Limits of Model Selection under Transfer Learning
CoRR(2023)
摘要
Theoretical studies on transfer learning or domain adaptation have so far focused on situations with a known hypothesis class or model; however in practice, some amount of model selection is usually involved, often appearing under the umbrella term of hyperparameter-tuning: for example, one may think of the problem of tuning for the right neural network architecture towards a target task, while leveraging data from a related source task. Now, in addition to the usual tradeoffs on approximation vs estimation errors involved in model selection, this problem brings in a new complexity term, namely, the transfer distance between source and target distributions, which is known to vary with the choice of hypothesis class. We present a first study of this problem, focusing on classification; in particular, the analysis reveals some remarkable phenomena: adaptive rates, i.e., those achievable with no distributional information, can be arbitrarily slower than oracle rates, i.e., when given knowledge on distances.
更多查看译文
关键词
model selection,transfer,learning
AI 理解论文
溯源树
样例
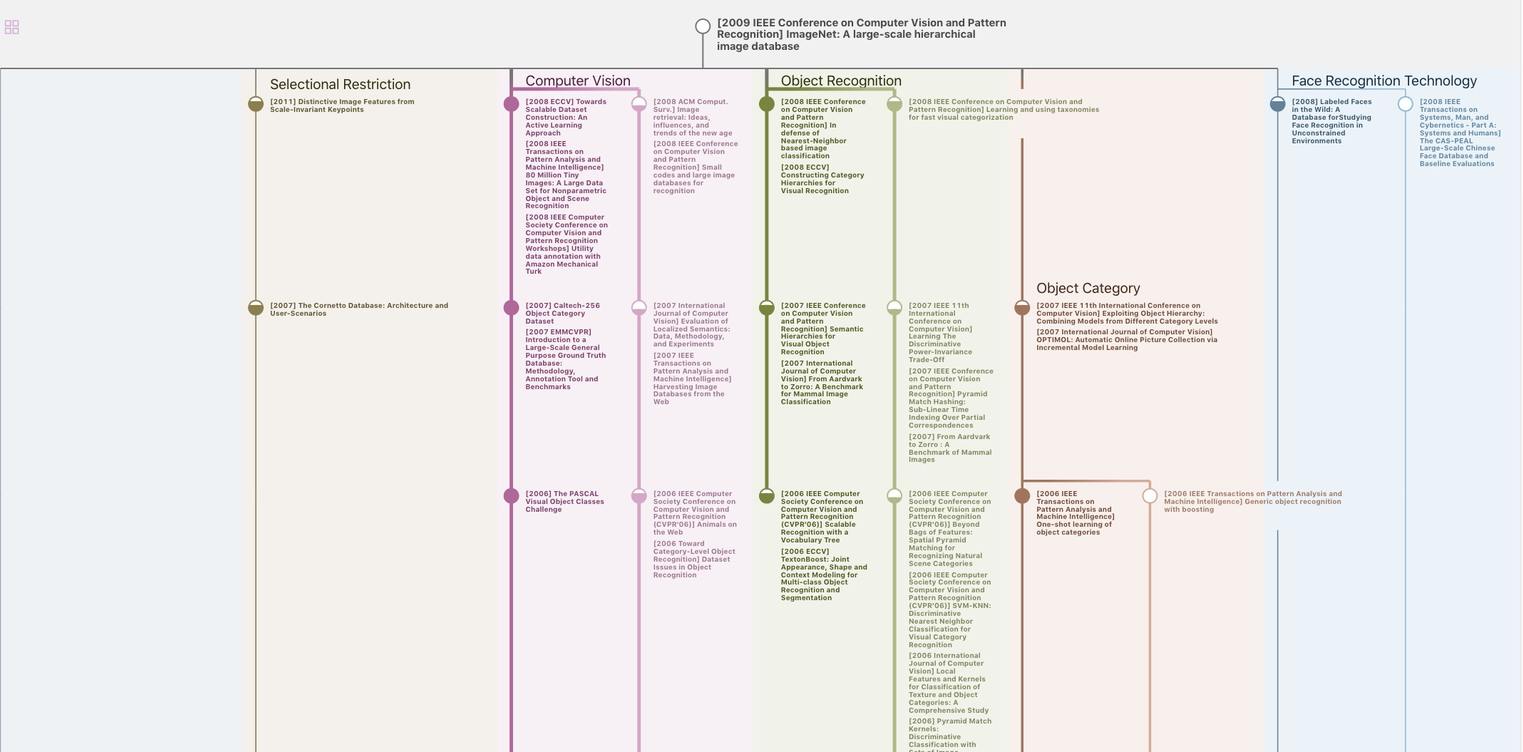
生成溯源树,研究论文发展脉络
Chat Paper
正在生成论文摘要