A hybrid deep learning approach for classification of music genres using wavelet and spectrogram analysis
Neural Comput. Appl.(2023)
摘要
Manual classification of millions of songs of the same or different genres is a challenging task for human beings. Therefore, there should be a machine intelligent model that can classify the genres of the songs very accurately. In this paper, a deep learning-based hybrid model is proposed for the analysis and classification of different music genre files. The proposed hybrid model mainly uses a combination of multimodal and transfer learning-based models for classification. This model is analyzed using GTZAN and Ballroom datasets. The GTZAN dataset contains 1000 music files classified with 10 different kinds of music genres such as Metal, Classical, Rock, Reggae, Pop, Disco, Blues, Country, Hip-Hop and Jazz, and the duration of each music file is 30 s. The Ballroom dataset contains 698 music files classified into 8 different kinds of music genres such as Tango, ChaChaCha, Rumba, Viennese waltz, Jlive, Waltz, Quickstep and Samba, and the duration of each music file is 30 s. The performance of the model is evaluated using the Python tool. The macro-average and weighted average are taken for computing the percentage of accuracy of each model. From the results, it is found that the proposed hybrid model is able to perform better as compared to other deep learning models such as the convolution neural network model, transfer learning-based model, multimodal model, machine learning models and other existing models in terms of training accuracy, validation accuracy, training loss, validation loss, precision, recall, F1-score and support.
更多查看译文
关键词
Music genre classification,Deep learning,Transfer learning,Multimodal
AI 理解论文
溯源树
样例
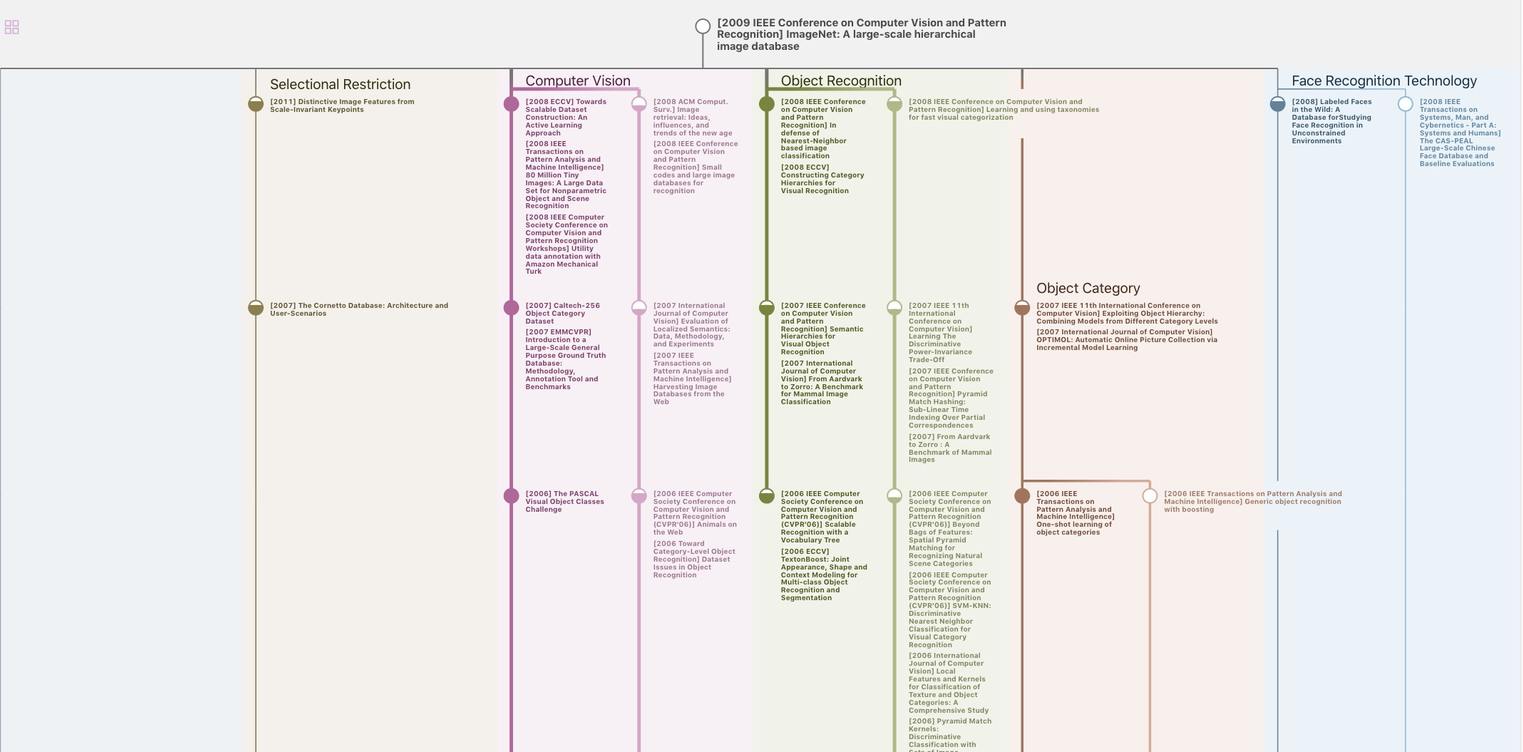
生成溯源树,研究论文发展脉络
Chat Paper
正在生成论文摘要