Scene Augmentation Methods for Interactive Embodied AI Tasks.
IEEE Trans. Instrum. Meas.(2023)
摘要
The emerging embodied artificial intelligence (AI) paradigm enables intelligent robots to learn like humans from interaction, and is thus considered an effective way approach to general AI. Unfortunately, even the best-performing agents still overfit and generalize poorly to unseen scenes, due to the limited scenes provided by embodied AI simulators. To alleviate this issue, we propose a scene augmentation (SA) strategy to scale up the scene diversity for interactive tasks and make the interactions more like the real world. Compared to existing methods focusing on improving diversity in observation space, our approach aims to automatically derive a new distribution of scene layout or object states to provide sufficient conditional transfer models for the agent to learn environmental invariant and irrelevant features through interaction. Specifically, we provide four representative and systematical SA methods that can derive scene variants for entities from different levels of a scene graph. We demonstrate the efficiency of our methods in the popular embodied AI simulator iGibson. To verify the effectiveness of interactive agents, we also conduct two representative interactive tasks with a proposed continuous action parameterized method. The evaluation results show that our SA strategy can boost the performance of interactive agents and generalize well to unseen scenes. Finally, we present a systematic generalization analysis using the proposed methods to explicitly estimate the ability of agents to generalize to new layouts, new objects, and new object states. We claim that the proposed methods are not limited to iGibson and can be extended to other interactive simulators. The code and additional information are available at: https://github.com/sanghongrui/SceneAug.
更多查看译文
关键词
Continuous action parameterization,embodied artificial intelligence (AI),interactive embodied AI tasks,scene augmentation (SA),systematic generalization estimation
AI 理解论文
溯源树
样例
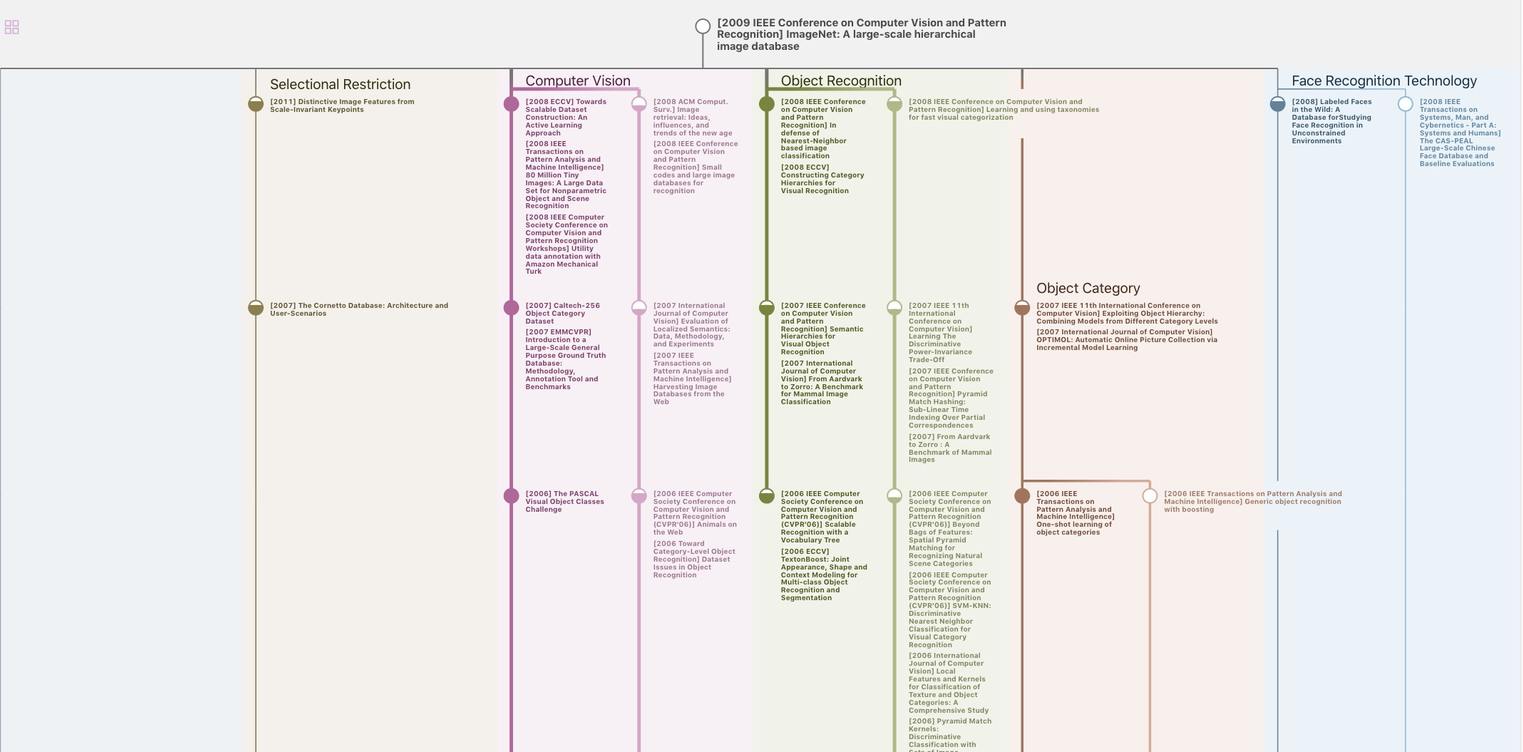
生成溯源树,研究论文发展脉络
Chat Paper
正在生成论文摘要