A verifiable and privacy-preserving framework for federated recommendation system
J. Ambient Intell. Humaniz. Comput.(2023)
摘要
The data such as features involved in recommendation systems often contain private information that can cause serious security problems if leaked to other participants in the system. At present, Federated Learning (FL) combined with encryption technology is a popular privacy preserving technology. However, the distributed computing of FL threatens the credibility of calculation results. Incorrect calculation results in the recommendation system can reduce the accuracy of the recommendation. In this paper, we design a verifiable and privacy-preserving framework for the federated recommendation system (VePriRec) to ensure the privacy of data and verifiability of calculation results. For three components involved in the system, we design three privacy-preserving protocols, including a secure similarity network construction protocol, a secure gradient descent protocol and a secure aggregation protocol. We conduct experiments on real-world datasets, the results demonstrate the effectiveness and efficiency of VePriRec.
更多查看译文
关键词
Recommendation system,Privacy preserving,Homomorphic encryption,Secret sharing
AI 理解论文
溯源树
样例
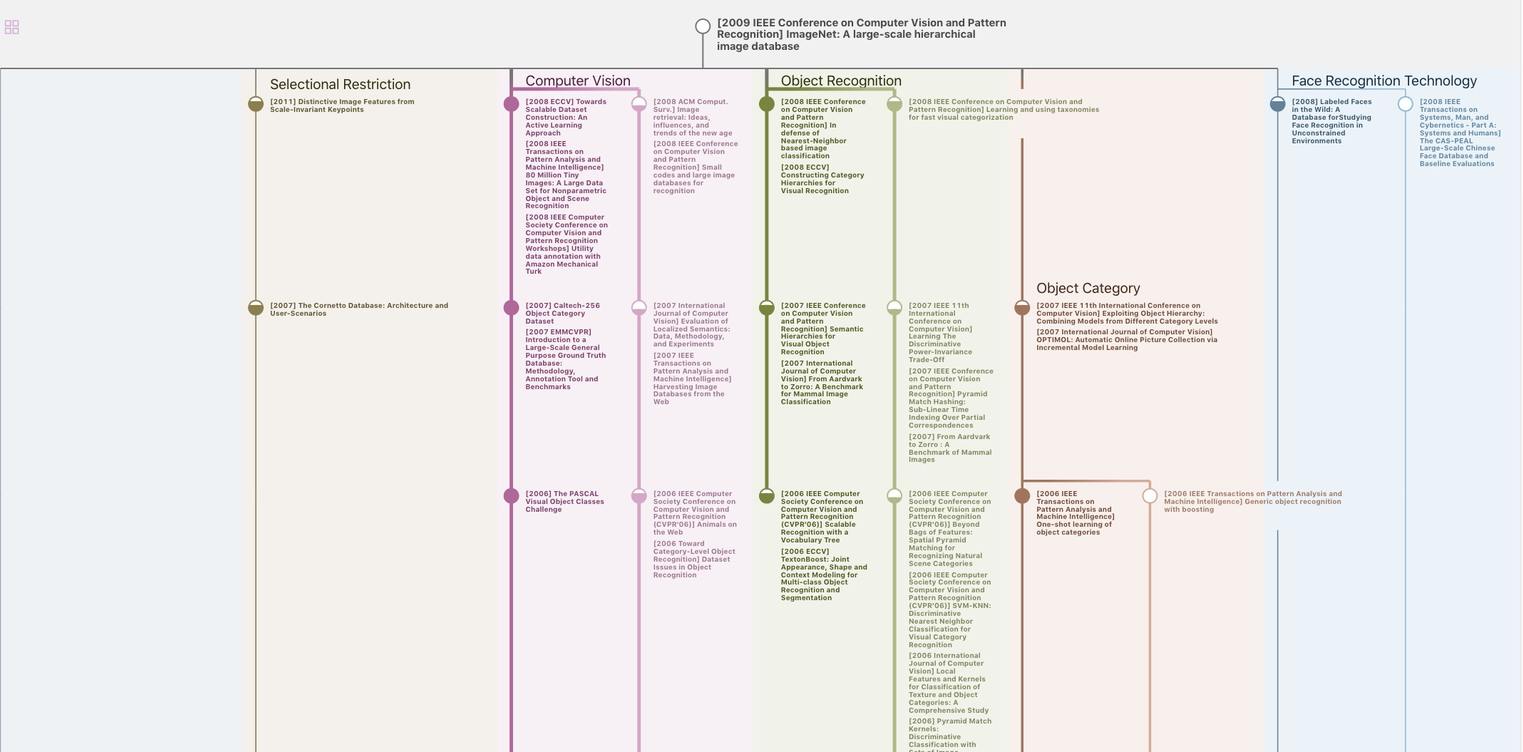
生成溯源树,研究论文发展脉络
Chat Paper
正在生成论文摘要