Solving Inverse Problems with Score-Based Generative Priors Learned from Noisy Data.
Asilomar Conference on Signals, Systems and Computers(2023)
摘要
We present SURE-Score: an approach for learning score-based generative modelsusing training samples corrupted by additive Gaussian noise. When a largetraining set of clean samples is available, solving inverse problems viascore-based (diffusion) generative models trained on the underlyingfully-sampled data distribution has recently been shown to outperformend-to-end supervised deep learning. In practice, such a large collection oftraining data may be prohibitively expensive to acquire in the first place. Inthis work, we present an approach for approximately learning a score-basedgenerative model of the clean distribution, from noisy training data. Weformulate and justify a novel loss function that leverages Stein's unbiasedrisk estimate to jointly denoise the data and learn the score function viadenoising score matching, while using only the noisy samples. We demonstratethe generality of SURE-Score by learning priors and applying posterior samplingto ill-posed inverse problems in two practical applications from differentdomains: compressive wireless multiple-input multiple-output channel estimationand accelerated 2D multi-coil magnetic resonance imaging reconstruction, wherewe demonstrate competitive reconstruction performance when learning atsignal-to-noise ratio values of 0 and 10 dB, respectively.
更多查看译文
AI 理解论文
溯源树
样例
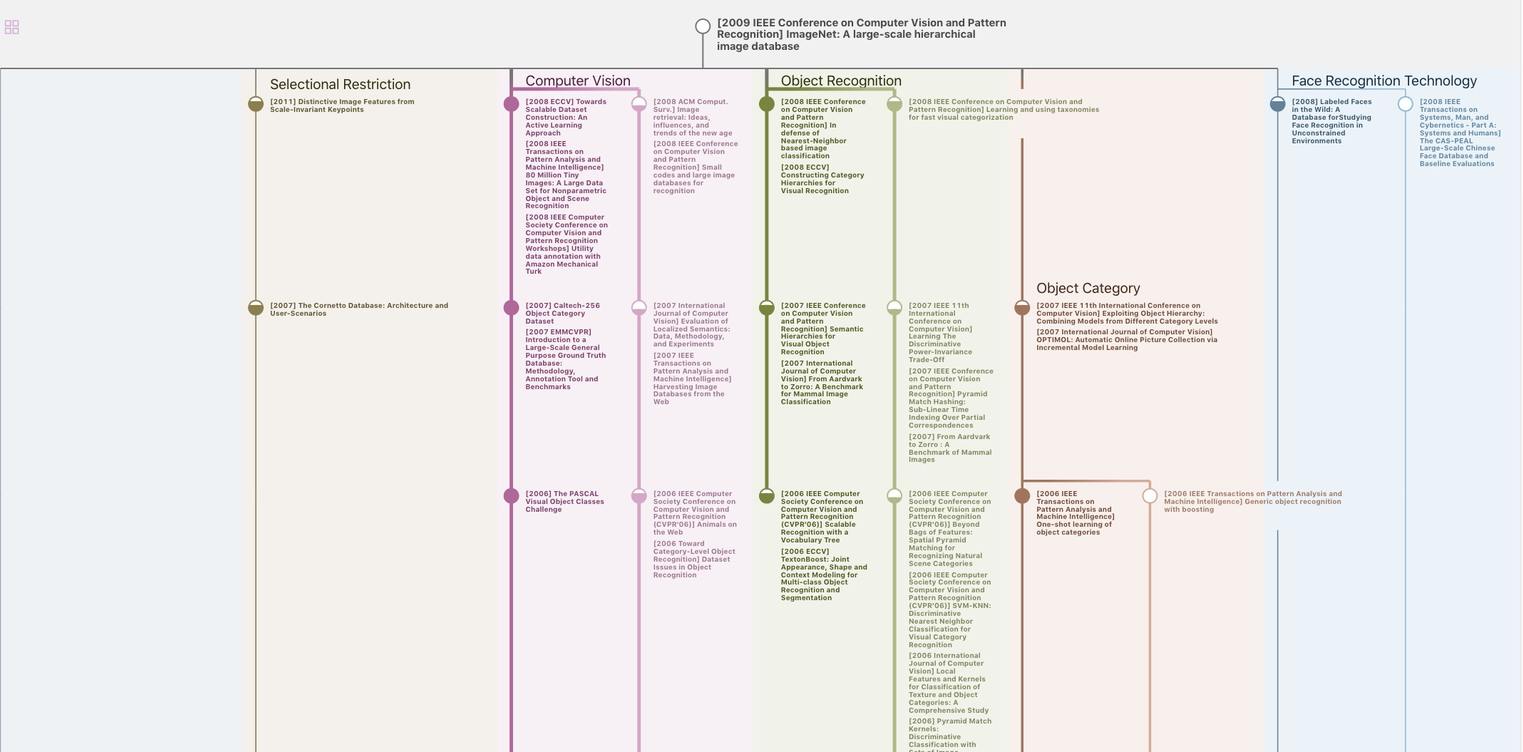
生成溯源树,研究论文发展脉络
Chat Paper
正在生成论文摘要