Analyzing the Performance of Transformers for the Prediction of the Blood Glucose Level Considering Imputation and Smoothing
Big Data Cogn. Comput.(2023)
摘要
In this paper we investigate the effect of two preprocessing techniques, data imputation and smoothing, in the prediction of blood glucose level in type 1 diabetes patients, using a novel deep learning model called Transformer. We train three models: XGBoost, a one-dimensional convolutional neural network (1D-CNN), and the Transformer model to predict future blood glucose levels for a 30-min horizon using a 60-min time series history in the OhioT1DM dataset. We also compare four methods of handling missing time series data during the model training: hourly mean, linear interpolation, cubic interpolation, and spline interpolation; and two smoothing techniques: Kalman smoothing and smoothing splines. Our experiments show that the Transformer performs better than XGBoost and 1D-CNN when only continuous glucose monitoring (CGM) is used as a predictor, and that it is very competitive against XGBoost when CGM and carbohydrate intake from the meal are used to predict blood glucose level. Overall, our results are more accurate than those appearing in the literature.
更多查看译文
关键词
diabetes,Transformer,1D-CNN,XGBoosting,glucose prediction,imputation,Kalman smoothing,smoothing splines
AI 理解论文
溯源树
样例
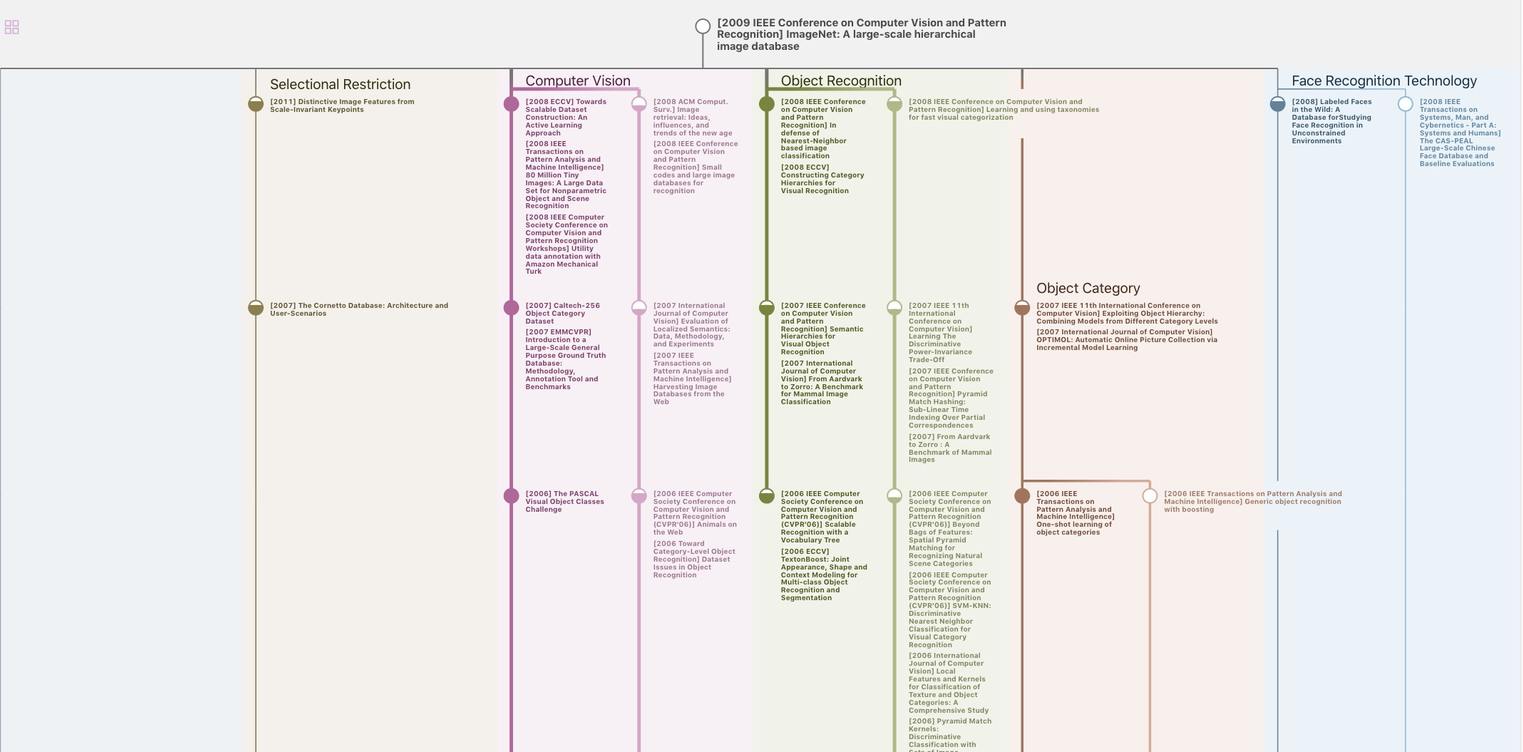
生成溯源树,研究论文发展脉络
Chat Paper
正在生成论文摘要