Radiance Field Gradient Scaling for Unbiased Near-Camera Training
CoRR(2023)
摘要
NeRF acquisition typically requires careful choice of near planes for the different cameras or suffers from background collapse, creating floating artifacts on the edges of the captured scene. The key insight of this work is that background collapse is caused by a higher density of samples in regions near cameras. As a result of this sampling bias, near-camera volumes receive significantly more gradients, leading to incorrect density buildup. We propose a gradient scaling approach to counter-balance this bias, removing the need for near planes, while preventing background collapse. Our method can be implemented in a few lines, does not induce any significant overhead, and is compatible with most NeRF implementations.
更多查看译文
AI 理解论文
溯源树
样例
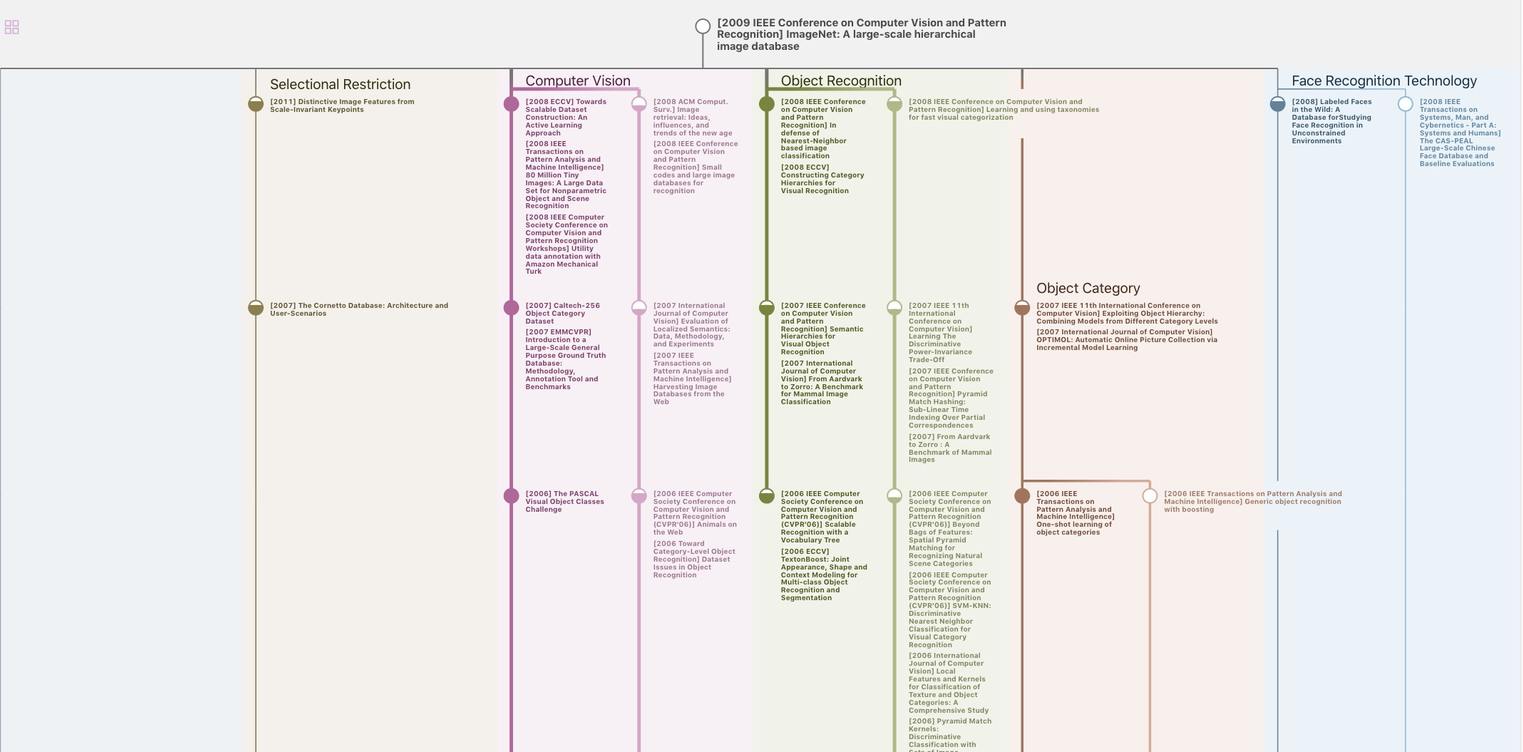
生成溯源树,研究论文发展脉络
Chat Paper
正在生成论文摘要