Calibrated Explanations: with Uncertainty Information and Counterfactuals
arXivorg(2023)
摘要
While local explanations for AI models can offer insights into individual predictions, such as feature importance, they are plagued by issues like instability. The unreliability of feature weights, often skewed due to poorly calibrated ML models, deepens these challenges. Moreover, the critical aspect of feature importance uncertainty remains mostly unaddressed in Explainable AI (XAI). The novel feature importance explanation method presented in this paper, called Calibrated Explanations (CE), is designed to tackle these issues head-on. Built on the foundation of Venn-Abers, CE not only calibrates the underlying model but also delivers reliable feature importance explanations with an exact definition of the feature weights. CE goes beyond conventional solutions by addressing output uncertainty. It accomplishes this by providing uncertainty quantification for both feature weights and the model’s probability estimates. Additionally, CE is model-agnostic, featuring easily comprehensible conditional rules and the ability to generate counterfactual explanations with embedded uncertainty quantification. Results from an evaluation with 25 benchmark datasets underscore the efficacy of CE, making it stand as a fast, reliable, stable, and robust solution.
更多查看译文
关键词
Explainable AI,Feature importance,Calibrated explanations,Venn-Abers,Uncertainty quantification,Counterfactual explanations
AI 理解论文
溯源树
样例
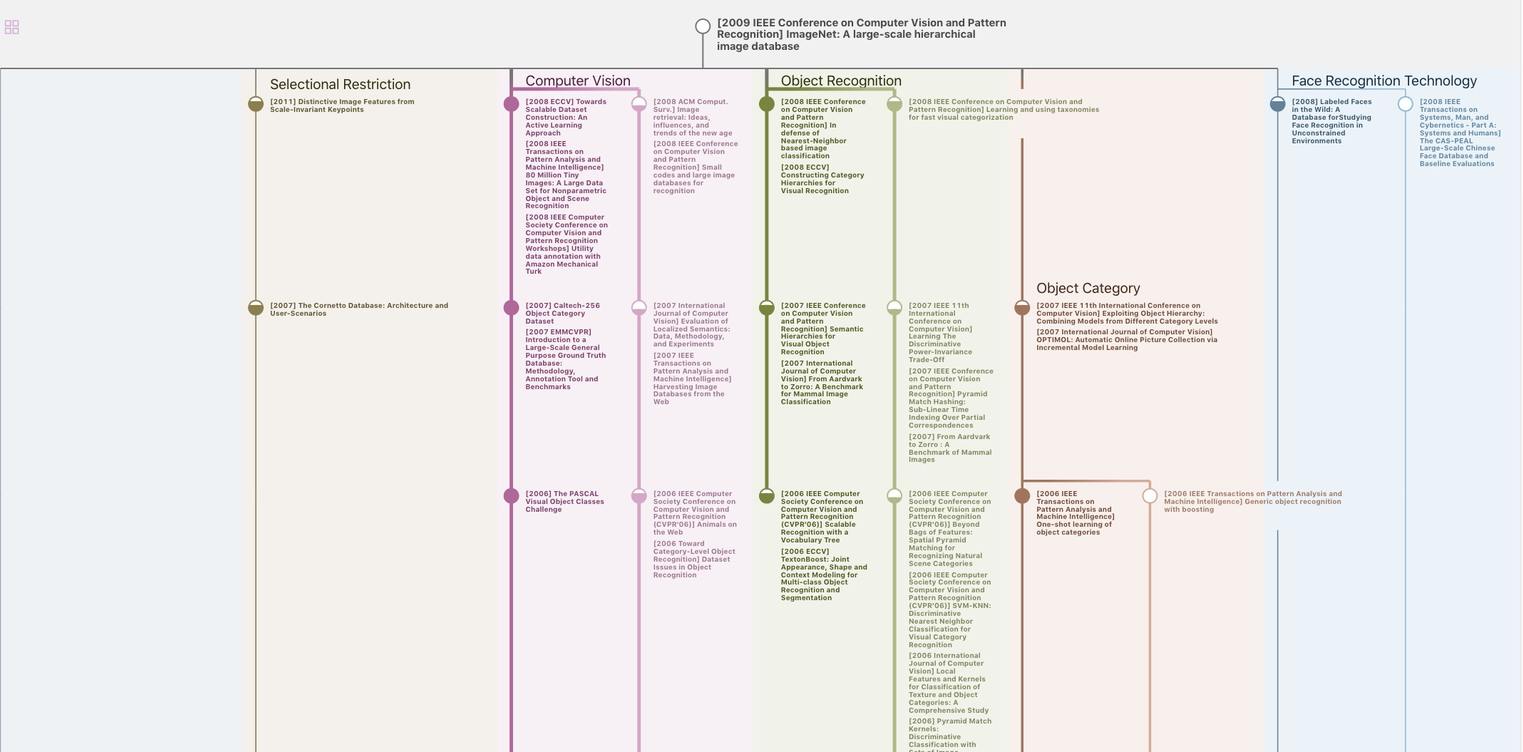
生成溯源树,研究论文发展脉络
Chat Paper
正在生成论文摘要