Learning Few-shot Open-set Classifiers using Exemplar Reconstruction.
CoRR(2021)
Abstract
We study the problem of how to identify samples from unseen categories (open-set classification) when there are only a few samples given from the seen categories (few-shot setting). The challenge of learning a good abstraction for a class with very few samples makes it extremely difficult to detect samples from the unseen categories; consequently, open-set recognition has received minimal attention in the few-shot setting. Most open-set few-shot classification methods regularize the softmax score to indicate uniform probability for open class samples but we argue that this approach is often inaccurate, especially at a fine-grained level. Instead, we propose a novel exemplar reconstruction-based meta-learning strategy for jointly detecting open class samples, as well as, categorizing samples from seen classes via metric-based classification. The exemplars, which act as representatives of a class, can either be provided in the training dataset or estimated in the feature domain. Our framework, named Reconstructing Exemplar based Few-shot Open-set ClaSsifier (ReFOCS), is tested on a wide variety of datasets and the experimental results clearly highlight our method as the new state of the art.
MoreTranslated text
Key words
exemplar reconstruction,learning,few-shot,open-set
AI Read Science
Must-Reading Tree
Example
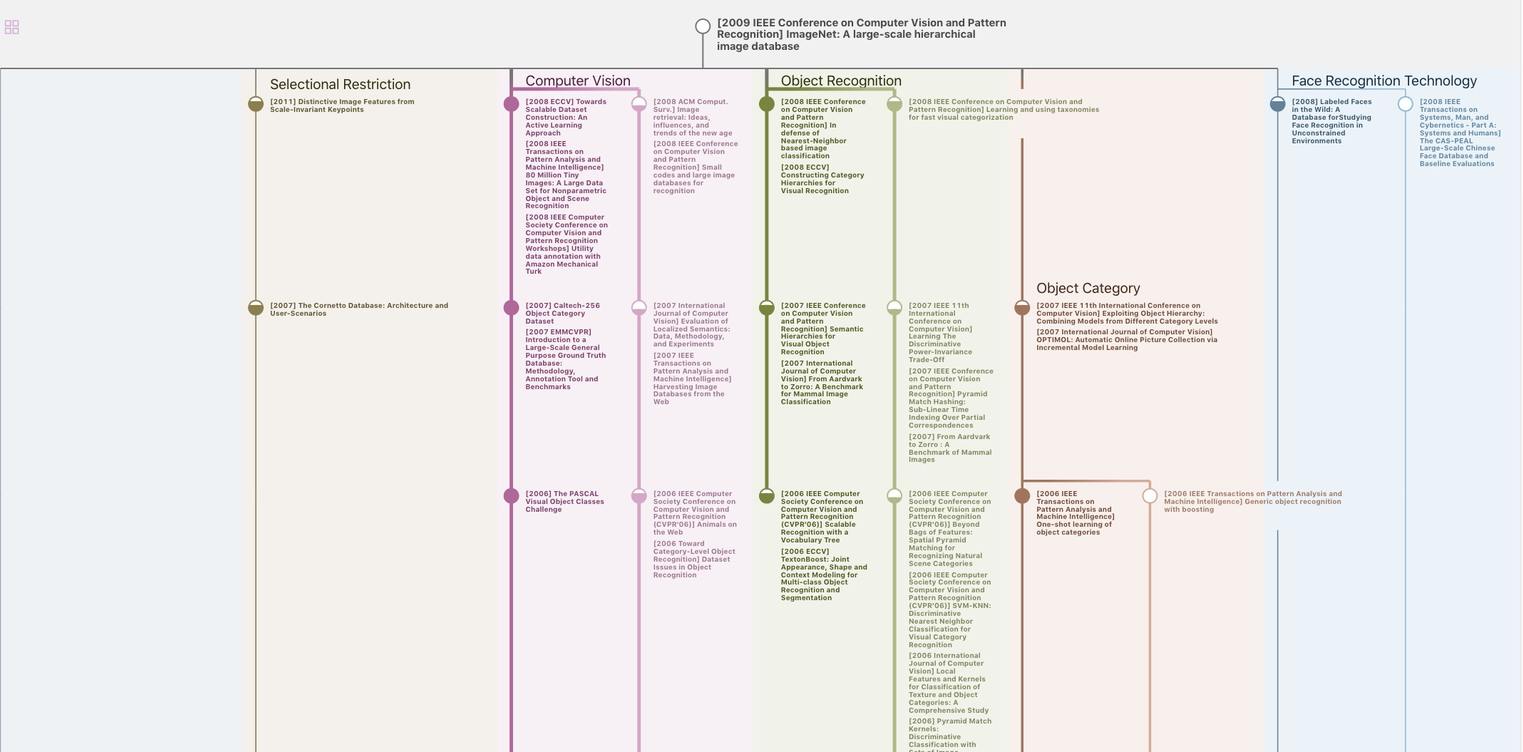
Generate MRT to find the research sequence of this paper
Chat Paper
Summary is being generated by the instructions you defined