships between various risk factors and glycemic control in T2DM patients. The study utilized a database from Lin et al. (2022) that included 647 T2DM patients. Regression tree analysis was conducted to identify the interactions among risk factors that contribute to glycated hemoglobin (HbA1c) values, and various machine learning methods were compared for their accuracy in classifying T2DM patients. The results of the regression tree analysis revealed that high depression scores may be a risk factor in one subgroup but not in others. When comparing different machine learning classification methods, the random forest algorithm emerged as the best-performing method with a small set of features. Specifically, the random forest algorithm achieved 84% accuracy, 95% area under the curve (AUC), 77% sensitivity, and 91% specificity. Using machine learning methods can provide significant value in accurately classifying patients with T2DM when considering depression as a risk factor.
更多查看译文
关键词
artificial intelligence,depression,glycemic control,machine learning,type 2 diabetes mellitus
AI 理解论文
溯源树
样例
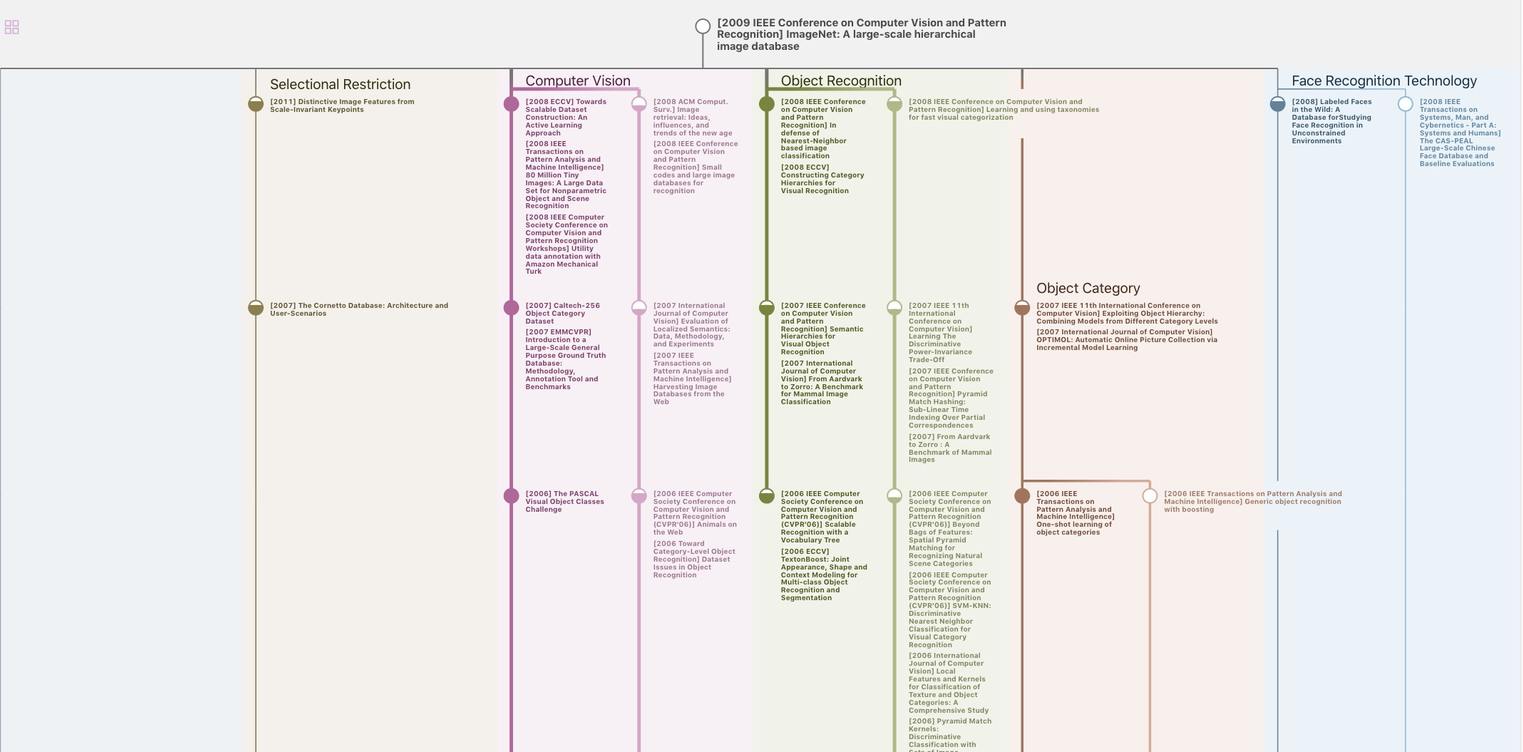
生成溯源树,研究论文发展脉络
Chat Paper
正在生成论文摘要