Large Linguistic Models: Analyzing theoretical linguistic abilities of LLMs.
CoRR(2023)
摘要
The performance of large language models (LLMs) has recently improved to the point where the models can generate valid and coherent meta-linguistic analyses of data. This paper illustrates a vast potential for analyses of the meta-linguistic abilities of large language models. LLMs are primarily trained on language data in the form of text; analyzing their meta-linguistic abilities is informative both for our understanding of the general capabilities of LLMs as well as for models of linguistics. In this paper, we propose several types of experiments and prompt designs that allow us to analyze the ability of GPT-4 to generate meta-linguistic analyses. We focus on three linguistics subfields with formalisms that allow for a detailed analysis of GPT-4's theoretical capabilities: theoretical syntax, phonology, and semantics. We identify types of experiments, provide general guidelines, discuss limitations, and offer future directions for this research program.
更多查看译文
关键词
large linguistic models,theoretical linguistic abilities,llms
AI 理解论文
溯源树
样例
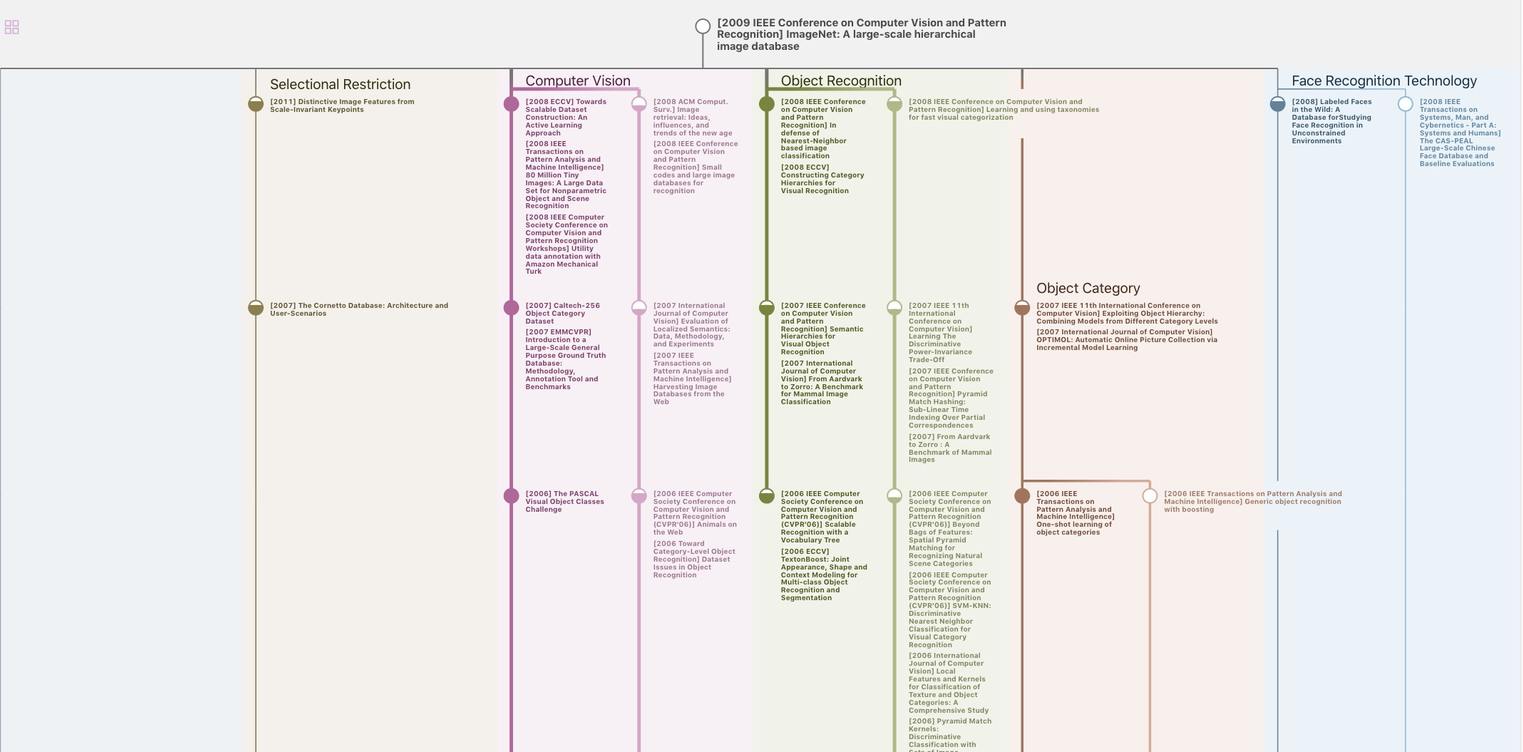
生成溯源树,研究论文发展脉络
Chat Paper
正在生成论文摘要