Semi-Supervised Domain-Adaptive Seizure Prediction via Feature Alignment and Consistency Regularization.
IEEE Trans. Instrum. Meas.(2023)
摘要
The interpatient variability still poses a great challenge for the real-world application of electroencephalogram (EEG)-based seizure prediction, where most previous methods could only work under the patient-specific fashion and fail to generalize across patients. To address this issue, some latest studies applied supervised domain adaptation (SDA), utilizing limited labeled data from the target patient to calibrate the model. However, as the epileptic EEG representation even varies within one single patient, limited target data could hardly cover the data distribution, thus the model still shows poor generalization on the target patient. To this end, we introduce a novel semi-supervised domain adaptive seizure prediction model (SSDA-SPM), using limited labeled target data and extra unlabeled target data for adaptation. SSDA-SPM mainly consists of two unsupervised modules, namely feature alignment (FA) module and consistency regularization (CR) module. The FA module aims to transfer knowledge from existing patients to the target patient coarsely by globally aligning the data distribution between them. Then the CR module further enhances the discriminability on the target patient by pushing the decision boundary into the low-density area. Our proposed method achieves 88.8% sensitivity (Sens), 0.182/h false prediction rate (FPR), and 0.849 area under the receiver operating characteristic curve (AUC) on the CHB-MIT database and 75.7% Sens, 0.165/h FPR, and 0.763 AUC on the Kaggle database. Experimental results demonstrate that our method has provided a promising solution to improve the cross-patient generalization for seizure prediction.
更多查看译文
关键词
Consistency regularization (CR),electroen-cephalogram (EEG),feature alignment (FA),seizure prediction,semi-supervised domain adaptation (SSDA)
AI 理解论文
溯源树
样例
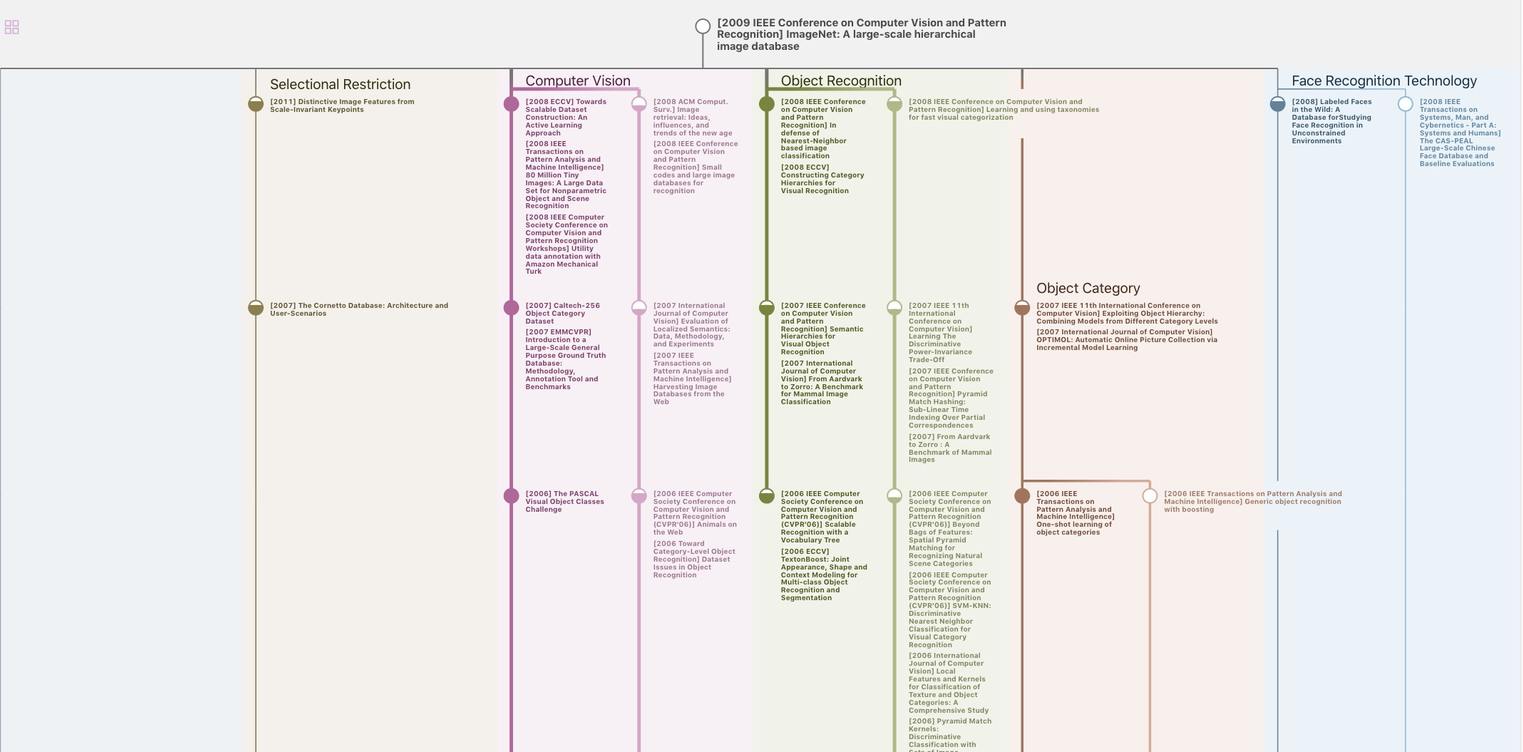
生成溯源树,研究论文发展脉络
Chat Paper
正在生成论文摘要