A Novel Radiomics Model Integrating Luminal and Mesenteric Features to Predict Mucosal Activity and Surgery Risk in Crohn's Disease Patients: A Multicenter Study.
ACADEMIC RADIOLOGY(2023)
摘要
BACKGROUND:To investigate the feasibility of integrating radiomics and morphological features based on computed tomography enterography (CTE) for developing a noninvasive grading model for mucosal activity and surgery risk of Crohn's disease (CD) patients.METHODS:A total of 167 patients from three centers were enrolled. Radiomics and image morphological features were extracted to quantify segmental and global simple endoscopic score for Crohn's disease (SES-CD). An image-fusion-based support vector machine (SVM) classifier was used for grading SES-CD and identifying moderate-to-severe SES-CD. The performance of the predictive model was assessed using the area under the receiver operating characteristic curve (AUC). A multiparametric model was developed to predict surgical progression in CD patients by combining sum-image scores and clinical data.RESULTS:The AUC values of the multicategorical segmental SES-CD fusion radiomic model based on a combination of luminal and mesenteric radiomics were 0.828 and 0.709 in training and validation cohorts. The image fusion model integrating the fusion radiomics and morphological features could accurately distinguish bowel segments with moderate-to-severe SES-CD in both the training cohort (AUC = 0.847, 95% confidence interval (CI): 0.784-0.902) and the validation cohort (AUC = 0.896, 95% CI: 0.812-0.960). A predictive nomogram for interval surgery was developed based on multivariable cox analysis.CONCLUSIONS:This study demonstrated the feasibility of integrating lumen and mesentery radiomic features to develop a promising noninvasive grading model for mucosal activity of CD. In combination with clinical data, the fusion-image score may yield an accurate prognostic model for time to surgery.
更多查看译文
关键词
Crohn's disease,computed tomography enterography,radiomics,deep learning,mesentery,surgery
AI 理解论文
溯源树
样例
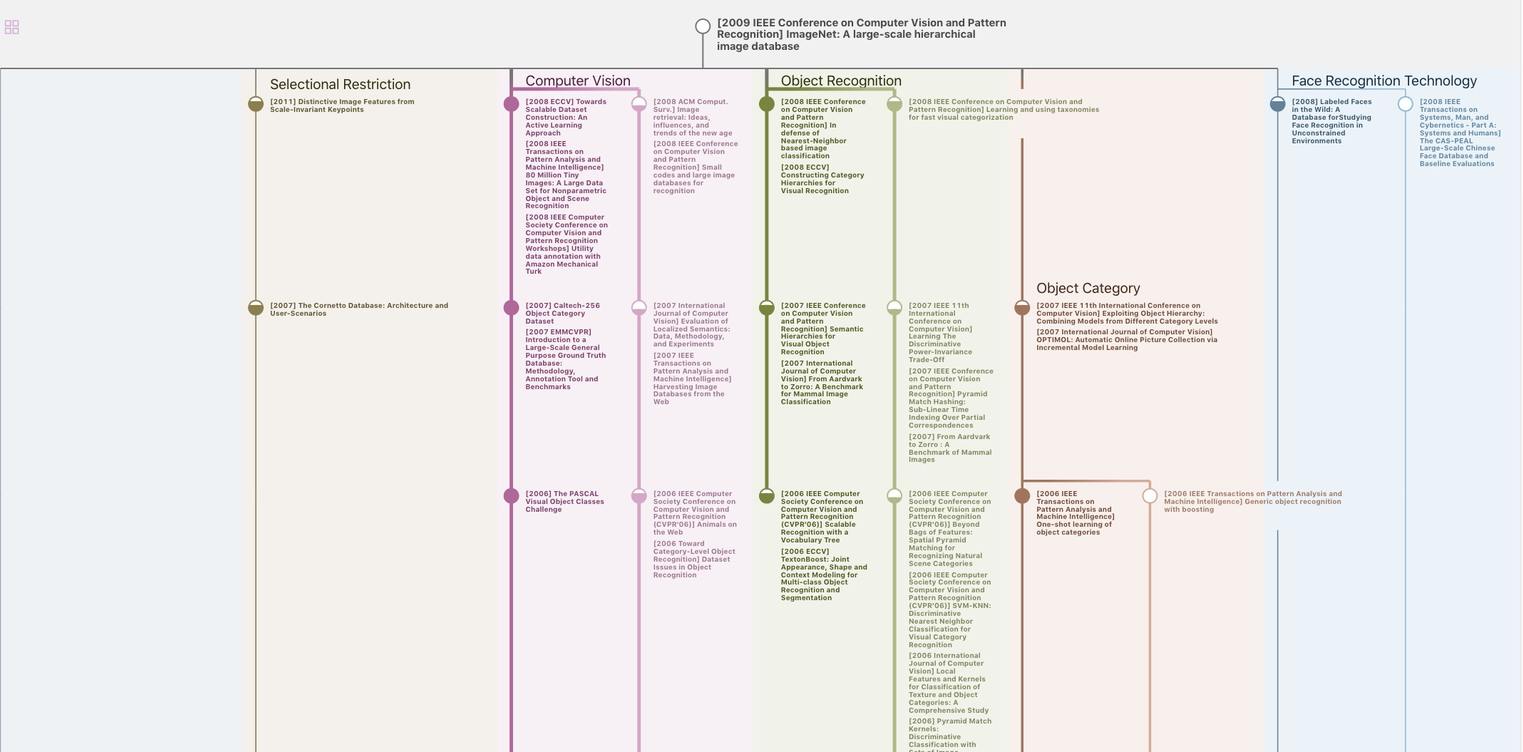
生成溯源树,研究论文发展脉络
Chat Paper
正在生成论文摘要