FUSegNet: A deep convolutional neural network for foot ulcer segmentation
BIOMEDICAL SIGNAL PROCESSING AND CONTROL(2024)
摘要
This paper presents FUSegNet, a new model for foot ulcer segmentation in diabetes patients, which uses the pretrained EfficientNet-b7 as a backbone to address the issue of limited training samples. A modified spatial and channel squeeze-and-excitation (scSE) module called parallel scSE or P-scSE is proposed that combines additive and max-out scSE. A new arrangement is introduced for the module by fusing it in the middle of each decoder stage. As the top decoder stage carries a limited number of feature maps, max-out scSE is bypassed there to form a shorted P-scSE. A set of augmentations, comprising geometric, morphological, and intensity-based augmentations, is applied before feeding the data into the network. The proposed model is first evaluated on a publicly available chronic wound dataset where it achieves a data-based dice score of 92.70%, which is the highest score among the reported approaches. The model outperforms other scSE-based U-Net models in terms of Pratt's figure of merits (PFOM) scores in most categories, which evaluates the accuracy of edge localization. The model is then tested in the MICCAI 2021 FUSeg challenge, where a variation of FUSegNet called x-FUSegNet is submitted. The x-FUSegNet model, which takes the average of outputs obtained by FUSegNet using 5-fold cross-validation, achieves a dice score of 89.23%, placing it at the top of the FUSeg Challenge leaderboard. The source code for the model is available at https://github.com/mrinal054/FUSegNet.
更多查看译文
关键词
Chronic wounds,Foot ulcers,Deep learning,Image segmentation,FUSeg Challenge 2021
AI 理解论文
溯源树
样例
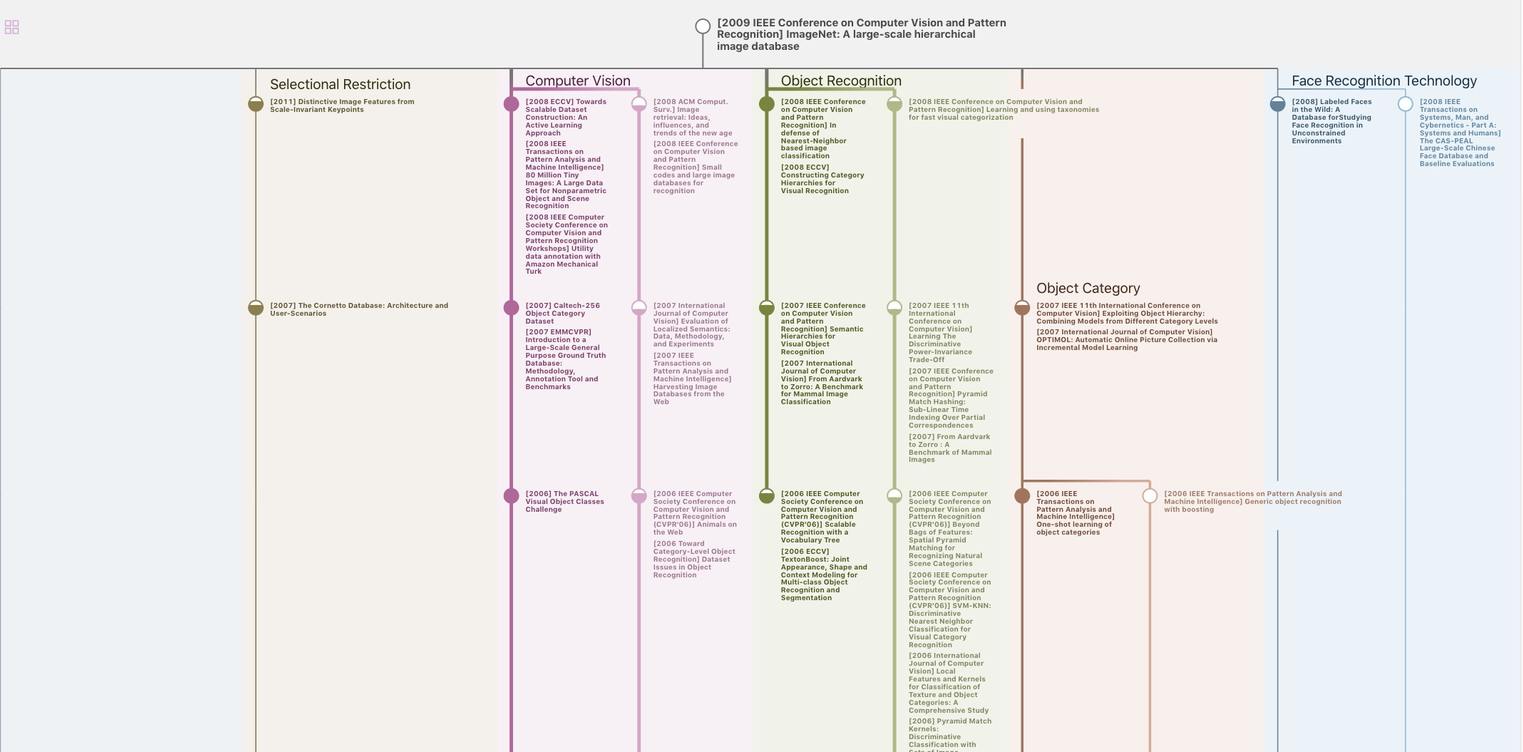
生成溯源树,研究论文发展脉络
Chat Paper
正在生成论文摘要