Interactive channel attention for rotating component fault detection with strong noise and limited data
Appl. Soft Comput.(2023)
摘要
Channel attention mechanisms have been widely applied to enhance the diagnosis performance of convolutional neural networks (CNN). However, most of the existing channel attention modules take insufficient account of the relationship among different channels, which restricts further improvement of channel attention-based diagnosis methods. Besides, the diagnosis model performance may greatly deteriorate when facing strong noise. To address the above problems, this paper proposed an improved intelligent fault detection method for rotating component based on interactive channel attention (ICA), which contains two submodules to help the CNN model pay attention to channel correlation of both global and local channels. Meanwhile, two more replaceable submodules based on adaptive multi-scale kernel (AMK) and self-attention (SA) are provided to consider the correlation of neighbor channels and long-term channels. Furthermore, a multi-scale convolutional layer with an adaptive selective kernel (ASK) unit is applied to replace the basic convolutional layer of CNN to further enhance the model performance. The proposed method can effectively enhance the feature extraction of the basic model in the scenario with a low signal-noise ratio (SNR) noise. Experimental results with two rotating component datasets demonstrate that the proposed method is superior to the comparison methods, especially in the occasions with strong noise and limited data.& COPY; 2023 Elsevier B.V. All rights reserved.
更多查看译文
关键词
Interactive channel attention,Convolutional neural network,Rotating component,Fault detection
AI 理解论文
溯源树
样例
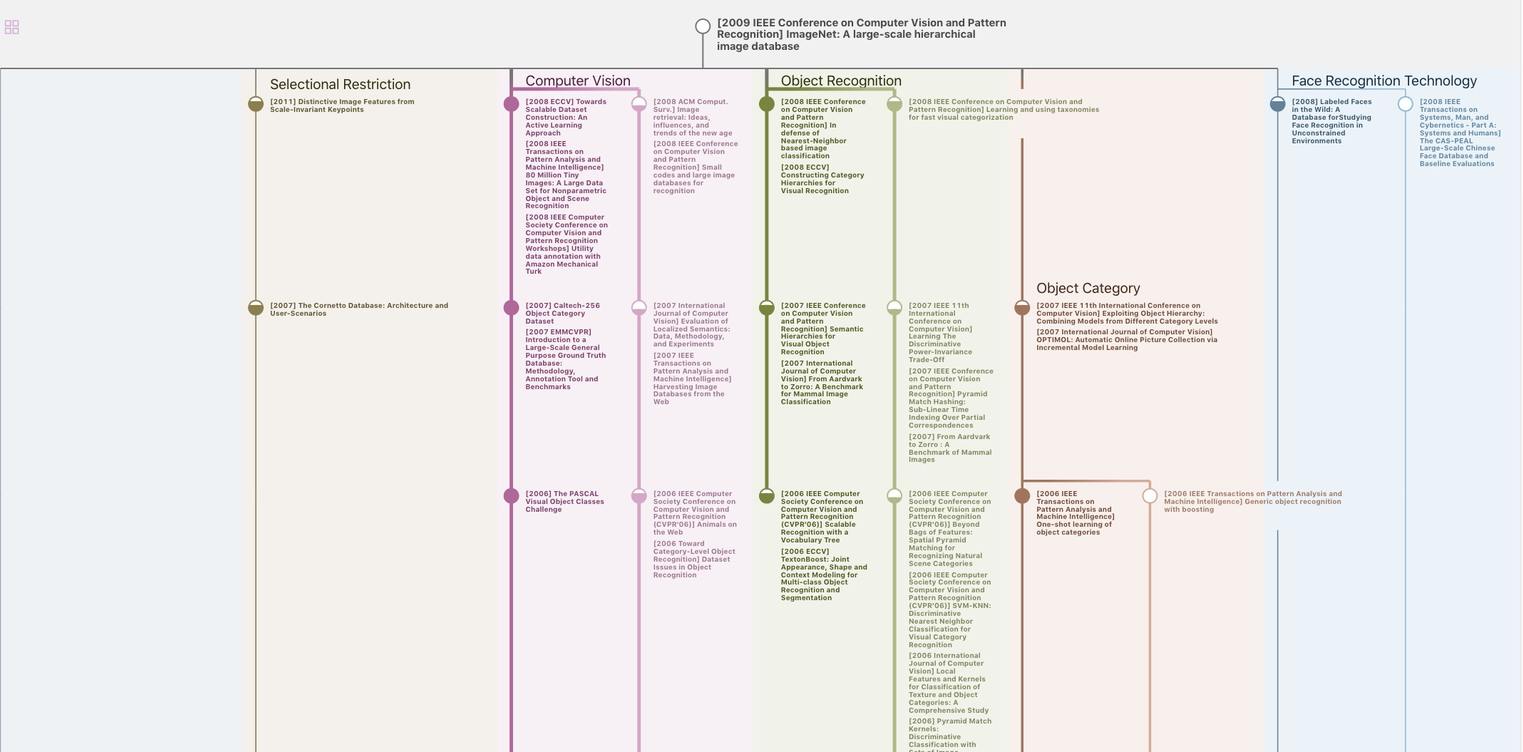
生成溯源树,研究论文发展脉络
Chat Paper
正在生成论文摘要