Safe semi-supervised learning for pattern classification.
Eng. Appl. Artif. Intell.(2023)
摘要
Semi-supervised learning (SSL) based on manifold regularization in many fields has attracted widespread attention , research. However, SSL still has two main challenges: On the one hand, studies have shown that unlabeled data may cause performance degradation in semi-supervised classifiers, which means that unlabeled data introduces uncertainty and potential hazards. On the other hand, for samples distributed on different class boundaries, manifold regularization is not necessarily satisfactory, which will result in samples near the boundary that are likely to be misclassified. In response to the above problems, we propose a new SSL framework called safe semi-supervised learning (Sa-SSLJR for short). In Sa-SSLJR, a risk degree regularization term is constructed to estimate the uncertainty and potential risk of unlabeled data in the semi-supervised learning process. Secondly, based on manifold regularization and discriminant regularization, a joint regularization term is developed to solve the second challenge of SSL. Extensive experiments on multiple datasets show that our approach is competitive with state-of-the-art methods in terms of classification performance and feasibility.
更多查看译文
关键词
Semi-supervised learning, Safe semi-supervised learning, Risk degree, Pattern classification, Manifold regularization
AI 理解论文
溯源树
样例
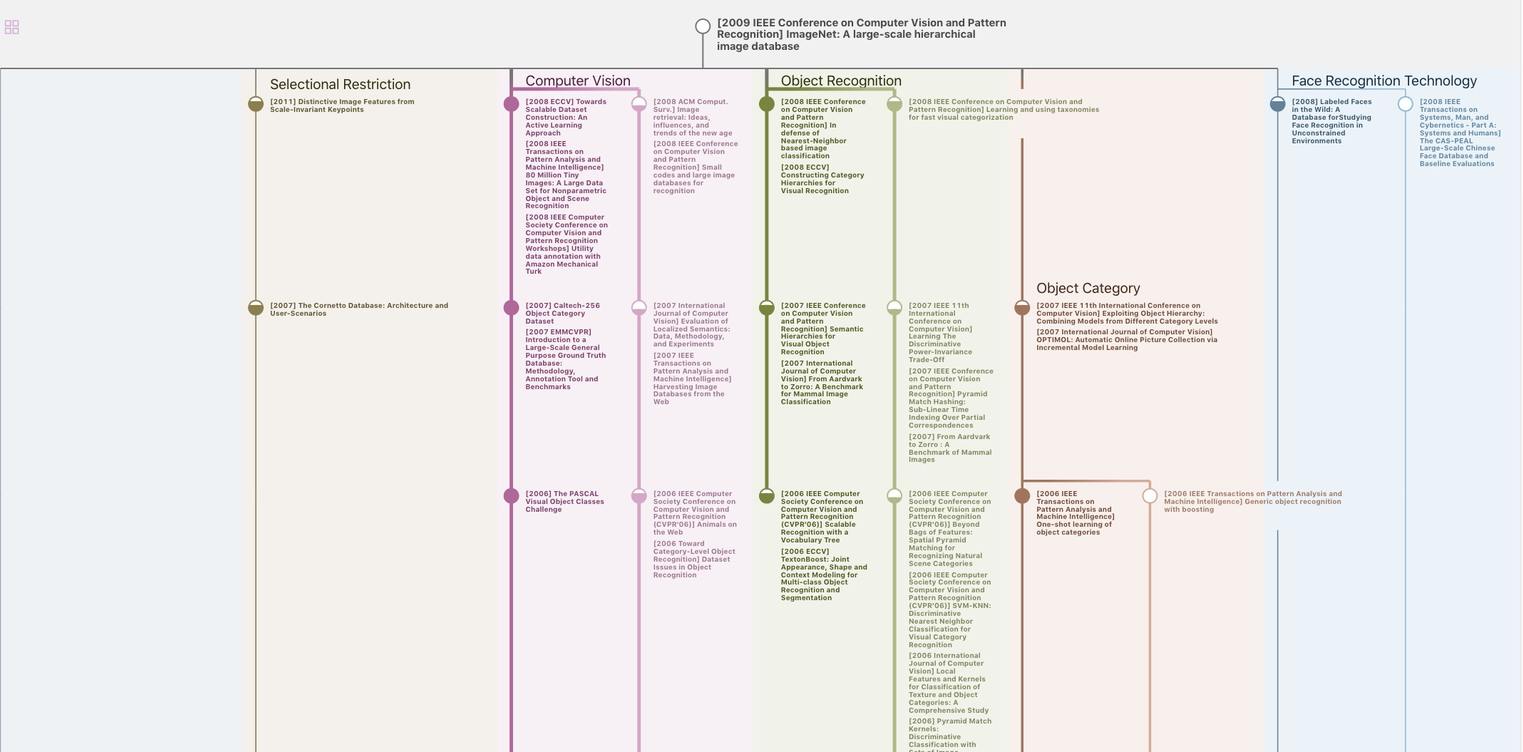
生成溯源树,研究论文发展脉络
Chat Paper
正在生成论文摘要