Complex Logical Reasoning over Knowledge Graphs Using Large Language Models
arXiv (Cornell University)(2023)
摘要
Reasoning over knowledge graphs (KGs) is a challenging task that requires adeep understanding of the complex relationships between entities and theunderlying logic of their relations. Current approaches rely on learninggeometries to embed entities in vector space for logical query operations, butthey suffer from subpar performance on complex queries and dataset-specificrepresentations. In this paper, we propose a novel decoupled approach,Language-guided Abstract Reasoning over Knowledge graphs (LARK), thatformulates complex KG reasoning as a combination of contextual KG search andlogical query reasoning, to leverage the strengths of graph extractionalgorithms and large language models (LLM), respectively. Our experimentsdemonstrate that the proposed approach outperforms state-of-the-art KGreasoning methods on standard benchmark datasets across several logical queryconstructs, with significant performance gain for queries of higher complexity.Furthermore, we show that the performance of our approach improvesproportionally to the increase in size of the underlying LLM, enabling theintegration of the latest advancements in LLMs for logical reasoning over KGs.Our work presents a new direction for addressing the challenges of complex KGreasoning and paves the way for future research in this area.
更多查看译文
关键词
Knowledge Graph Embedding,Knowledge Representation,Description Logics,Signal Processing on Graphs,Representation Learning
AI 理解论文
溯源树
样例
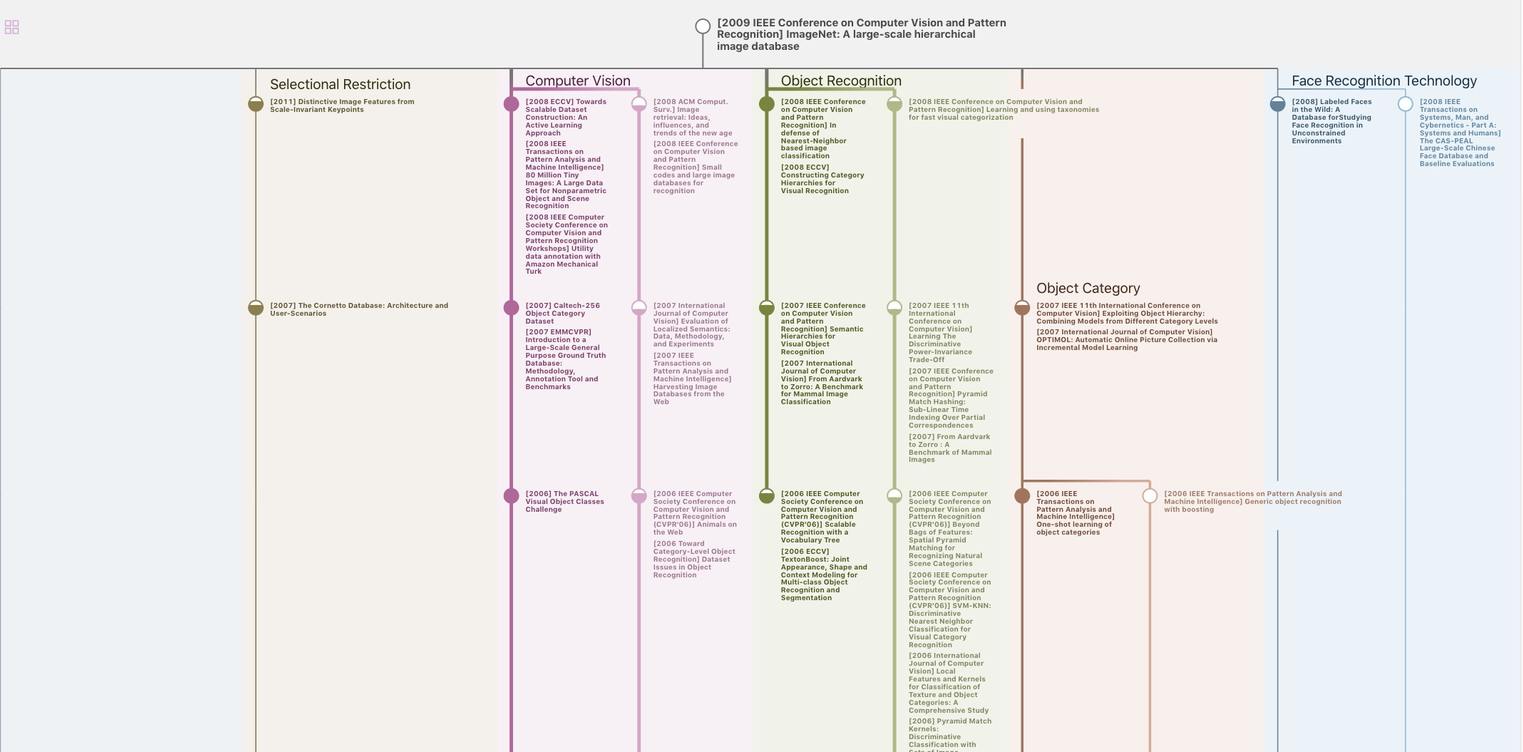
生成溯源树,研究论文发展脉络
Chat Paper
正在生成论文摘要