Forecasting the eddy current loss of a large turbo generator using hybrid ensemble Gaussian process regression.
Eng. Appl. Artif. Intell.(2023)
摘要
For the issue that the sample space of wedge winding eddy current losses of large generator does not obey Gaussian distribution, a hybrid ensemble Gaussian process regression (HEGPR) model is proposed in this paper. The HEGPR contains three layers. First, four tree regression models (XGBoost, CatBoost, LGBM and NGBoost) are built. Then, the output of the first layer is taken as the input of multiple Gaussian regression models, so that the input samples of the second layer obey Gaussian distribution, which can effectively improve the generalization ability of Gaussian process regression. The results show that the root mean squared error (RMSE) is 0.0282 and the goodness of fit (R2) is 0.9973. The model has good prediction performance for the eddy current loss of large turbo generator. Compared with kinds of Gaussian process models and traditional ensemble learning models, the prediction accuracy of this model is higher, and it is more suitable for forecasting eddy current loss of the large generator. HEGPR model can effectively solve the problem of insufficient regression accuracy of Gaussian process when sample space does not obey Gaussian distribution.
更多查看译文
关键词
Large generator, Eddy current loss, Gaussian process, Ensemble learning
AI 理解论文
溯源树
样例
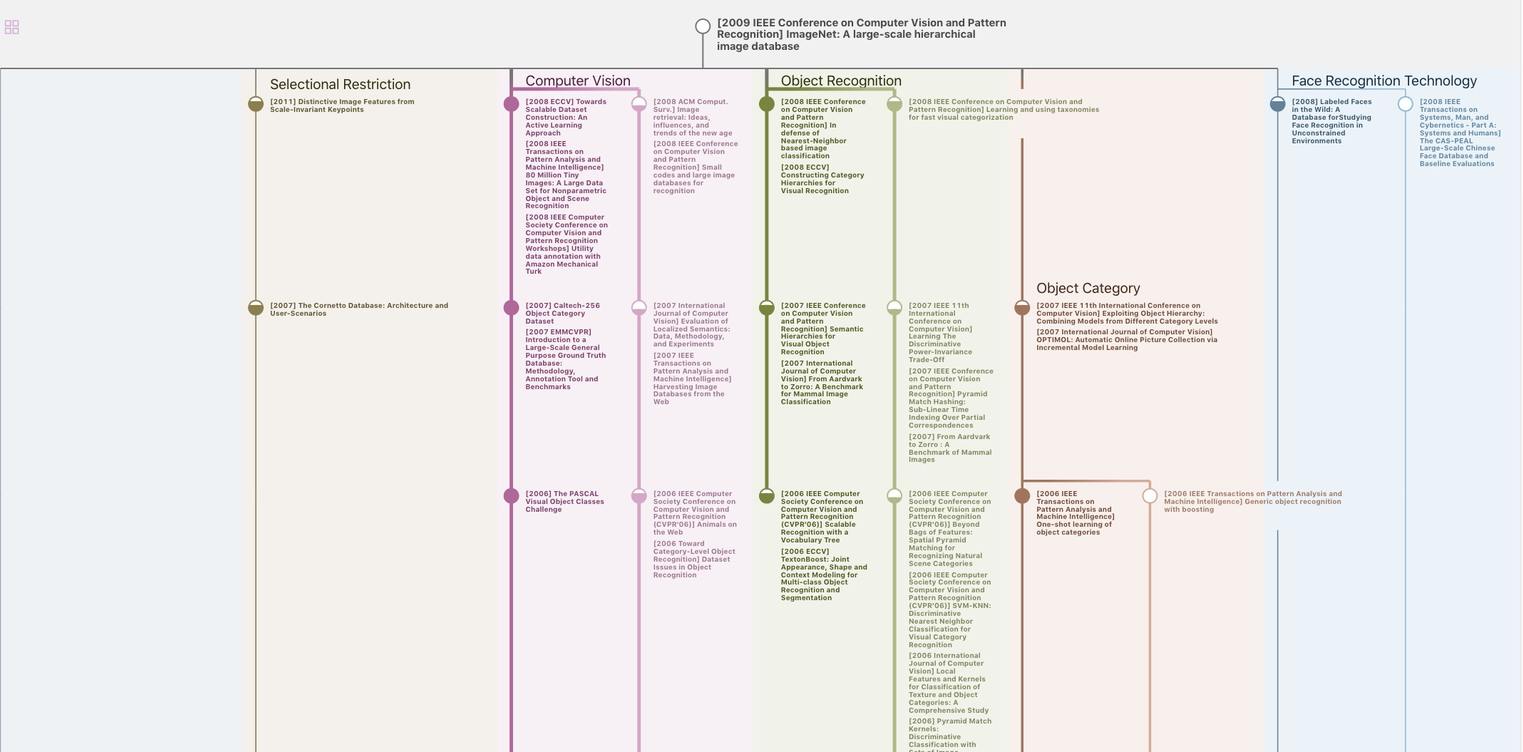
生成溯源树,研究论文发展脉络
Chat Paper
正在生成论文摘要