Veg-W2TCN: A parallel hybrid forecasting framework for non-stationary time series using wavelet and temporal convolution network model.
Appl. Soft Comput.(2023)
摘要
Long-term vegetation time series (TS) forecasting based on climatic data is one of the most challenging topics, capable of assisting in advanced estimation and management for scientific decision-making in different applications. However, these vegetation TS from remote sensing (i.e. normalized difference vegetation index (NDVI) and climatic data (standardized precipitation index (SPI)) are generally non-stationary in the real world. For this reason, traditional TS forecasting algorithms are incapable of extracting sufficient sequence data features, resulting in poor forecasting accuracy. In this paper, a novel parallel hybrid forecasting framework (Veg-W2TCN) is proposed combining multi-resolution analysis wavelet transform (MRA-WT) and temporal convolution network (TCN) model for vegetation change forecasting. In the first step, the NDVI and SPI TSs are divided into multiple groups using land cover information. For effective feature extraction, each group of data is decomposed using MRA-WT into trend and seasonal. Then, the obtained trend/seasonal from NDVI and SPI are concatenated. Multivariate TSs are obtained for each land cover. Then, each component is trained individually using the TCN model. In this step, the grid search algorithm is exploited to select the optimal TCN hyperparameters. Finally, a sum is used to integrate the different results. The proposed method is tested in the Mediterranean area. Veg-W2TCN proved its effectiveness for non-stationary TS forecasting. In fact, around 83% of pixels have a RMSE lower than 0,1 using Veg-W2TCN, compared to 74%, 72%, 60% and 59% of pixels are presented in TCN, long short-term memory (LSTM), multiple linear regression (MLR) and gated recurrent unit (GRU).
更多查看译文
关键词
parallel hybrid forecasting framework,wavelet,non-stationary
AI 理解论文
溯源树
样例
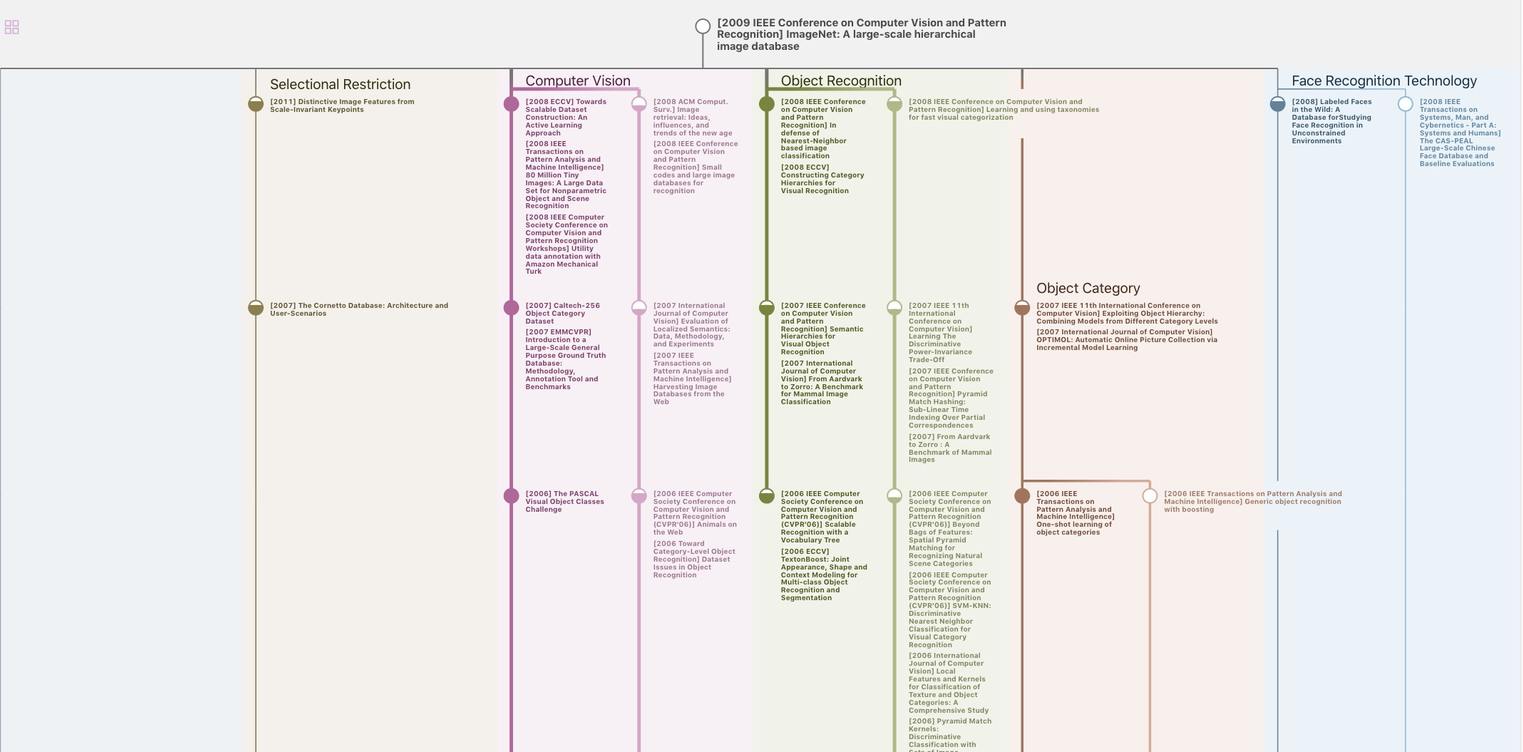
生成溯源树,研究论文发展脉络
Chat Paper
正在生成论文摘要