Delayed Feedback in Episodic Reinforcement Learning.
CoRR(2021)
摘要
There are many provably efficient algorithms for episodic reinforcement learning. However, these algorithms are built under the assumption that the sequences of states, actions and rewards associated with each episode arrive immediately, allowing policy updates after every interaction with the environment. This assumption is often unrealistic in practice, particularly in areas such as healthcare and online recommendation. In this paper, we study the impact of delayed feedback on several provably efficient algorithms for regret minimisation in episodic reinforcement learning. Firstly, we consider updating the policy as soon as new feedback becomes available. Using this updating scheme, we show that the regret increases by an additive term involving the number of states, actions, episode length and the expected delay. This additive term changes depending on the optimistic algorithm of choice. We also show that updating the policy less frequently can lead to an improved dependency of the regret on the delays.
更多查看译文
关键词
episodic reinforcement learning,reinforcement learning,feedback
AI 理解论文
溯源树
样例
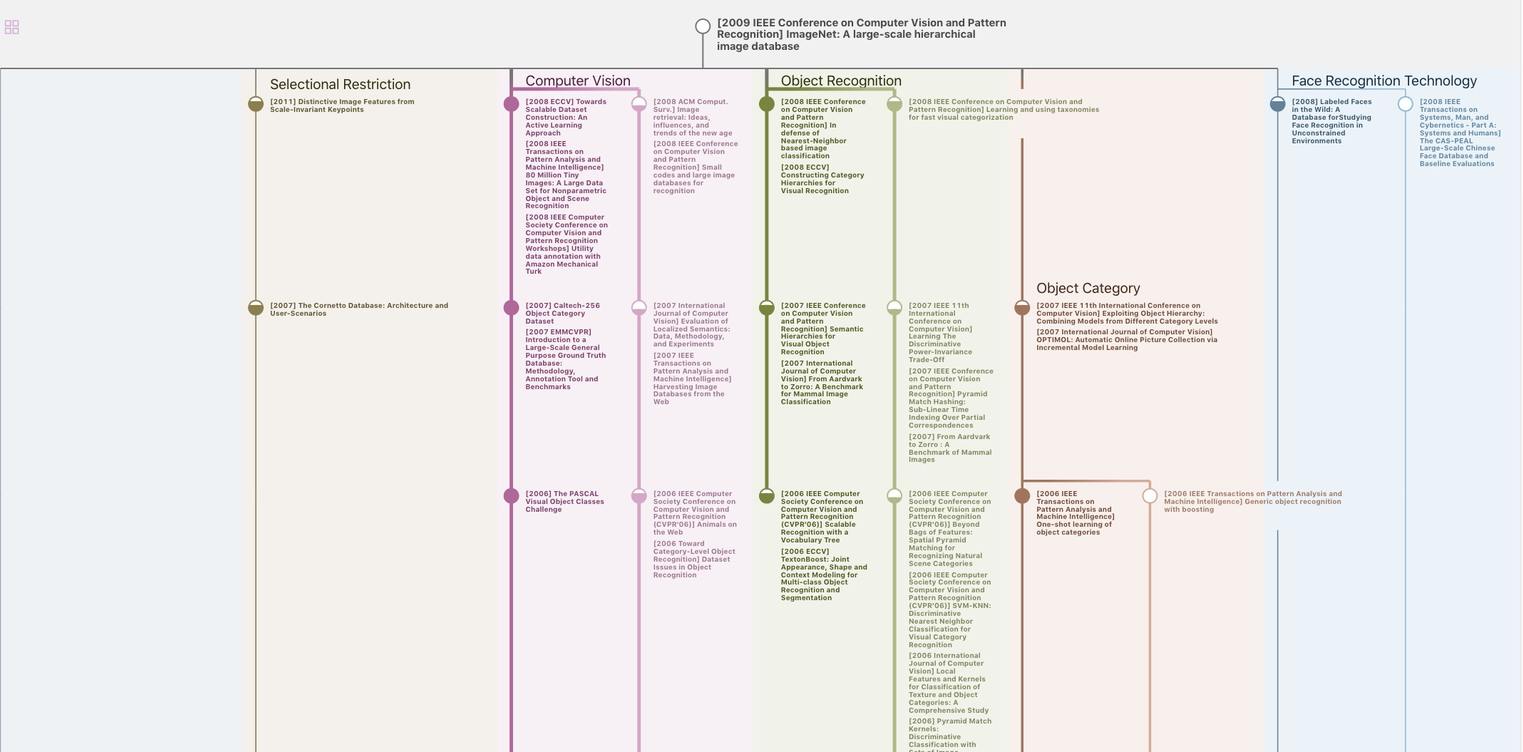
生成溯源树,研究论文发展脉络
Chat Paper
正在生成论文摘要