Defending against Insertion-based Textual Backdoor Attacks via Attribution
conf_acl(2023)
摘要
Textual backdoor attack, as a novel attack model, has been shown to be effective in adding a backdoor to the model during training. Defending against such backdoor attacks has become urgent and important. In this paper, we propose AttDef, an efficient attribution-based pipeline to defend against two insertion-based poisoning attacks, BadNL and InSent. Specifically, we regard the tokens with larger attribution scores as potential triggers since larger attribution words contribute more to the false prediction results and therefore are more likely to be poison triggers. Additionally, we further utilize an external pre-trained language model to distinguish whether input is poisoned or not. We show that our proposed method can generalize sufficiently well in two common attack scenarios (poisoning training data and testing data), which consistently improves previous methods. For instance, AttDef can successfully mitigate both attacks with an average accuracy of 79.97% (56.59% up) and 48.34% (3.99% up) under pre-training and post-training attack defense respectively, achieving the new state-of-the-art performance on prediction recovery over four benchmark datasets.
更多查看译文
关键词
textual backdoor attacks,insertion-based
AI 理解论文
溯源树
样例
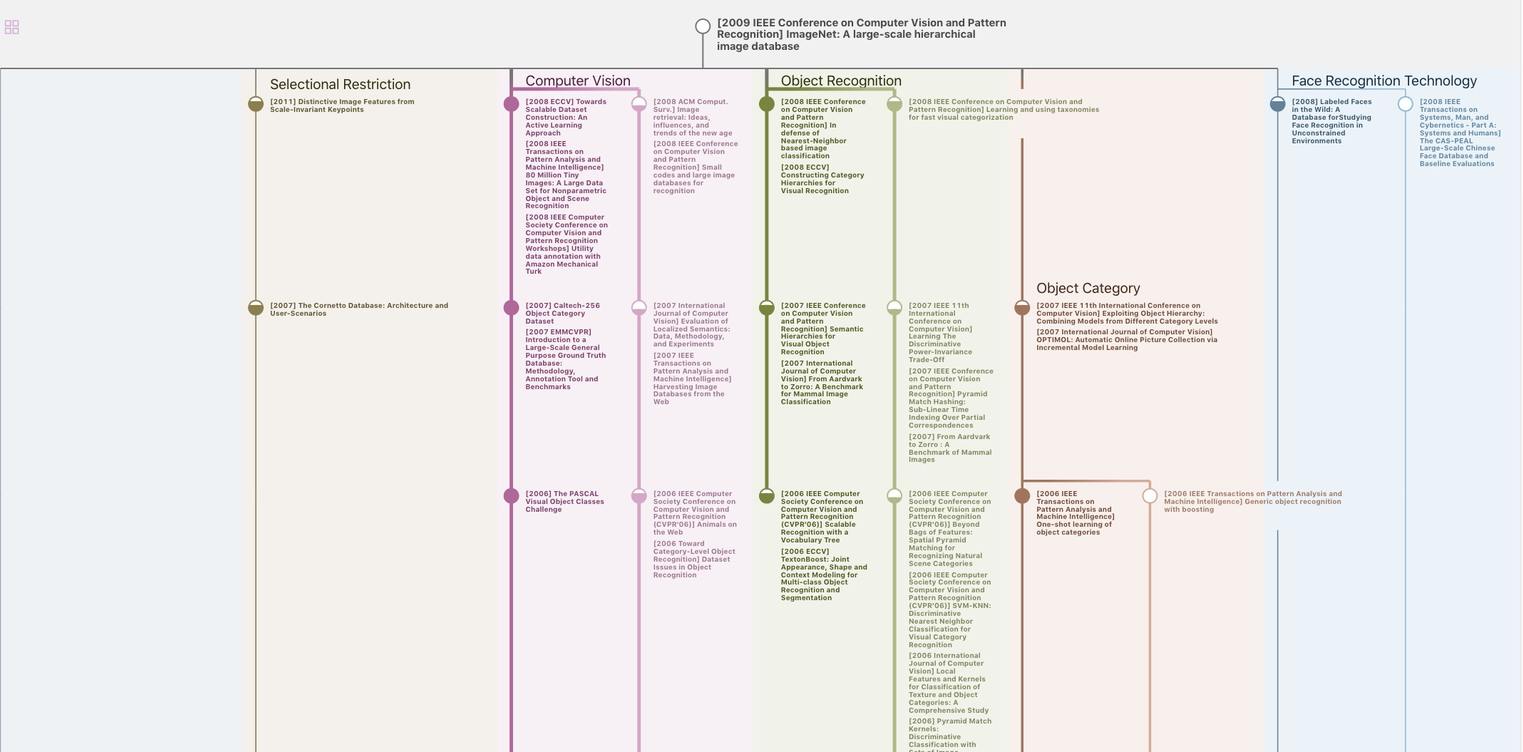
生成溯源树,研究论文发展脉络
Chat Paper
正在生成论文摘要