Learning broad learning system with controllable sparsity through L-0 regularization
Appl. Soft Comput.(2023)
摘要
As a novel neural network with efficient learning capacity, broad learning system (BLS) has achieved remarkable success in various regression and classification problems. Due to the broad expansion of nodes, however, BLS is known to have many redundant parameters and nodes, which will increase the memory and computation cost and is adverse to its deployment on equipment with limited resources. To optimize the number of neurons and parameters of BLS and then find the optimal sparse model under a given resource budget, in this paper, we introduce to train BLS through L0 regularization. The regularization constraint term of the BLS objective function is replaced by the L0 regularization method, and the normalized hard threshold iterative method is used to optimize the output weight. More concretely, the size of the model is fixed by controlling the number of output weights under given the resource size, and then parameters and nodes in the network are evaluated and selected from the node set in the training to obtain a BLS with controllable sparsity (CSBLS). Experiments on various data sets demonstrate the effectiveness of our proposed method. (C) 2023 Published by Elsevier B.V.
更多查看译文
关键词
broad learning system,controllable sparsity
AI 理解论文
溯源树
样例
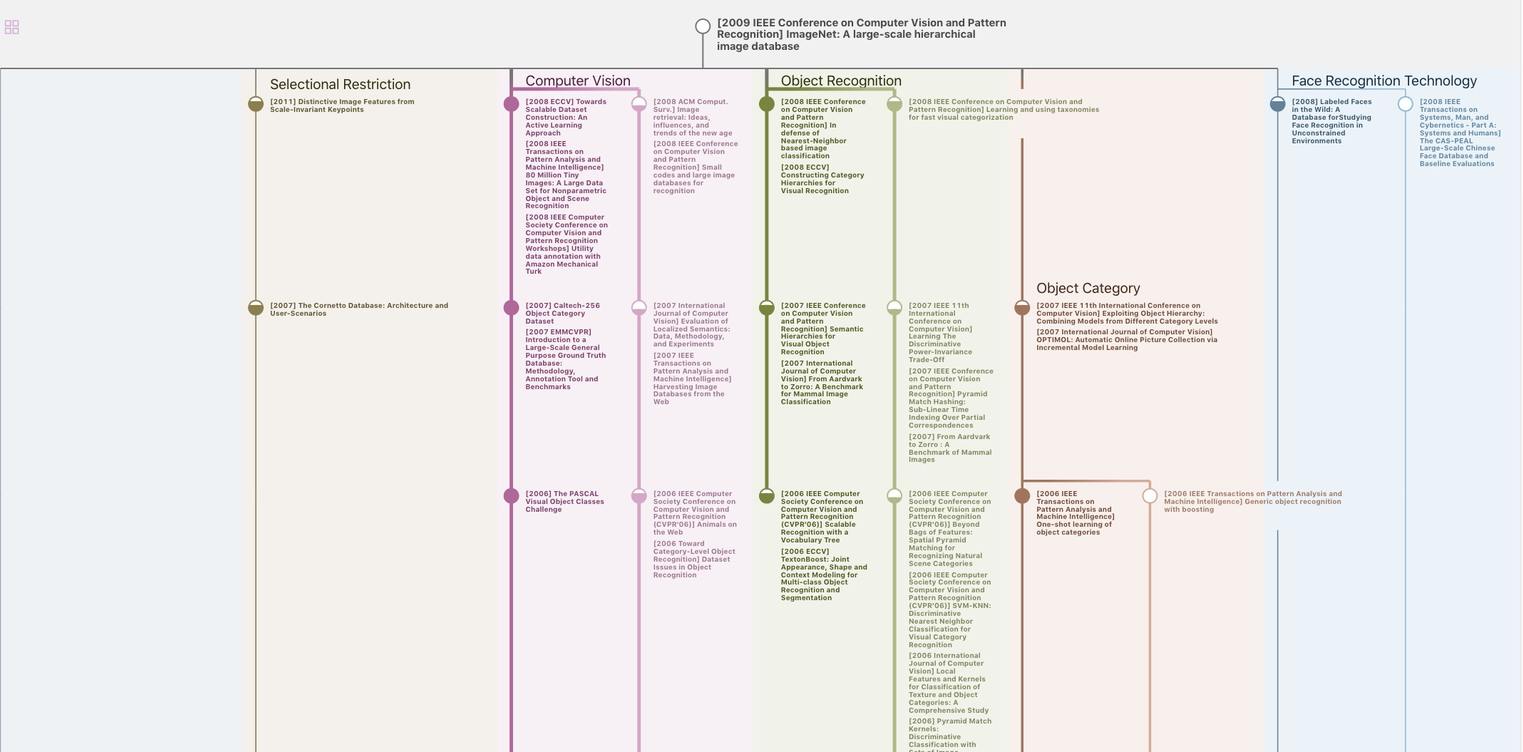
生成溯源树,研究论文发展脉络
Chat Paper
正在生成论文摘要