A Multiscale Attentional Unet Model for Automatic Segmentation in Medical Ultrasound Images.
Ultrasonic imaging(2023)
摘要
Ultrasonography has become an essential part of clinical diagnosis owing to its noninvasive, and real-time nature. To assist diagnosis, automatically segmenting a region of interest (ROI) in ultrasound images is becoming a vital part of computer-aided diagnosis (CAD). However, segmenting ROIs on medical images with relatively low contrast is a challenging task. To better achieve medical ROI segmentation, we propose an efficient module denoted as multiscale attentional convolution (MSAC), utilizing cascaded convolutions and a self-attention approach to concatenate features from various receptive field scales. Then, MSAC-Unet is constructed based on Unet, employing MSAC instead of the standard convolution in each encoder and decoder for segmentation. In this study, two representative types of ultrasound images, one of the thyroid nodules and the other of the brachial plexus nerves, were used to assess the effectiveness of the proposed approach. The best segmentation results from MSAC-Unet were achieved on two thyroid nodule datasets (TND-PUH3 and DDTI) and a brachial plexus nerve dataset (NSD) with Dice coefficients of 0.822, 0.792, and 0.746, respectively. The analysis of segmentation results shows that our MSAC-Unet greatly improves the segmentation accuracy with more reliable ROI edges and boundaries, decreasing the number of erroneously segmented ROIs in ultrasound images.
更多查看译文
关键词
ultrasound images,automatic ROI segmentation model,thyroid nodule segmentation,brachial plexus nerve segmentation,multiscale attentional convolution (MSAC),computer-aided diagnosis
AI 理解论文
溯源树
样例
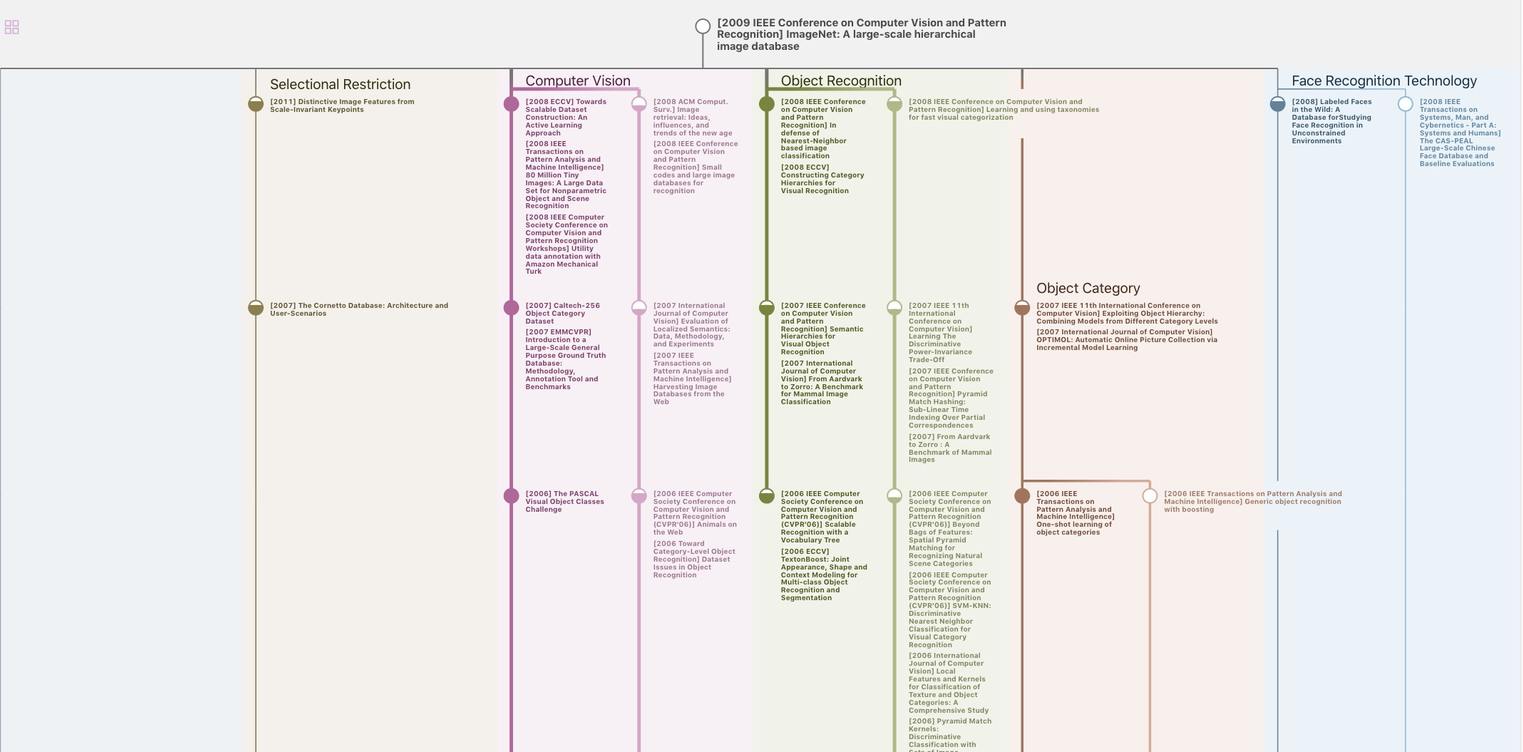
生成溯源树,研究论文发展脉络
Chat Paper
正在生成论文摘要