Learning Implicit Credit Assignment for Multi-Agent Actor-Critic.
CoRR(2020)
摘要
We present a new policy-based multi-agent reinforcement learning algorithm that implicitly addresses the credit assignment problem under fully cooperative settings. Our key motivation is that credit assignment may not require an explicit formulation as long as (1) the policy gradients of a trained, centralized critic carry sufficient information for the decentralized agents to maximize the critic estimate through optimal cooperation and (2) a sustained level of agent exploration is enforced throughout training. In this work, we achieve the former by formulating the centralized critic as a hypernetwork such that the latent state representation is now fused into the policy gradients through its multiplicative association with the agent policies, and we show that this is key to learning optimal joint actions that may otherwise require explicit credit assignment. To achieve the latter, we further propose a practical technique called adaptive entropy regularization where magnitudes of the policy gradients from the entropy term are dynamically rescaled to sustain consistent levels of exploration throughout training. Our final algorithm, which we call LICA, is evaluated on several benchmarks including the multi-agent particle environments and a set of challenging StarCraft II micromanagement tasks, and we show that LICA significantly outperforms previous methods.
更多查看译文
关键词
implicit credit assignment,learning,multi-agent,actor-critic
AI 理解论文
溯源树
样例
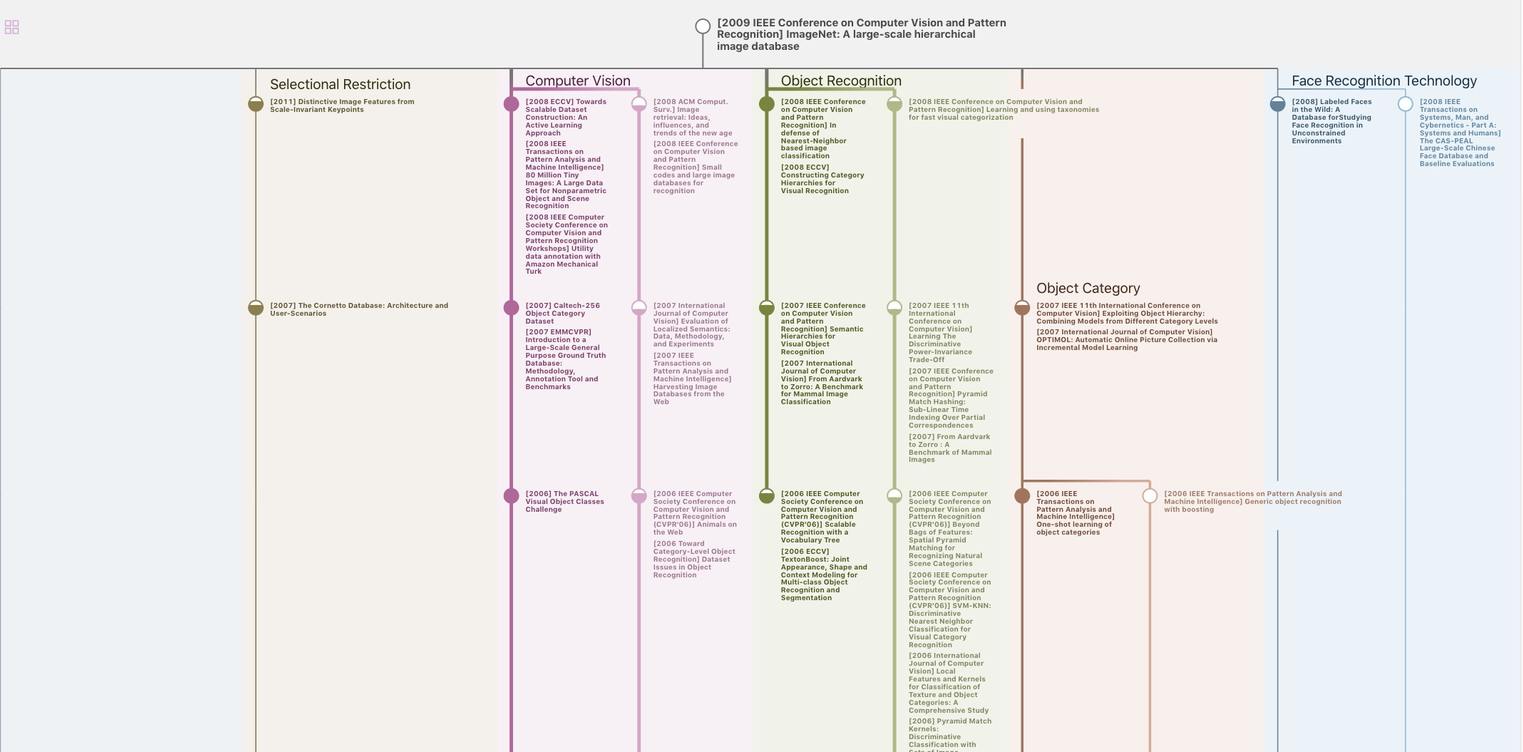
生成溯源树,研究论文发展脉络
Chat Paper
正在生成论文摘要