Analyzing drop coalescence in microfluidic device with a deep learning generative model
CoRR(2023)
摘要
Predicting drop coalescence based on process parameters is crucial for experiment design in chemical engineering. However, predictive models can suffer from the lack of training data and more importantly, the label imbalance problem. In this study, we propose the use of deep learning generative models to tackle this bottleneck by training the predictive models using generated synthetic data. A novel generative model, named double space conditional variational autoencoder (DSCVAE) is developed for labelled tabular data. By introducing label constraints in both the latent and the original space, DSCVAE is capable of generating consistent and realistic samples compared to standard conditional variational autoencoder (CVAE). Two predictive models, namely random forest and gradient boosting classifiers, are enhanced on synthetic data and their performances are evaluated on real experimental data. Numerical results show that considerable improvement in prediction accuracy can be achieved by using synthetic data and the proposed DSCVAE clearly outperforms the standard CVAE. This research clearly brings more insight into handling imbalanced data for classification problems, especially in chemical engineering
更多查看译文
关键词
microfluidic devices,drop coalescence,deep learning
AI 理解论文
溯源树
样例
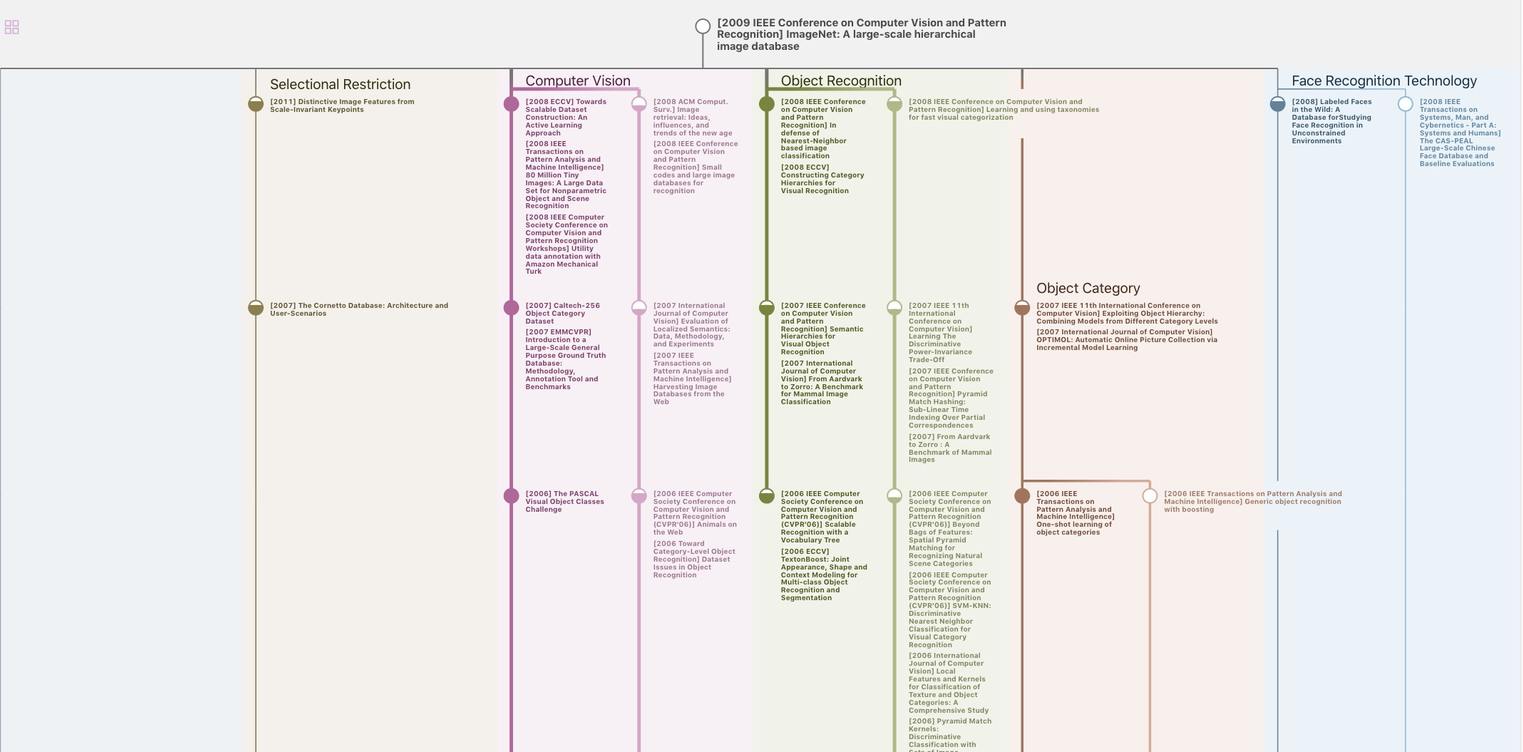
生成溯源树,研究论文发展脉络
Chat Paper
正在生成论文摘要