Disentangled Contrastive Collaborative Filtering
PROCEEDINGS OF THE 46TH INTERNATIONAL ACM SIGIR CONFERENCE ON RESEARCH AND DEVELOPMENT IN INFORMATION RETRIEVAL, SIGIR 2023(2023)
摘要
Recent studies show that graph neural networks (GNNs) are prevalent to model high-order relationships for collaborative filtering (CF). Towards this research line, graph contrastive learning (GCL) has exhibited powerful performance in addressing the supervision label shortage issue by learning augmented user and item representations. While many of them show their effectiveness, two key questions still remain unexplored: i) Most existing GCL-based CF models are still limited by ignoring the fact that user-item interaction behaviors are often driven by diverse latent intent factors (e.g., shopping for family party, preferred color or brand of products); ii) Their introduced non-adaptive augmentation techniques are vulnerable to noisy information, which raises concerns about the model's robustness and the risk of incorporating misleading self-supervised signals. In light of these limitations, we propose a Disentangled Contrastive Collaborative Filtering framework (DCCF) to realize intent disentanglement with self-supervised augmentation in an adaptive fashion. With the learned disentangled representations with global context, our DCCF is able to not only distill finer-grained latent factors from the entangled self-supervision signals but also alleviate the augmentation-induced noise. Finally, the cross-view contrastive learning task is introduced to enable adaptive augmentation with our parameterized interaction mask generator. Experiments on various public datasets demonstrate the superiority of our method compared to existing solutions. Our model implementation is released at the link https://github.com/HKUDS/DCCF.
更多查看译文
关键词
Collaborative Filtering,Contrastive Learning,Disentangled Representation,Graph Neural Networks,Recommendation
AI 理解论文
溯源树
样例
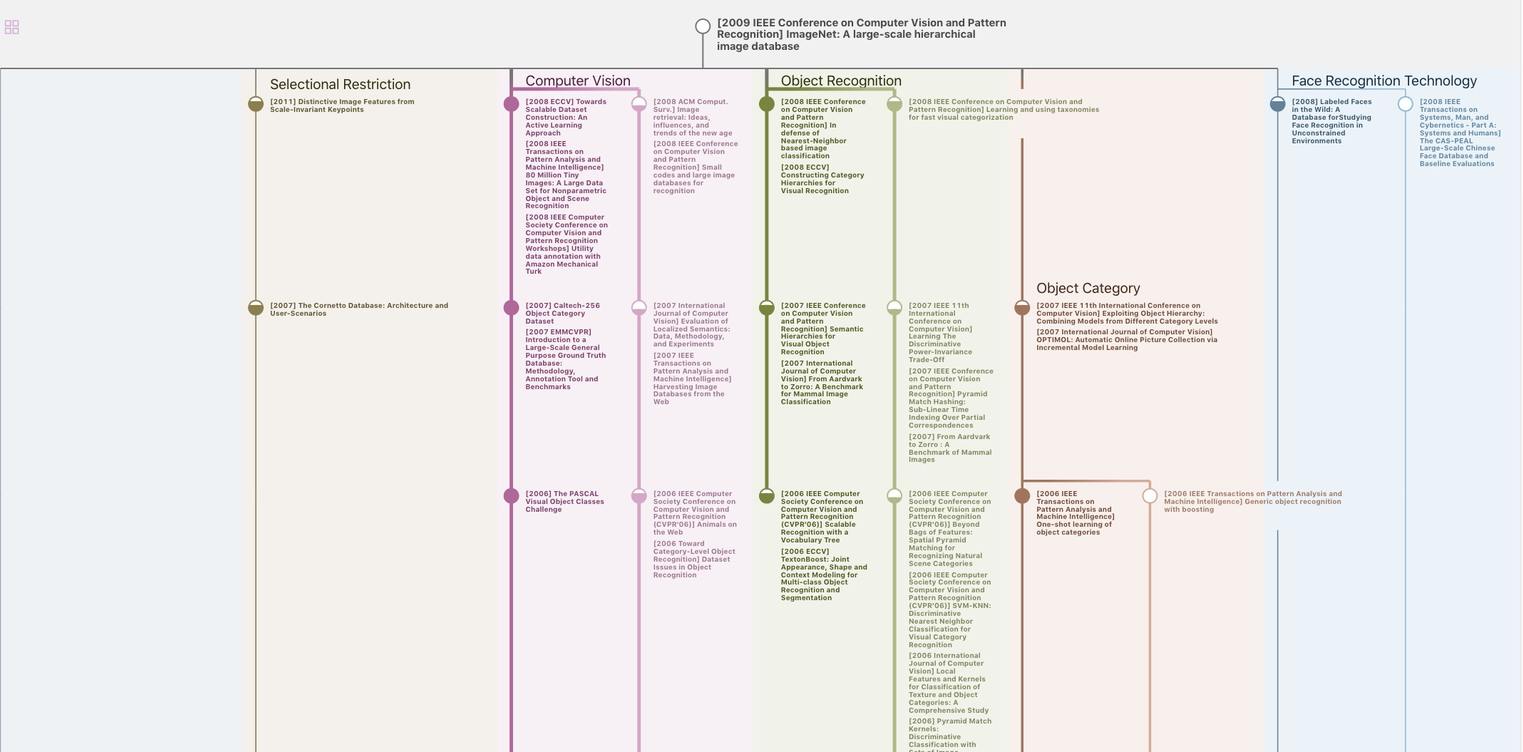
生成溯源树,研究论文发展脉络
Chat Paper
正在生成论文摘要