Single-machine scheduling with autonomous and induced learning to minimize total weighted number of tardy jobs.
Eur. J. Oper. Res.(2023)
摘要
As commonly observed in practice, firms can accelerate job processing by adding additional resources to the manufacturing system while striving to sustain on-time delivery. In this paper we consider due date assignment and scheduling with a learning effect that can be invoked by proactive investment at the beginning, known as induced learning in the literature. The objective is to find the optimal decisions on due date assignment, job sequencing, and level of induced learning to minimize a cost function consisting of the total weighted number of tardy jobs, due date penalty, and investment cost. We find a novel property of the optimal solution that although the due date penalty may be larger, the number of on-time jobs will not decrease with the increasing induced learning effect, which is a breakthrough to solve the problem. Exploiting the property, we present a polynomial-time algorithm that generates an approximation solution with a gap less than LE compared with the optimal result, where c can tend to be infinitesimal, and L is a constant.
更多查看译文
关键词
Scheduling,Induced learning effect,Tardy jobs,Dynamic programming
AI 理解论文
溯源树
样例
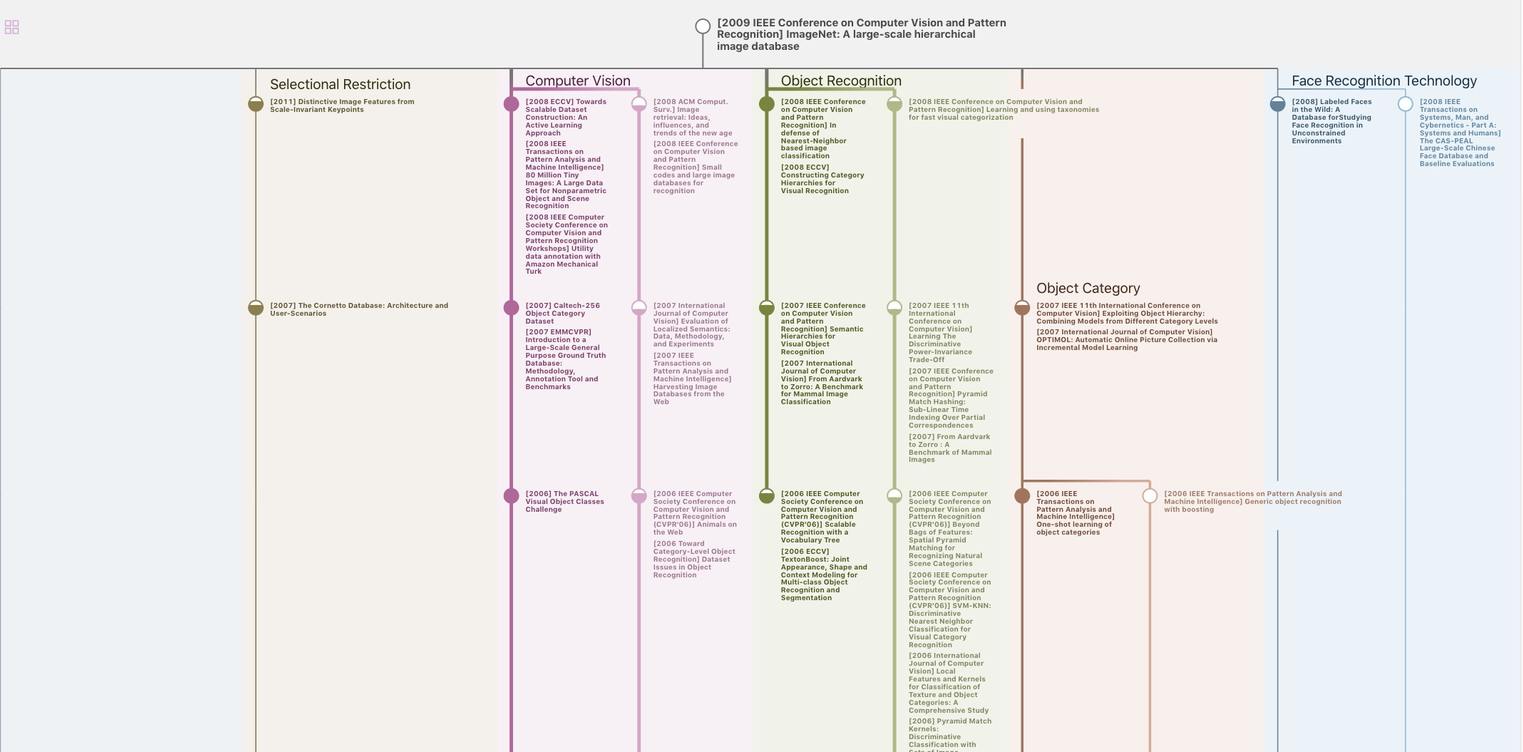
生成溯源树,研究论文发展脉络
Chat Paper
正在生成论文摘要