Performative Prediction with Bandit Feedback: Learning through Reparameterization
CoRR(2023)
摘要
Performative prediction, as introduced by Perdomo et al. (2020), is a framework for studying social prediction in which the data distribution itself changes in response to the deployment of a model. Existing work on optimizing accuracy in this setting hinges on two assumptions that are easily violated in practice: that the performative risk is convex over the deployed model, and that the mapping from the model to the data distribution is known to the model designer in advance. In this paper, we initiate the study of tractable performative prediction problems that do not require these assumptions. To tackle this more challenging setting, we develop a two-level zeroth-order optimization algorithm, where one level aims to compute the distribution map, and the other level reparameterizes the performative prediction objective as a function of the induced data distribution. Under mild conditions, this reparameterization allows us to transform the non-convex objective into a convex one and achieve provable regret guarantees. In particular, we provide a regret bound that is sublinear in the total number of performative samples taken and only polynomial in the dimension of the model parameter.
更多查看译文
关键词
bandit feedback,prediction,learning
AI 理解论文
溯源树
样例
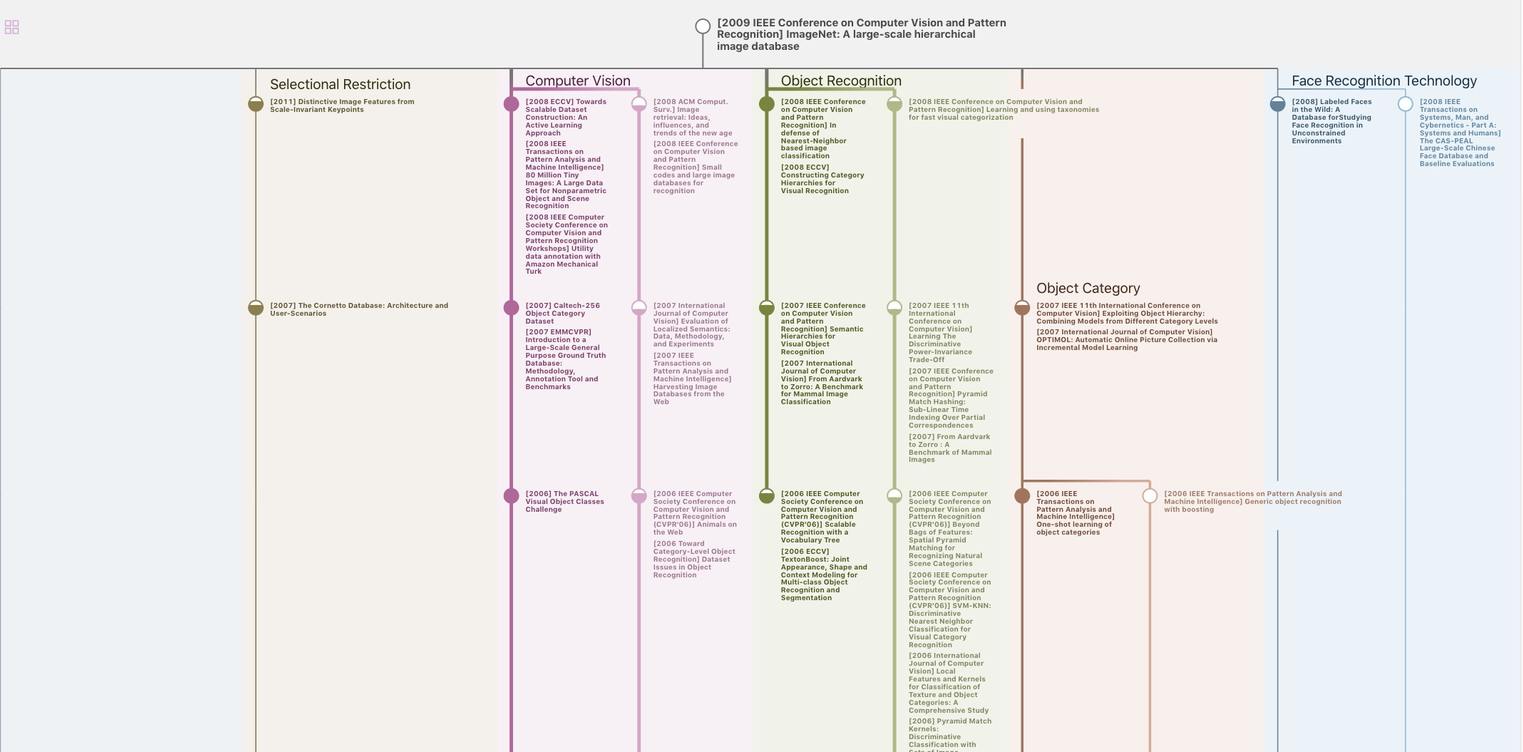
生成溯源树,研究论文发展脉络
Chat Paper
正在生成论文摘要