Active Visual Localization in Partially Calibrated Environments.
CoRR(2020)
摘要
Humans can robustly localize themselves without a map after they get lost following prominent visual cues or landmarks. In this work, we aim at endowing autonomous agents the same ability. Such ability is important in robotics applications yet very challenging when an agent is exposed to partially calibrated environments, where camera images with accurate 6 Degree-of-Freedom pose labels only cover part of the scene. To address the above challenge, we explore using Reinforcement Learning to search for a policy to generate intelligent motions so as to actively localize the agent given visual information in partially calibrated environments. Our core contribution is to formulate the active visual localization problem as a Partially Observable Markov Decision Process and propose an algorithmic framework based on Deep Reinforcement Learning to solve it. We further propose an indoor scene dataset ACR-6, which consists of both synthetic and real data and simulates challenging scenarios for active visual localization. We benchmark our algorithm against handcrafted baselines for localization and demonstrate that our approach significantly outperforms them on localization success rate.
更多查看译文
关键词
active visual localization,calibrated environments
AI 理解论文
溯源树
样例
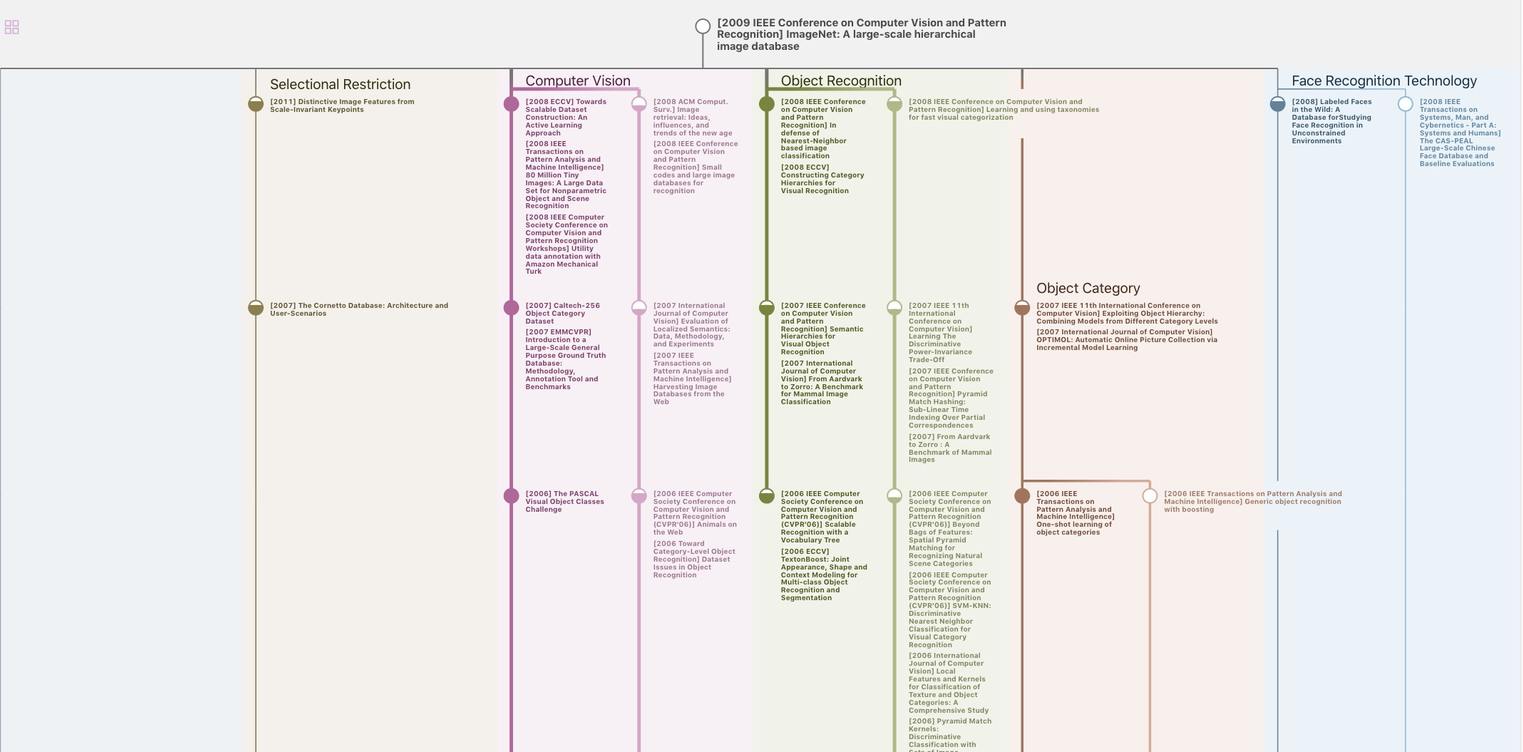
生成溯源树,研究论文发展脉络
Chat Paper
正在生成论文摘要