Multitask learning in Audio Captioning: a sentence embedding regression loss acts as a regularizer
CoRR(2023)
摘要
In this work, we propose to study the performance of a model trained with a sentence embedding regression loss component for the Automated Audio Captioning task. This task aims to build systems that can describe audio content with a single sentence written in natural language. Most systems are trained with the standard Cross-Entropy loss, which does not take into account the semantic closeness of the sentence. We found that adding a sentence embedding loss term reduces overfitting, but also increased SPIDEr from 0.397 to 0.418 in our first setting on the AudioCaps corpus. When we increased the weight decay value, we found our model to be much closer to the current state-of-the-art methods, with a SPIDEr score up to 0.444 compared to a 0.475 score. Moreover, this model uses eight times less trainable parameters. In this training setting, the sentence embedding loss has no more impact on the model performance.
更多查看译文
关键词
sound event description,multitask learning,audio language task,overfitting,sentence embedding regression loss,semantic loss
AI 理解论文
溯源树
样例
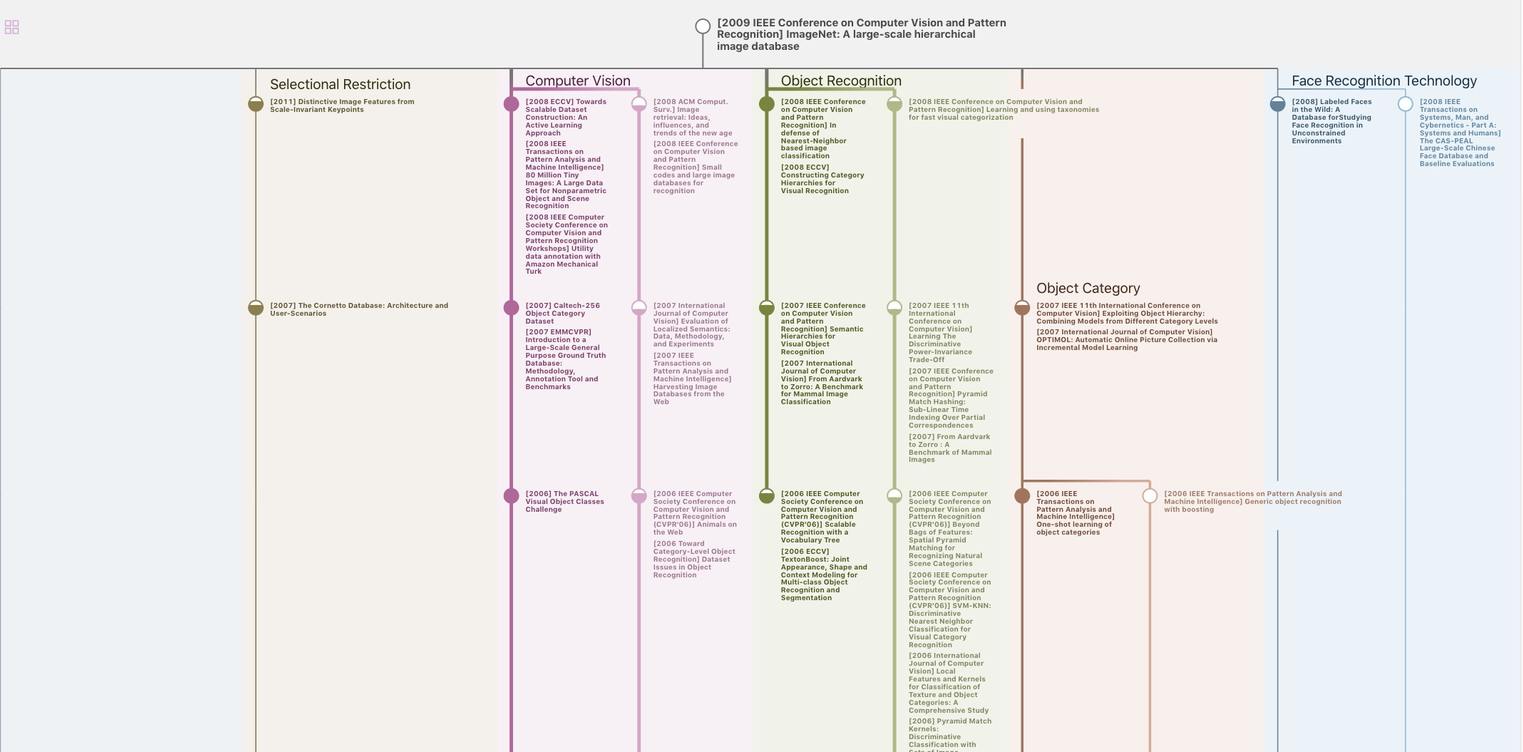
生成溯源树,研究论文发展脉络
Chat Paper
正在生成论文摘要