Contextual Multi-View Query Learning for Short Text Classification in User-Generated Data.
CoRR(2021)
摘要
Mining user-generated content--e.g., for the early detection of outbreaks or for extracting personal observations--often suffers from the lack of enough training data, short document length, and informal language model. We propose a novel multi-view active learning model, called Context-aware Co-testing with Bagging (COCOBA), to address these issues in the classification tasks tailored for a query word--e.g., detecting illness reports given the disease name. COCOBA employs the context of user postings to construct two views. Then it uses the distribution of the representations in each view to detect the regions that are assigned to the opposite classes. This effectively leads to detecting the contexts that the two base learners disagree on. Our model also employs a query-by-committee model to address the usually noisy language of user postings. The experiments testify that our model is applicable to multiple important representative Twitter tasks and also significantly outperforms the existing baselines.
更多查看译文
关键词
short contextual classification,multi-view,user-generated
AI 理解论文
溯源树
样例
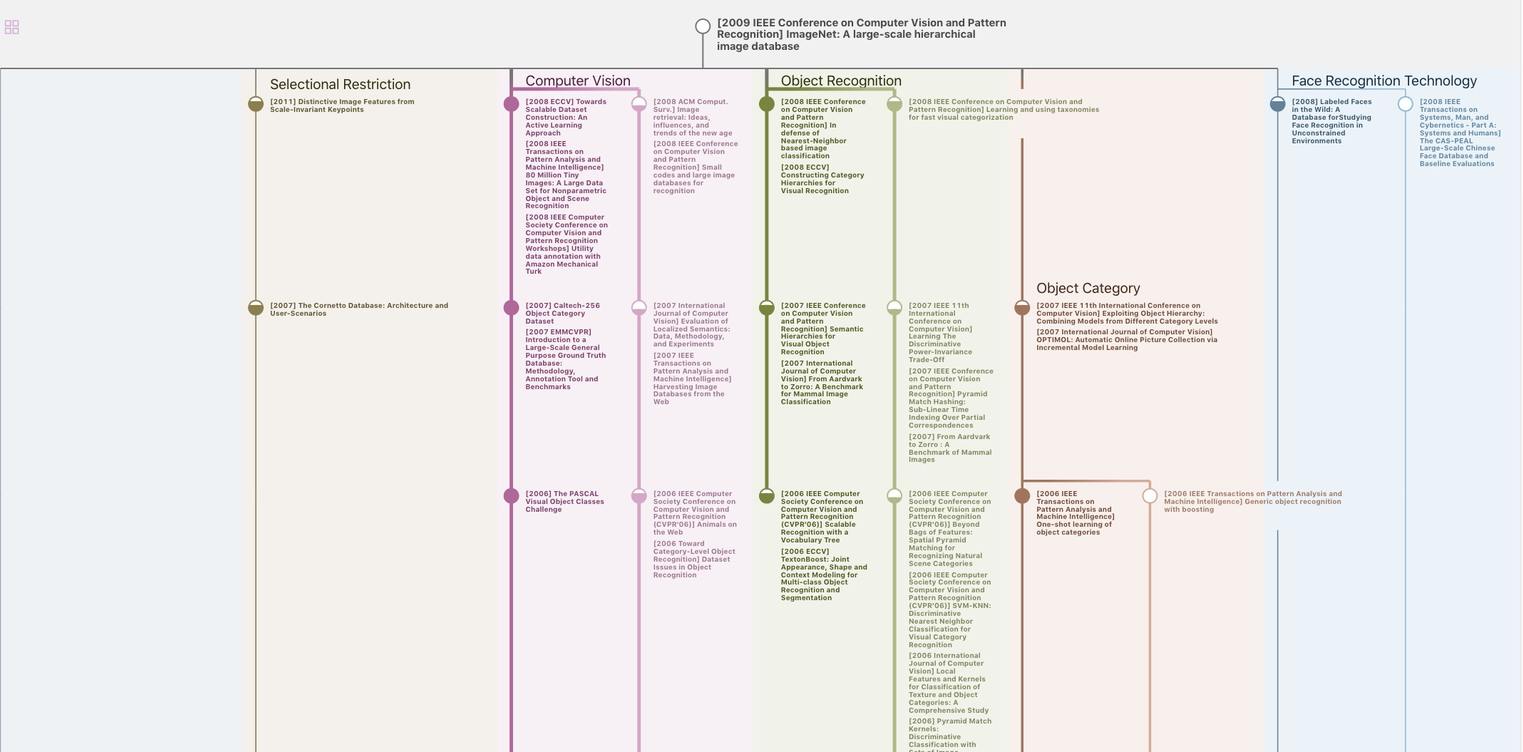
生成溯源树,研究论文发展脉络
Chat Paper
正在生成论文摘要