Predictions Based on Pixel Data: Insights from PDEs and Finite Differences
arxiv(2023)
Abstract
As supported by abundant experimental evidence, neural networks are
state-of-the-art for many approximation tasks in high-dimensional spaces.
Still, there is a lack of a rigorous theoretical understanding of what they can
approximate, at which cost, and at which accuracy. One network architecture of
practical use, especially for approximation tasks involving images, is
(residual) convolutional networks. However, due to the locality of the linear
operators involved in these networks, their analysis is more complicated than
that of fully connected neural networks. This paper deals with approximation of
time sequences where each observation is a matrix. We show that with relatively
small networks, we can represent exactly a class of numerical discretizations
of PDEs based on the method of lines. We constructively derive these results by
exploiting the connections between discrete convolution and finite difference
operators. Our network architecture is inspired by those typically adopted in
the approximation of time sequences. We support our theoretical results with
numerical experiments simulating the linear advection, heat, and Fisher
equations.
MoreTranslated text
AI Read Science
Must-Reading Tree
Example
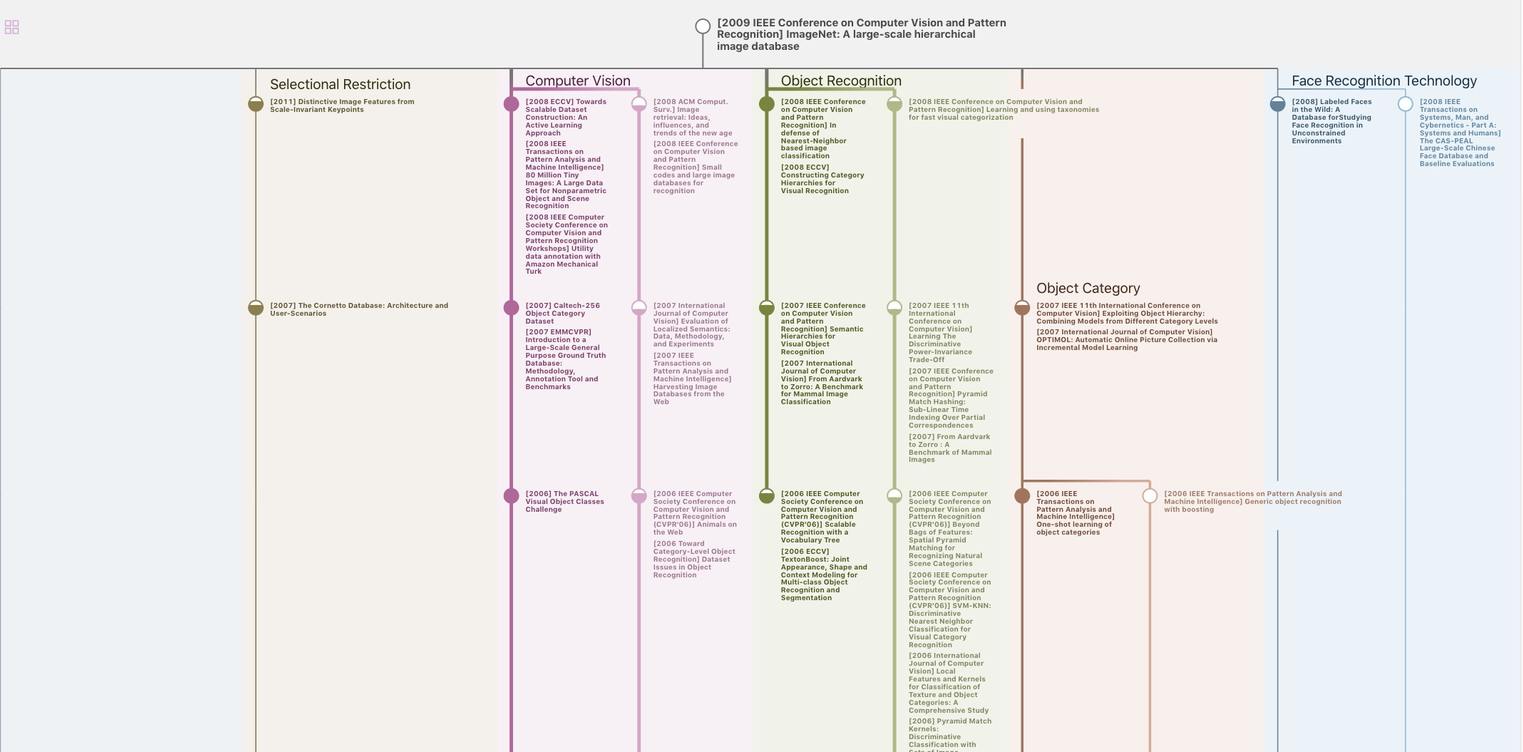
Generate MRT to find the research sequence of this paper
Chat Paper
Summary is being generated by the instructions you defined