RepCPSI: Coordinate-Preserving Proximity Spectral Interaction Network With Reparameterization for Lightweight Spectral Super-Resolution.
IEEE Trans. Geosci. Remote. Sens.(2023)
摘要
Existing remarkable models for spectral super-resolution (SSR) achieve higher precision at the expense of computations with larger parameters. These algorithms require the heavy memory footprint and sufficient computing power, limiting their practical deployments and applications on portable devices. In this article, we propose an efficient reparameterizing coordinate-preserving proximity spectral interaction (RepCPSI) network for lightweight SSR. Specifically, the basic architecture is constituted of several polymorphic residual context restructuring (PRCR) modules to fully explore spatial and spectral contextual information with a multibranch topology during the training stage. Using a structural reparameterization scheme, the training-completed network is converted equivalently into a high-efficiency inference-time model, when it runs in the testing phase. To significantly improve the accuracy of SSR with an extra negligible computational overhead, a lightweight coordinate-preserving proximity spectral-aware attention (CPSA) block is developed. Such CPSA block can adaptively emphasize informative signatures and suppress useless ones among intermediate spatial-spectral features, which effectively enables the model to quickly locate features that are beneficial to network learning and representation. Furthermore, considering the continuity of spectral variation for capturing real-world hyperspectral images (HSIs), a spectral physical consistency loss (SPCL) is added to the end-to-end network to constrain the changing trend of the spectral curve to be consistent with the ground-truth objects. Finally, our RepCPSI can accomplish a favorable balance between the reconstructed quality and the model complexity. Extensive experimental results on six benchmarks demonstrate that our method obtains excellent performance with fewer parameters in terms of quantitative and qualitative measurements over the current advanced SSR approaches.
更多查看译文
关键词
Image reconstruction, Computational modeling, Correlation, Runtime, Benchmark testing, Adaptation models, Training, Coordinate-preserving proximity spectral-aware attention (CPSA), lightweight spectral super-resolution (SSR), polymorphic residual context restructuring (PRCR), reparameterizing coordinate-preserving proximity spectral interaction (RepCPSI), spectral physical consistency loss (SPCL)
AI 理解论文
溯源树
样例
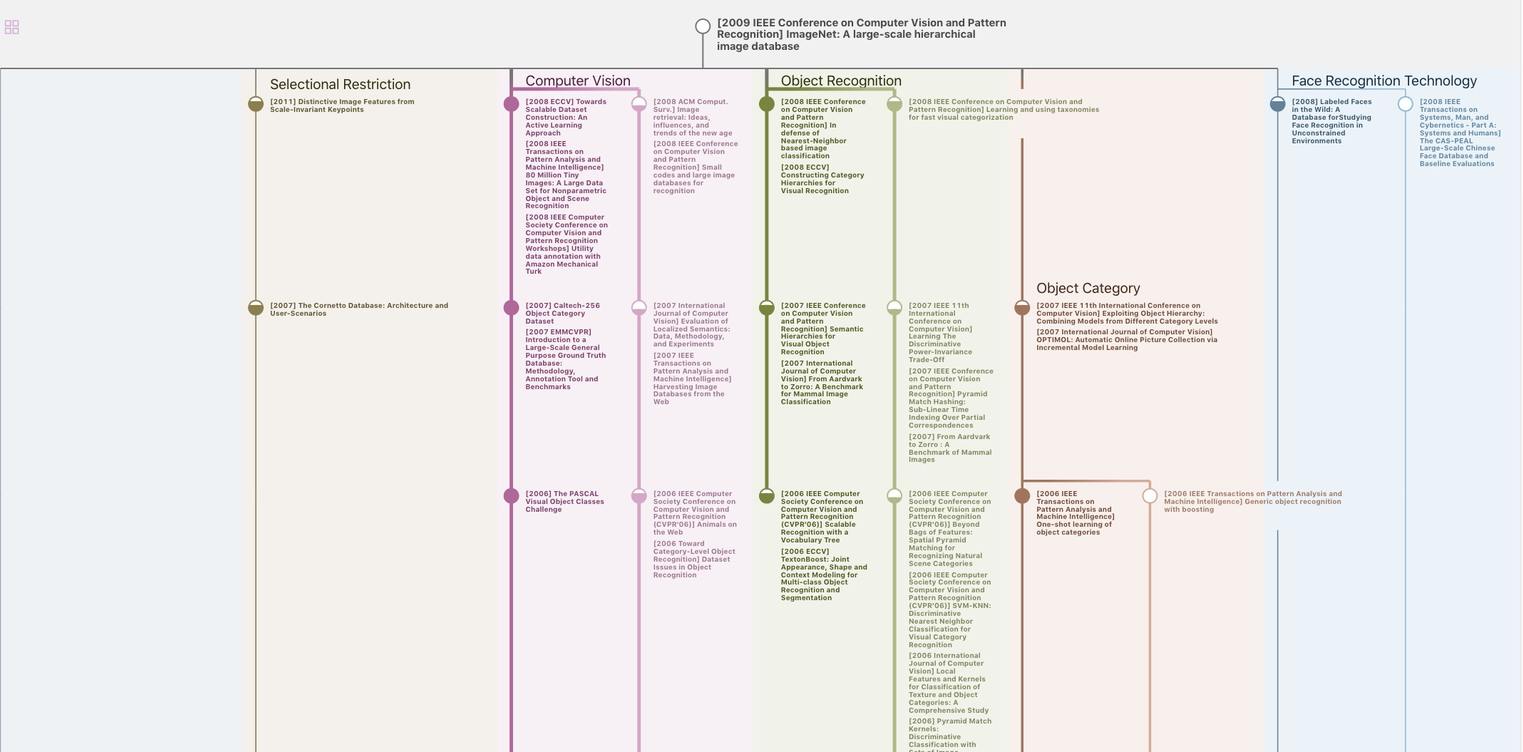
生成溯源树,研究论文发展脉络
Chat Paper
正在生成论文摘要