End-to-end learning of representations for instance-level document image retrieval
Appl. Soft Comput.(2023)
摘要
Instance-level document image retrieval plays a vital role in many document image processing systems. An appropriate image representation is of paramount importance for effective retrieval. To this end, we propose an image representation that is well-suited for the instance-level document image retrieval task. A novel end-to-end three-stream Siamese network is presented to learn the image representation, which accepts a triplet: a query image, its matching image and its non-matching image. The network is trained to jointly minimize two types of loss: ranking loss and classification loss. By employing the ranking loss, the distance between the representations of the query image and its matching image can be explicitly forced to be smaller than that between the query image and its non-matching image. Besides, each stream of the network is further extended as a classification model to fully exploit the supervised information of each individual image. The cross-entropy loss is then employed for the classification model. After training, an arbitrary image can be fed to either stream of the network to generate its representation. Extensive comparison and ablation experiments on three datasets have demonstrated the effectiveness of the proposed image representation. The two types of loss have been shown to complement each other.
更多查看译文
关键词
Instance-level document image retrieval,Image representation,Three-stream siamese network,Ranking loss,Classification loss
AI 理解论文
溯源树
样例
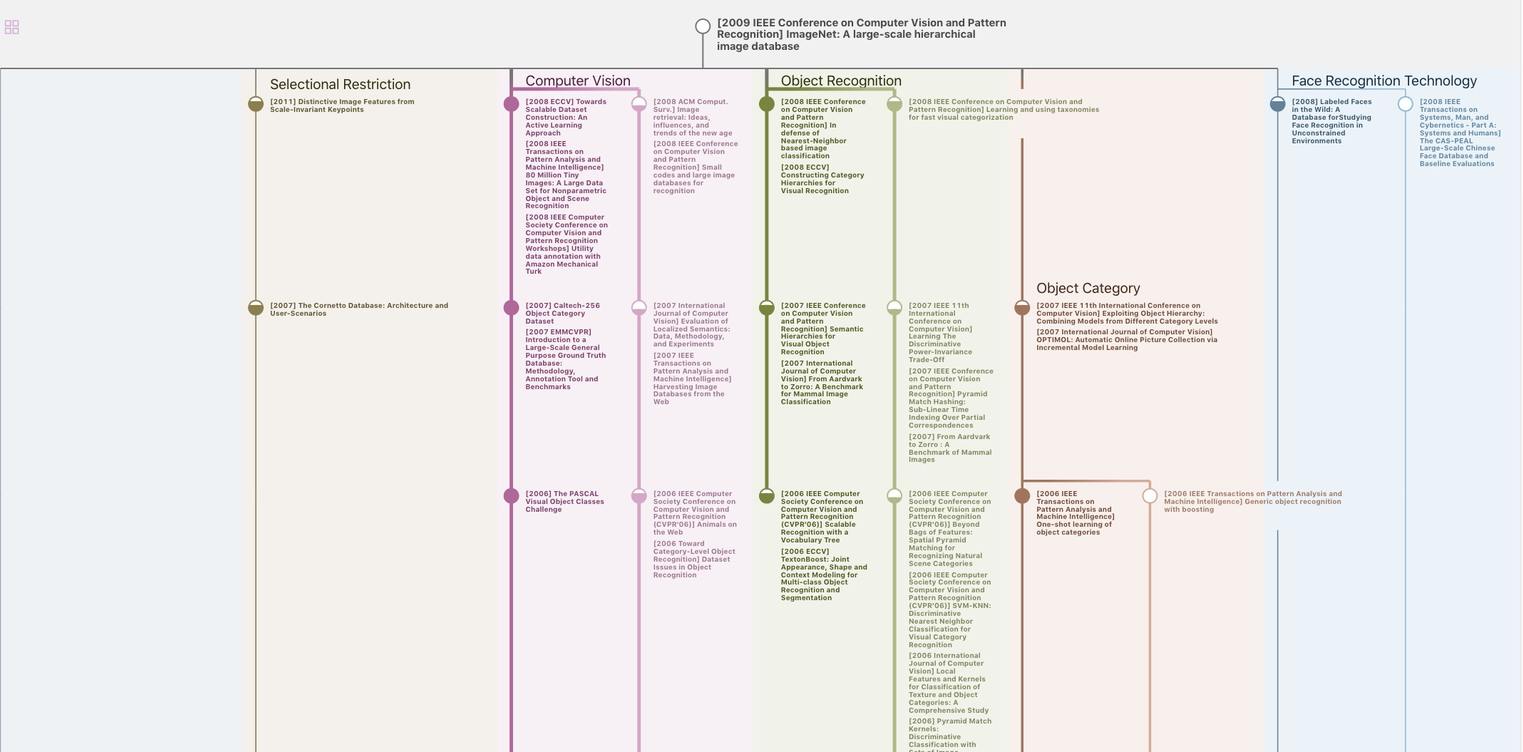
生成溯源树,研究论文发展脉络
Chat Paper
正在生成论文摘要