Reinforcement learning based joint trajectory design and resource allocation for RIS-aided UAV multicast networks.
Comput. Networks(2023)
摘要
This paper investigates an unmanned aerial vehicle (UAV)-enabled multicast network, where the UAV serves as a mobile transmitter to send typical contents to its corresponding ground receivers. A reconfigurable intelligent surface (RIS) is deployed to enhance the service quality with a limited power supply in the UAV-enabled multicast network. It can reconfigure the signal propagation environment and improve the received power of ground receivers by adjusting the reflection coefficients. The sum rate maximization problem is formulated by jointly designing the UAV movement, RIS reflection matrix, and beamforming design from the UAV to users. This paper proposes a Beamforming control and Trajectory design algorithm based on a Multi-Pass Deep Q -Network (BT-MP-DQN). In the proposed algorithm, the UAV acts as an agent for periodically observing the state of the UAV multicast network and takes actions to adapt to the dynamic environment. Specifically, the movement of the UAV is discrete action, and the beamforming design is continuous action. The simulation results show that this proposed algorithm can effectively improve the achievable rate and satisfy the minimum rate of multicast group users. The deployment of the RIS is beneficial to network performance enhancement. In addition, the multicast network with UAV also outperforms the conventional multicast channel with a fixed-location transmitter.
更多查看译文
关键词
Reconfigurable intelligent surface,UAV network,Multicast communication,Resource allocation,MP-DQN
AI 理解论文
溯源树
样例
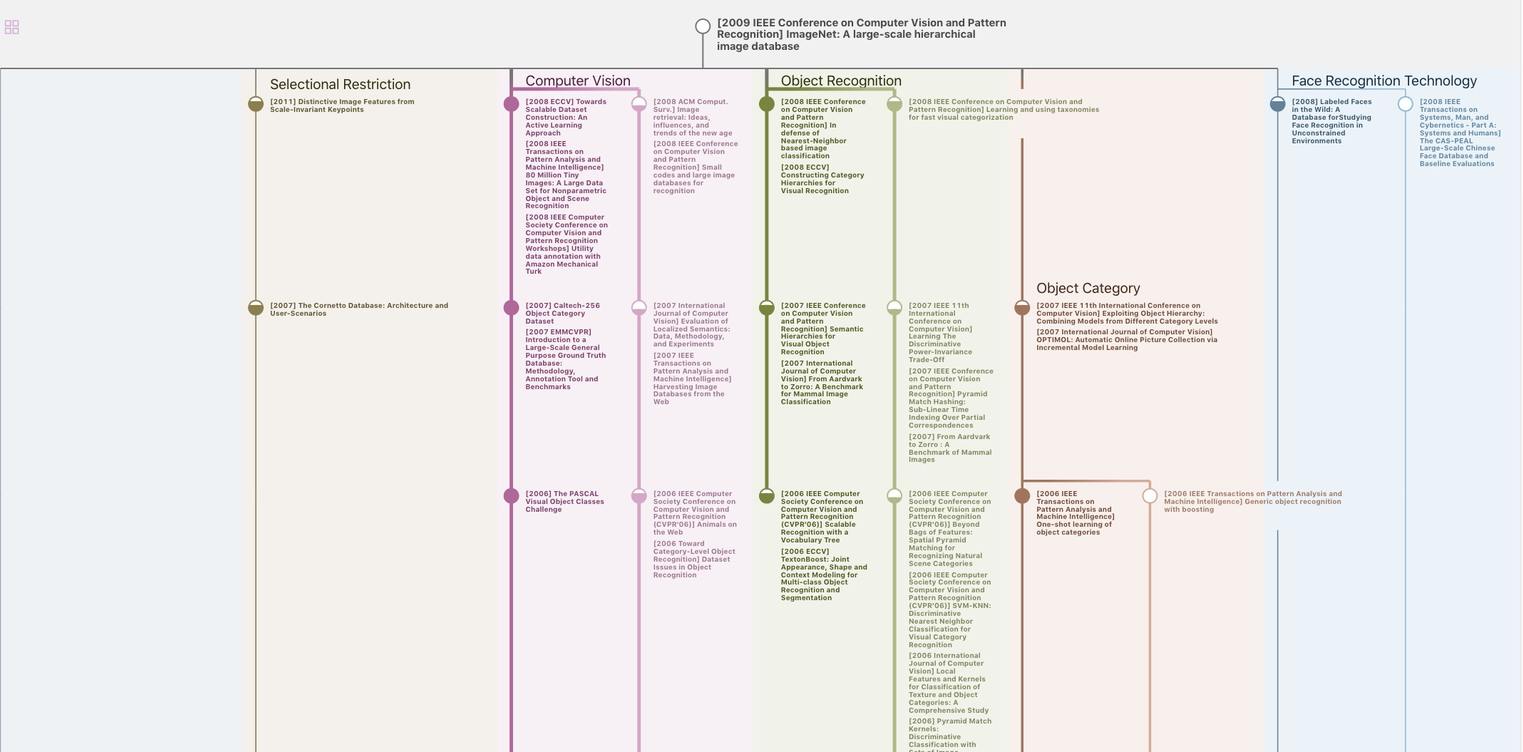
生成溯源树,研究论文发展脉络
Chat Paper
正在生成论文摘要