Uncertainty Quantification and Confidence Intervals for Naive Rare-Event Estimators
Journal of applied probability(2024)SCI 4区
Abstract
We consider the estimation of rare-event probabilities using sample proportions output by naive Monte Carlo or collected data. Unlike using variance reduction techniques, this naive estimator does not have an a priori relative efficiency guarantee. On the other hand, due to the recent surge of sophisticated rare-event problems arising in safety evaluations of intelligent systems, efficiency-guaranteed variance reduction may face implementation challenges which, coupled with the availability of computation or data collection power, motivate the use of such a naive estimator. In this paper we study the uncertainty quantification, namely the construction, coverage validity, and tightness of confidence intervals, for rare-event probabilities using only sample proportions. In addition to the known normality, Wilson, and exact intervals, we investigate and compare them with two new intervals derived from Chernoff's inequality and the Berry-Esseen theorem. Moreover, we generalize our results to the natural situation where sampling stops by reaching a target number of rare-event hits. Our findings show that the normality and Wilson intervals are not always valid, but they are close to the newly developed valid intervals in terms of half-width. In contrast, the exact interval is conservative, but safely guarantees the attainment of the nominal confidence level. Our new intervals, while being more conservative than the exact interval, provide useful insights into understanding the tightness of the considered intervals.
MoreTranslated text
Key words
Rare-event estimation,confidence interval,relative error,sample proportion
PDF
View via Publisher
AI Read Science
AI Summary
AI Summary is the key point extracted automatically understanding the full text of the paper, including the background, methods, results, conclusions, icons and other key content, so that you can get the outline of the paper at a glance.
Example
Background
Key content
Introduction
Methods
Results
Related work
Fund
Key content
- Pretraining has recently greatly promoted the development of natural language processing (NLP)
- We show that M6 outperforms the baselines in multimodal downstream tasks, and the large M6 with 10 parameters can reach a better performance
- We propose a method called M6 that is able to process information of multiple modalities and perform both single-modal and cross-modal understanding and generation
- The model is scaled to large model with 10 billion parameters with sophisticated deployment, and the 10 -parameter M6-large is the largest pretrained model in Chinese
- Experimental results show that our proposed M6 outperforms the baseline in a number of downstream tasks concerning both single modality and multiple modalities We will continue the pretraining of extremely large models by increasing data to explore the limit of its performance
Try using models to generate summary,it takes about 60s
Must-Reading Tree
Example
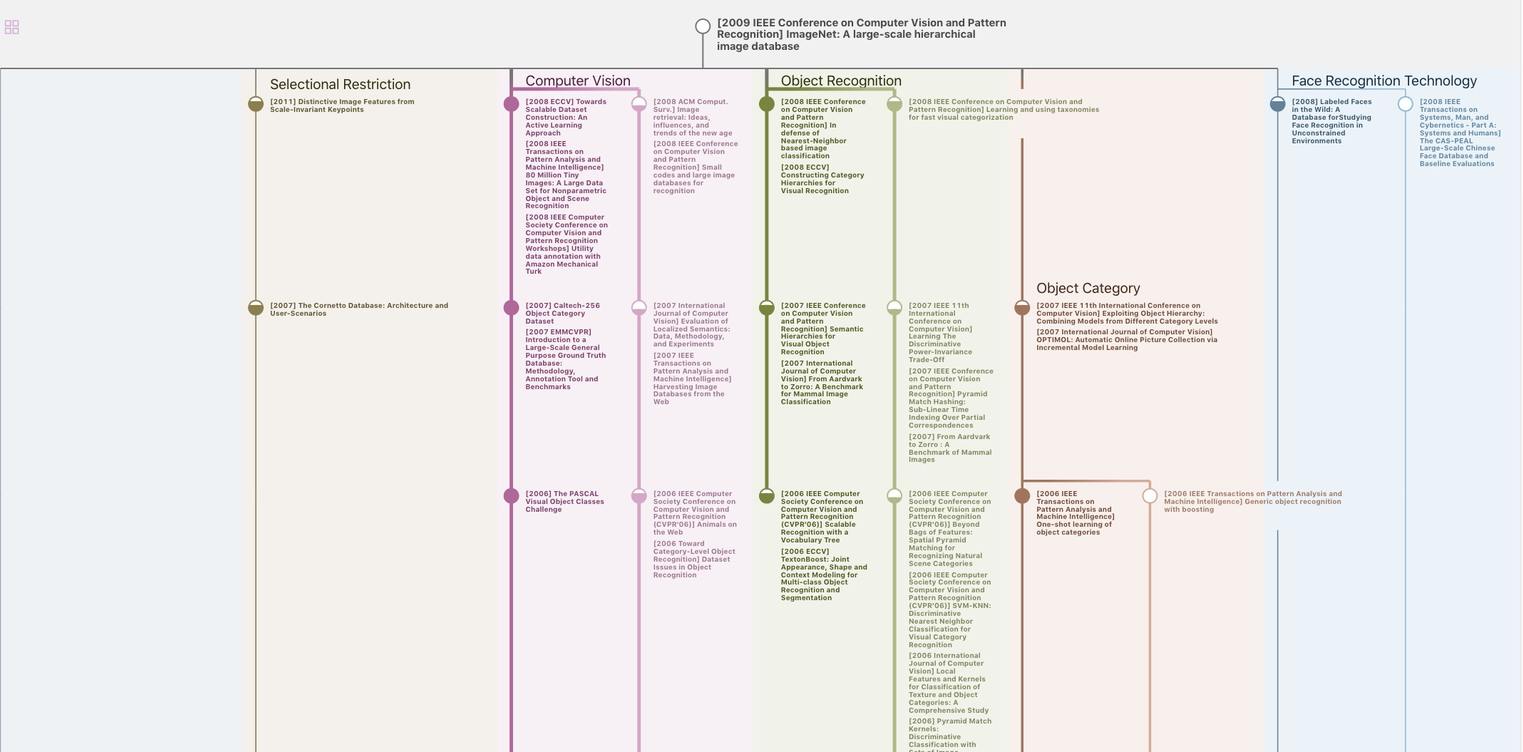
Generate MRT to find the research sequence of this paper
Related Papers
1993
被引用63 | 浏览
2002
被引用54 | 浏览
2008
被引用15 | 浏览
1991
被引用130 | 浏览
2012
被引用44 | 浏览
2017
被引用409 | 浏览
Data Disclaimer
The page data are from open Internet sources, cooperative publishers and automatic analysis results through AI technology. We do not make any commitments and guarantees for the validity, accuracy, correctness, reliability, completeness and timeliness of the page data. If you have any questions, please contact us by email: report@aminer.cn
Chat Paper
GPU is busy, summary generation fails
Rerequest